Signal Processing in Control Systems with MATLAB: From Filtering to Frequency Domain Analysis
发布时间: 2024-09-15 00:44:49 阅读量: 10 订阅数: 18 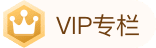
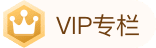
# 1. An Overview of MATLAB Signal Processing in Control Systems
In the design and analysis of modern control systems, the MATLAB software platform has become an indispensable tool for engineers and researchers, thanks to its powerful numerical computing capabilities and graphical functions. MATLAB's application in signal processing is particularly widespread; it not only aids in processing and analyzing various signals but also provides convenience in designing and simulating control systems. This chapter will outline the role of MATLAB in signal processing within control systems and discuss its importance in solving real-world problems.
The performance of control systems largely depends on the quality and processing of signals. Signal processing acts as a bridge combining control theory with practice, enabling us to manipulate, analyze, and optimize signals in digital form. MATLAB, with its extensive signal processing toolbox, offers the capability to process different types of signals (such as time-domain and frequency-domain signals) and implement various signal processing techniques.
Typical signal processing tasks include noise reduction, filtering, feature extraction, and signal transformation. MATLAB's signal processing toolbox provides a wealth of functions and tools for these tasks, such as Fourier transforms for spectral analysis, and filter design for signal smoothing. Additionally, MATLAB supports advanced applications for real-time signal processing and simulation, which is particularly useful for optimizing the performance of control systems. In this chapter, we will delve into these concepts and in subsequent chapters, we will detail the implementation methods of related technologies.
# 2. Basic Signal Theory and MATLAB Implementation
## 2.1 Classification and Characteristics of Signals
### 2.1.1 Continuous Signals and Discrete Signals
Signals are functions of time used to convey information. In the field of signal processing, signals are primarily divided into continuous signals and discrete signals. A continuous signal can be mathematically represented as a function of continuous variables, typically as a continuous function over time. Discrete signals, on the other hand, are sequences that take discrete values in time.
Continuous signals can be described by mathematical functions, for example:
```
f(t) = sin(ωt)
```
Here, `t` represents the time variable, and `ω` is the angular frequency. Continuous signal processing generally requires the use of mathematical tools such as integration and differentiation.
Discrete signals can be represented using vectors or arrays:
```
f[n] = sin(ωn)
```
Where `n` is an integer sequence, and discrete signal processing often involves operations like sequence addition, multiplication, and convolution.
MATLAB provides a rich set of toolboxes for the creation and manipulation of both continuous and discrete signals, allowing users to process signal problems using numerical and symbolic computation.
### 2.1.2 Common Signal Types: Step, Impulse, Sine Signals
In signal processing, there are several common signal types: step signals, impulse signals, and sine signals, which are widely used in both theory and practice.
A step signal (or unit step signal) is a sudden signal, mathematically defined as:
```
u(t) = 0, t < 0
u(t) = 1, t >= 0
```
In MATLAB, you can generate and plot a unit step signal using the following code:
```matlab
t = -1:0.01:1;
u = double(t >= 0);
stem(t,u);
xlabel('Time');
ylabel('Amplitude');
title('Unit Step Signal');
```
An impulse signal (or Dirac δ function) is mathematically described as having an infinite value at `t = 0`, with its integral being 1. In MATLAB, an impulse signal is often simulated using a narrow rectangular pulse with a height of `1/s` and a width of `s`, where `s` is sufficiently small, for example:
```matlab
s = 0.01;
t = -0.5:s:0.5;
impulse = double(abs(t) < s/2);
plot(t, impulse);
xlabel('Time');
ylabel('Amplitude');
title('Impulse Signal');
```
Sine signals are among the most common periodic signals, which can be represented mathematically as:
```
x(t) = A * sin(ωt + φ)
```
Where `A` is the amplitude, `ω` is the angular frequency, and `φ` is the phase. In MATLAB, the `sin` function can be used to create and manipulate sine signals:
```matlab
t = 0:0.01:2*pi;
A = 1;
w = 2*pi;
phi = pi/2;
sinusoidal = A * sin(w * t + phi);
plot(t, sinusoidal);
xlabel('Time');
ylabel('Amplitude');
title('Sine Wave Signal');
```
Processing signals in MATLAB can visually demonstrate the characteristics of different signals, facilitating the study and understanding of signal attributes.
## 2.2 Signal Representation and Operations in MATLAB
### 2.2.1 Creation and Representation of Signals
In MATLAB, creating and representing signals is very intuitive and straightforward. Built-in functions like `sin`, `cos`, `exp`, etc., can be used to create different types of signals. For discrete signals, we frequently use vectors to represent signal samples.
For example, to create a sine signal with a frequency of `f`, the following code can be used:
```matlab
Fs = 1000; % Sampling frequency
t = 0:1/Fs:1; % Time vector, 1 second long
f = 5; % Sine wave frequency is 5Hz
A = 2; % Amplitude
y = A*sin(2*pi*f*t); % Sine signal
plot(t,y);
xlabel('Time (s)');
ylabel('Amplitude');
title('Sine Wave Signal');
```
For complex signals, the sample values of different signals can be directly defined as vector forms and subjected to appropriate mathematical operations.
### 2.2.2 Time-Domain Operations on Signals
Operating on signals in the time domain includes adding, multiplying, scaling, and shifting signals. These operations are easily implemented in MATLAB because signals can be treated as arrays or vectors.
For example, the addition of two signals can be achieved through direct addition operations:
```matlab
% Continuing from the previous example
f2 = 10; % Frequency of another sine wave is 10Hz
y2 = 0.5*sin(2*pi*f2*t); % Second sine signal
y_combined = y + y2; % Adding two signals
figure;
plot(t, y_combined);
xlabel('Time (s)');
ylabel('Amplitude');
title('Sum of Two Sine Waves');
```
Signal scaling can be accomplished using multiplication operations, such as amplifying the signal amplitude by 2:
```matlab
y_scaled = 2 * y;
plot(t, y_scaled);
xlabel('Time (s)');
ylabel('Amplitude');
title('Scaled Sine Wave Signal');
```
Signal shifting can be implemented through addition and multiplication by time, for instance, shifting 0.1 seconds to the right:
```matlab
t_shifted = t + 0.1;
y_shifted = y;
plot(t_shifted, y_shifted);
xlabel('Time (s)');
ylabel('Amplitude');
title('Time Shifted Sine Wave');
```
Signal operations in MATLAB are very flexible and support more complex operations such as filtering, modulation, demodulation, etc., providing powerful tools for signal processing.
## 2.3 Signal Transformations in MATLAB
### 2.3.1 Fourier Transform
The Fourier transform is a core concept in signal processing, capable of transforming time-domain signals into the frequency domain for analysis. The Fourier transform can decompose a signal, representing it as a superposition of sine waves at different frequencies.
In MATLAB, the `fft` function can be used to compute the Fast Fourier Transform (FFT) of a signal. For example, for the previously created sine signal, we can obtain its frequency spectrum:
```matlab
N = length(t); % Signal length
Y = fft(y, N); % FFT transformation
f = Fs*(0:(N/2))/N; % Frequency vector
figure;
plot(f, abs(Y(1:N/2+1)));
xlabel('Frequency (Hz)');
ylabel('Magnitude');
title('Magnitude Spectrum of Sine Wave');
```
### 2.3.2 Laplace Transform
The Laplace transform is crucial in the analysis of continuous systems, especially in control systems and circuit analysis. MATLAB provides the `laplace` function to calculate the Laplace transform of a function or the `tf` function to create transfer function models.
For example, calculating the Laplace transform of the unit step function:
```matlab
syms t s
f = heaviside(t); % Unit step function
F = laplace(f, t, s); % Laplace transform
pretty(F)
```
The Laplace transform can also be used to solve ordinary differential equations. For instance, consider a first-order linear differential equation:
```matlab
% Initial conditions and differential equation
y0 = 0;
ode = diff(y,t) + 2*y == 1;
cond = y(0) == y0;
% Laplace transform solution
Ys = dsolve(ode, cond, 's')
```
MATLAB offers a suite of tools for processing time-domain and frequency-domain analysis, making complex mathematical computations straightforward. In practical applications, these signal transformation tools help engineers gain an in-depth understanding of the intrinsic characteristics of signals, thus designing more effective signal processing systems.
# 3. ```
# Chapter 3: MATLAB Filter Design and Application
In automation and communication systems, filter design is a critical part of signal processing. Filters primarily allow signals within a specific frequency range to pass through while suppressing others. MATLAB provides powerful toolboxes for designing and analyzing various types of filters. This chapter will delve into the basic theory of filters, how to design filters in MATLAB, and perform frequency domain analysis.
## 3.1 Basic Filter Theory
### 3.1.1 Filter Classification and Characteristics
Filters are typically categorized by their frequency response type and mainly include low-pass, high-pass, band-pass, and band-stop (notch) filters. Low-pass filters permit low-frequency signals to pass through while blocking high-frequency signals; high-pass filters allow high-frequency signals to pass; band-pass filters permit signals within a specific frequency band to pass through; band-stop fil
```
0
0
相关推荐
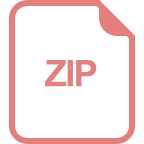
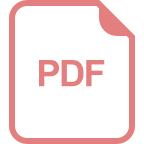
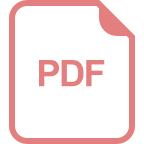





