Challenges and Solutions for Multi-Label Classification Problems: 5 Strategies to Help You Overcome Difficulties
发布时间: 2024-09-15 11:45:32 阅读量: 36 订阅数: 31 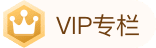
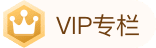
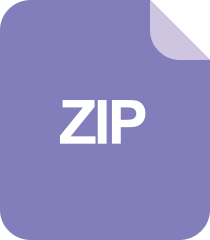
Javascript-Common-Challenges-Problems:JavaScriptPython中日常挑战技术的集合
# Challenges and Solutions for Multi-Label Classification Problems: 5 Strategies to Overcome the Difficulties
## 1.1 Definition and Applications of Multi-Label Classification
Multi-label classification is an important branch of machine learning, different from traditional single-label classification, it aims to predict multiple labels for instances. In the real world, this problem widely exists in various fields such as image recognition, natural language processing, and bioinformatics. For example, a photo may contain various tags such as "beach", "sunset", and "portrait" at the same time. The difficulty of this problem lies in the possible correlation between tags, the complexity of the label space and feature space, which requires the algorithm to not only accurately predict individual tags but also reasonably deal with the dependencies between tags.
## 1.2 Importance of Multi-Label Classification
Multi-label classification has attracted widespread attention because it can provide richer and more flexible information descriptions in many practical problems. For example, through multi-label classification, personalized recommendations can be provided for user recommendation systems, or more comprehensive tag descriptions can be provided for cases in medical diagnosis to assist doctors in making more accurate judgments. Therefore, mastering multi-label classification technology is of great value for improving the intelligence level of related applications.
# 2. Theoretical Foundation and Algorithm Framework
### Theoretical Foundation of Multi-Label Classification
Multi-label classification is an important problem in machine learning, in which each instance is associated with a set of labels, rather than being associated with only one label as in traditional single-label classification problems. Understanding the theoretical foundation of multi-label classification is crucial for correctly implementing algorithms and evaluating their performance.
#### Label Space and Feature Space
In multi-label classification, the label space and feature space are two core concepts.
- **Label Space**: refers to the set of all possible labels, and the size of the label space is determined by the number and nature of different categories. For example, in image annotation tasks, the label space may include various categories such as "cat", "dog", "bird".
- **Feature Space**: represents the set of attributes of instances, each instance corresponds to a feature vector in the feature space.
In multi-label problems, an instance may belong to multiple labels at the same time, so the label space is no longer binary (belonging or not belonging) as in single-label problems, but is multi-valued. In this case, researchers cannot simply use traditional binary classifiers, but need more complex models to handle the prediction of multiple labels at the same time.
#### Multi-Label Classification and Multi-Task Learning
Multi-label classification is closely related to multi-task learning (MTL). In multi-task learning, a model is designed to learn multiple related tasks at the same time, hoping to help other tasks while learning one task. Multi-label classification can be regarded as a multi-task learning problem, where the prediction task of each label is an individual task.
### Common Multi-Label Classification Algorithms
The choice of multi-label classification algorithms depends on factors such as the complexity of the specific problem, the size of the dataset, and the type of features. The following are some common algorithms and their brief introductions.
#### Binary Relevance Algorithm
Binary relevance algorithms, such as binary association rule learning, are often used in multi-label classification problems, breaking the problem down into several binary classification problems. The simplest method is to train a binary classifier for each label, and then use the outputs of these classifiers to determine the final multi-label prediction.
#### Tree-Based Algorithms
Tree-based algorithms, such as random forests and gradient boosting machines (GBM), are also commonly used in multi-label classification due to their natural multi-output capability and good interpretability. These algorithms can be trained in parallel and do not require extensive preprocessing of the feature space.
#### Neural Network Methods
In recent years, deep learning methods, especially convolutional neural networks (CNN) and recurrent neural networks (RNN), have achieved significant results in multi-label classification tasks. Neural network methods can learn complex nonlinear mapping relationships and are effective for processing large datasets.
### Algorithm Performance Evaluation Criteria
In multi-label classification problems, the evaluation criteria are also more complex. The definitions of accuracy, precision, and recall are slightly different from traditional single-label classification. Next, we will introduce several commonly used evaluation criteria.
#### Accuracy and Precision
- **Accuracy**: In multi-label classification problems, accuracy usually refers to the ratio of the size of the intersection to the size of the union of the predicted label set and the actual label set.
- **Precision**: Indicates what proportion of the predicted positive labels are actually positive.
#### F1 Score and H Index
- **F1 Score**: Is the harmonic mean of precision and recall, a high F1 score means both precision and recall are high.
- **H Index**: Is a measure of the balance between the model's precision and recall, suitable for evaluating the robustness of the model.
#### ROC and AUC Curves
- **ROC Curve**: The receiver operating characteristic curve shows the true positive rate and false positive rate of the model under different thresholds.
- **AUC Value**: The area under the ROC curve is used to measure the overall performance of the model.
In the next chapter, we will delve into data preprocessing and feature engineering to understand how to improve the accuracy and efficiency of multi-label classification through these methods.
# 3. Data Preprocessing and Feature Engineering
Data is the "food" for machine learning models, and preprocessing and feature engineering are important steps to improve model performance. This chapter will delve into how to efficiently perform data preprocessing and feature engineering in multi-label classification problems.
## 3.1 Data Cleaning and Preprocessing Techniques
### 3.1.1 Handling Missing Values
In real-world datasets, missing values are a common problem. Missing values may be caused by errors in data collection, recording, or transmission. Depending on the situation of missing values, we can adopt several strategies to handle them:
- Delete records containing missing values.
- Fill in missing values (e.g., using mean, median, mode, or prediction models).
#### Example Code
```python
import pandas as pd
from sklearn.impute import SimpleImputer
# Assuming df is a DataFrame containing missing values
imputer = SimpleImputer(strategy='mean') # Use the mean of each column to fill in
df_filled = pd.DataFrame(imputer.fit_transform(df), columns=df.columns)
```
#### Parameter Explanation and Logical Analysis
In the above code, the `SimpleImputer` class is used to fill in missing values. The `strategy='mean'` parameter specifies that the mean of each column is used for filling. Using the `fit_transform` method, the model first fits the dataset to calculate the mean of each column, and then these means are used to fill in the missing values.
### 3.1.2 Anomaly Detection and Handling
Anomalies can be errors in data entry or may be part of natural variation. Correctly identifying and handling anomalies is one of the key steps in preprocessing.
#### Example Code
```python
from sklearn.ensemble import IsolationForest
import numpy as np
# Assuming X is the feature matrix
clf = IsolationForest(n_estimators=100, contamination=0.01)
scores_pred = clf.fit_predict(X)
outliers = np.where(scores_pred == -1)
```
#### Parameter Explanation and Logical Analysis
In this code snippet, the `IsolationForest` class is used for anomaly detection. `n_estimators=100` specifies that 100 trees are used for detection, and `contamination=0.01` indicates that it is expected that 1% of the data are anomalies. The `fit_predict` method trains the model and predicts whether each data point is an anomaly, and the return value of -1 indicates an anomaly.
## 3.2 Feature Selection and Extraction
### 3.2.1 Univariate Feature Selection
Univariate feature selection selects features by examining the statistical relationship between each feature and the labels. This method is simple and effective, especially when the dataset is large.
#### Example Code
```python
from sklearn.feature_selection import SelectKBest, f_classif
# Assuming X is the feature matrix, y is the label vector
selector = SelectKBest(score_func=f_classif, k=10)
X_new = selector.fit_transform(X, y)
```
#### Parameter Explanation and Logical Analysis
The `SelectKBest` class is used to select the most important k features. `score_func=f_classif` specifies that the ANOVA F-value is used as the scoring function, which is suitable for classification problems. `k=10` indicates that the top 10 features with the highest scores are selected. The `fit_transform` method fits the feature selector and returns the new feature matrix
0
0
相关推荐
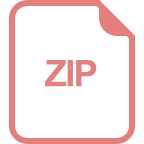
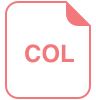
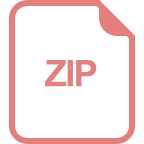
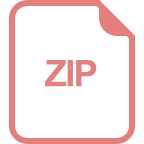
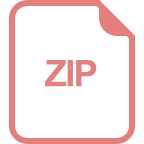
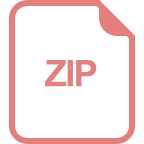
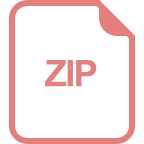
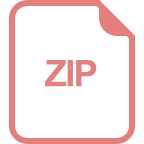