Best Practices for Model Deployment: 5 Steps to Ensure Your Model Runs Steadily
发布时间: 2024-09-15 11:29:51 阅读量: 34 订阅数: 32 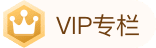
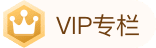
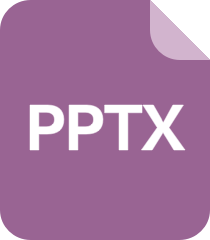
BRK2255 - OneNote best practices for your organization.pptx
# Model Deployment Best Practices: 5 Steps to Ensure Stable Model Operation
## Overview
Model deployment is the essential process of transforming machine learning models into actual applications. It is a critical step in the entire model lifecycle, involving careful considerations of technology, tools, and processes.
## Importance
The quality of the deployment process directly affects the performance and scalability of the model. A good deployment strategy ensures that the model runs stably in different environments and meets the business needs for real-time processing and resource efficiency.
## Key Steps
Pre-deployment preparations include model testing, optimization, and security evaluation. Specific operations involve model format conversion, performance optimization, and adaptability testing for both hardware and software environments.
```markdown
### Model Format Conversion Example
Before deployment, it is often necessary to convert the model from one format to another to accommodate different runtime environments. For instance, converting a trained TensorFlow model to the ONNX format to adapt to edge computing devices.
```python
import onnx
import tensorflow as tf
# Load TensorFlow model
model = tf.keras.models.load_model('path/to/your/model.h5')
# Convert the model to ONNX
tf_rep = tf2onnx.convert.from_keras(model)
onnx.save(tf_rep, 'model.onnx')
```
### Hardware Acceleration Technologies
For applications requiring high-performance computing, hardware acceleration technologies like GPUs, TPUs, or FPGA chips can provide significant speed improvements during model deployment.
```markdown
## Code Explanation
### Performance Optimization Strategies
Performance optimization strategies may include but are not limited to:
- Model pruning and compression to reduce computation
- Utilization of hardware acceleration technologies, such as GPUs
- Software optimization methods, such as quantization and parallel computing
- Compatibility testing to ensure consistent model behavior across environments
### Identifying Compatibility Issues
Compatibility issues might include:
- Incompatibilities between the model and the target platform versions
- Missing or inconsistent dependencies required for the model's runtime environment
These issues typically require identification and resolution through a detailed testing process.
```
In subsequent chapters, we will explore how to prepare and optimize models for deployment, the specifics of setting up the deployment environment, and how to monitor and maintain models. Each part is a critical element in achieving successful deployment, providing IT professionals with in-depth theoretical and practical guidance.
# 2. Model Preparation and Optimization
## 2.1 Preparations for the Model
### 2.1.1 Model Pruning and Compression
Model pruning and compression are key steps in optimizing the size of machine learning models and improving their operational efficiency. Model pruning involves removing redundant or unimportant parameters, while model compression includes applying specific techniques to reduce the overall size of the model. These methods help reduce the computational complexity of the model, lower storage requirements, and maintain performance as much as possible.
- **Pruning**
- **Technical Principle**: Reduces model complexity by removing certain connections with smaller weights in the neural network, retaining only those connections that most affect the model's performance.
- **Operational Steps**: First determine the pruning ratio, ***mon methods include L1 regularization and sensitivity-based pruning.
- **Weight Sharing**
- **Technical Principle**: By sharing weights, multiple neurons use the same parameters to reduce the number of model parameters.
- **Operational Steps**: Analyze the model structure to find layers that can share weights and then modify the network structure to allow these layers' weights to be shared by all relevant neurons.
- **Quantization**
- **Technical Principle**: Converts model weights and activations from floating-point representations to lower precision representations (like integers) to reduce the model size and computational requirements.
- **Operational Steps**: Use a series of algorithms to map floating-point values to a smaller range of bit values. Quantization-aware training is typically used during the training process to adapt the model to quantized weights.
For example, here is how to perform simple pruning using the `torch` library in Python code:
```python
import torch
# Assume net is a pretrained model
def prune_model(net, amount_to_prune=0.1):
# For each layer
for name, module in net.named_children():
# This is just an example; in practice, weights would be selected based on size
if len(module.weight) > 100 and 'conv' in name:
# Select the smallest weights for pruning
prune_target = module.weight.data.abs().argmin()
prune_amount = int(amount_to_prune * len(module.weight))
module.weight.data = torch.cat((module.weight.data[:prune_target],
module.weight.data[prune_target + prune_amount:]))
print(f'Pruning {prune_amount} weights from layer {name}')
return net
```
### 2.1.2 Model Format Conversion
Converting trained models into deployment-ready formats such as ONNX, TensorRT, or OpenVINO not only optimizes model performance but also enhances deployment flexibility.
- **ONNX (Open Neural Network Exchange)**
- **Technical Principle**: ONNX provides a common format that allows model conversion between different deep learning frameworks.
- **Operational Steps**: Use the tools provided by the framework, such as `torch.onnx.export`, to export the model to ONNX format.
- **TensorRT**
- **Technical Principle**: Offered by NVIDIA, TensorRT optimizes models through techniques like layer fusion and kernel auto-tuning.
- **Operational Steps**: Use the TensorRT API to optimize and serialize the model.
- **OpenVINO**
- **Technical Principle**: Provided by Intel, OpenVINO optimizes deep learning models to run on Intel hardware.
- **Operational Steps**: Use the Model Optimizer to convert the model into IR (Intermediate Representation), then deploy with the Inference Engine.
## 2.2 Model Performance Optimization Strategies
### 2.2.1 Hardware Acceleration Technologies
Hardware acceleration technologies, such as GPU acceleration, TPU usage, and specialized hardware like FPGAs and ASICs, can greatly enhance the computational performance of machine learning models.
- **GPU Acceleration**
- **Technical Principle**: Uses GPUs for parallel computing, significantly improving efficiency in scenarios with large data volumes and complex operations.
- **Operational Steps**: Construct and train models using deep learning frameworks that support GPU acceleration (such as TensorFlow or PyTorch).
- **TPU (Tensor Processing Unit)**
- **Technical Principle**: A processor developed by Google, optimized specifically for machine learning tasks.
- **Operational Steps**: When using TensorFlow, specify TPUs as the computing resource for model training and inference.
### 2.2.2 Software Optimization Methods
At the software level, improving model performance through algorithm selection, optimization, and code-level optimization is also crucial.
- **Algorithm Optimization**
- **Technical Principle**: Choosing the appropriate algorithms and model structures can reduce computational load and increase running speed.
- **Operational Steps**: Select the optimal algorithms based on the type of problem and the characteristics of the data.
- **Parallel Computing and Multithreading**
- **Technical Principle**: Utilize the multi-core capabilities of modern CPUs to enhance performance through parallel computing and multithreading.
- **Operational Steps**: Use parallel computing libraries like OpenMP, MPI, or multithreading libraries like Python's `threading` and `multiprocessing`.
## 2.3 Model Compatibility Testing
### 2.3.1 Identifying Compatibility Issues
Model compatibility issues may stem from differences between deep learning frameworks and inconsistencies in system environments.
- **Framework Differences**
- **Analysis**: Different deep learning frameworks may have discrepancies in numerical computations and function implementations.
- **Solution**: Use cross-framework tools for compatibility testing before model conv
```
0
0
相关推荐
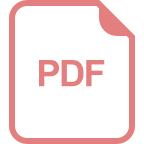
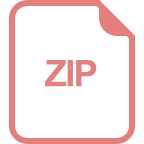
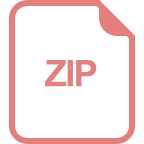
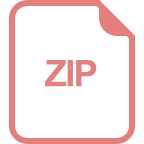
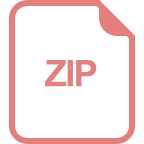
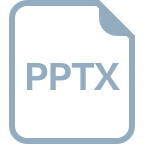
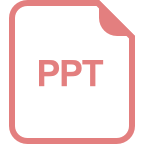