Time Series Causal Relationship Analysis: An Expert Guide to Identification and Modeling
发布时间: 2024-09-15 07:00:37 阅读量: 67 订阅数: 27 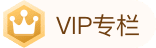
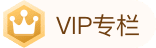
# 1. Overview of Machine Learning Methods in Time Series Causality Analysis
In the realm of data analysis, understanding the dynamic interactions between variables is key to time series causality analysis. It goes beyond mere correlation, focusing instead on uncovering the underlying causal connections. Thanks to their unique temporal dimension, time series data offer rich information for observing causal effects. In this chapter, we will introduce time series causality analysis, discussing its definition, importance, and potential value in real-world applications. Furthermore, this chapter aims to lay a solid foundation for understanding subsequent chapters.
## 1.1 Characteristics of Time Series Data
Time series data is a series of observations arranged in chronological order, typically used for analyzing and predicting trends and patterns that evolve over time. Its characteristics include temporal correlation, seasonality, and trend. It is precisely these features that, when combined with causal relationship exploration, reveal the dynamic causal chains between different events or variables.
## 1.2 Necessity of Causal Relationship Analysis
In disciplines such as economics, biology, and sociology, correctly understanding the causal relationships between variables is crucial for prediction and decision-making. However, finding true causal relationships in observational data is often more complex than it appears. This section will explore why traditional correlation analysis falls short and emphasize the importance of causal relationship analysis across various scientific fields.
## 1.3 Prospects for Applying Time Series Causality Analysis
Time series causality analysis not only helps to uncover causal pathways between variables but also has broad application prospects in fields such as financial risk management, economic policy-making, and disease control. This section will briefly introduce some specific application scenarios to inspire readers' interest in further studying causal relationship analysis.
# 2. Theoretical Foundations of Time Series Causality
## 2.1 Definition and Importance of Causality
### 2.1.1 Differences Between Causality and Correlation
Causality and correlation are two foundational concepts in statistics and data analysis. They differ markedly in definition and are often confused in practice. Correlation describes the strength and direction of the relationship between two variables, but it does not indicate whether one variable causes another. For instance, in weather forecasting, the relationship between air pressure and weather changes has a high correlation, but this does not mean that changes in air pressure directly cause changes in the weather.
In contrast, causality emphasizes that after one event (the cause) occurs, another event (the effect) follows. In other words, a causal relationship requires a temporal sequence and a logical necessity. If an event does not occur, the subsequent event will not occur either, and such a relationship can be considered causal. For example, if an area increases its afforestation efforts, and subsequently, the air quality and soil conservation in that area improve, then afforestation and environmental improvement can be considered a causal relationship.
### 2.1.2 History and Methodology of Causal Inference
The history of causal inference dates back to the early 20th century, when statistical methods began attempting to distinguish between correlation and causality. By the mid-20th century, statisticians had developed more complex mathematical models to explain this relationship. One of the most famous theories is the "Granger Causality" proposed by economist David Hendry, which identifies causal relationships between variables through time series data analysis.
Subsequently, Judea Pearl's causal diagram models (Causal Diagrams) and structural equation models (SEMs) provided a more solid theoretical foundation for causal inference. Pearl's models interpret causal relationships as structures where variables are connected by directed edges, indicating a direct or indirect influence of one variable on another.
In terms of methodology, econometric techniques such as instrumental variables (IV) and difference-in-differences (DID) are commonly used causal inference methods. In recent years, Bayesian networks, latent variable models, and machine learning methods like random forests and deep learning have been introduced into causal relationship analysis, providing new insights and tools.
## 2.2 Types of Causal Models
### 2.2.1 Linear and Nonlinear Causal Models
Among causal model classifications, linear causal models are the most basic and common form. They assume that the relationship between variables can be described by a linear equation. For example, a simple time series causal model can be expressed as:
\[ Y_t = \beta_0 + \beta_1 X_t + \varepsilon_t \]
where \( Y_t \) is the outcome variable, \( X_t \) is the cause variable, \( \beta_1 \) represents the magnitude of the causal effect, and \( \varepsilon_t \) is the error term.
However, not all causal relationships are linear. Nonlinear causal models allow the relationship between outcome and cause variables to vary with different values of the variables, making them more suitable for describing interactions in complex systems. Examples include polynomial regression models, neural network models, and certain types of nonlinear difference equations.
### 2.2.2 Static and Dynamic Causal Models
Static models generally describe the causal relationship between variables at a particular moment or in the short term, ignoring the impact of time factors on variable relationships. In contrast, dynamic causal models consider the dynamic changes of variables over time, typically involving lag effects, cumulative effects, and feedback mechanisms.
For example, in financial markets, a dynamic causal model might consider the historical performance of an investment strategy on current decision-making. Dynamic models often use time series analysis methods such as autoregressive models (e.g., ARIMA) or difference equations to build.
## 2.3 Methods for Identifying Causality
### 2.3.1 Granger Causality Test
The Granger causality test is a widely used statistical method for testing whether one time series can provide predictive information about another time series. If adding a time series improves the prediction of another time series, given other relevant variable information, then it is considered that the former Granger causes the latter.
The steps for conducting a Granger causality test are roughly as follows:
1. Check the stationarity of the series; if not stationary, difference.
2. Build a vector autoregressive model (VAR) ***
***pare the restricted VAR model (excluding certain variables) with the unrestricted VAR model (including all variables).
4. Use the F-test to determine if it is statistically significant to reject the null hypothesis.
An important limitation of the Granger causality test is that it cannot provide a true causal relationship, only indicating whether one variable can statistically predict another variable's changes.
### 2.3.2 Causal Diagrams and Structural Equation Models
Causal diagrams represent causal relationships between variables graphically, with nodes representing variables and directed edges representing causal relationships. Structural equation models combine regression analysis and factor analysis, describing direct and indirect effects between variables.
The typical steps for using causal diagrams and structural equation models for causal inference are as follows:
1. Define the causal diagram and determine the causal relationships between variables.
2. Extract the structural equation model from the diagram.
3. Estimate model parameters using observed data.
4. Perform goodness-of-fit tests and hypothesis testing on the model.
This method allows for a more intuitive understanding of causal paths in complex systems, especially when dealing with systems that
0
0
相关推荐
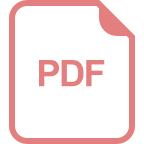
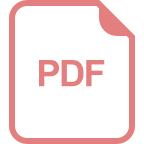
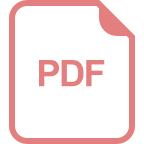
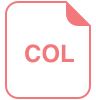
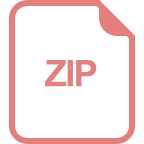
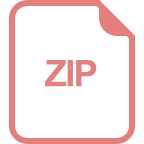
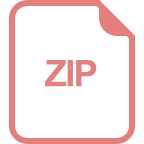
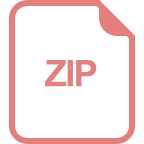
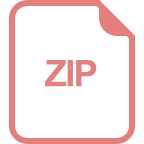