Vector Autoregression Model VAR in Time Series: Application and In-Depth Case Analysis
发布时间: 2024-09-15 07:06:20 阅读量: 81 订阅数: 29 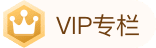
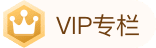
# Chapter 1: Overview of Vector Autoregression (VAR) Model in Time Series Prediction
Time series analysis is a critical tool in data analysis across fields such as economics and finance. Among various models, the Vector Autoregression (VAR) model has gained attention for its ability to capture the dynamic relationships between multiple time series. VAR models are not only capable of handling autoregressive problems of single variables but can also analyze the dynamic characteristics of interdependence among different variables in a multivariate environment. This approach offers researchers a new way to comprehensively analyze interactions between economic indicators and financial market data. With VAR models, we can better predict future trends of variables and provide decision support for policymakers and investors. This chapter aims to provide a foundational introduction to the VAR model, laying the groundwork for readers to understand subsequent theoretical and practical applications.
# Chapter 2: Theoretical Foundations of VAR Models
## 2.1 Mathematical Principles of VAR Models
### 2.1.1 Definition and Mathematical Expression of VAR Models
Vector Autoregression (VAR) models are multivariate time series models that express each variable in a system as a linear function of its past values and current values. In mathematical form, VAR models can be expressed as:
```
y_t = c + Π_1 y_{t-1} + Π_2 y_{t-2} + ... + Π_p y_{t-p} + ε_t
```
Here, `y_t` is a k-dimensional time series vector, `c` is a constant vector, `Π_1, Π_2, ..., Π_p` are parameter matrices, `p` represents the lag order of the model, and `ε_t` is an error vector assumed to be uncorrelated at different time points. The goal of the model is to estimate these parameters using data.
The effectiveness of VAR models largely depends on the stationarity of the data. If time series data is non-stationary, it typically needs to be transformed into a stationary series through differencing or other methods.
#### Code Block Presentation
Assuming we have a time series dataset `ts_data`, we use the R language to estimate a simple VAR model, with the following execution logic and parameter explanations:
```r
# Load the vars package for VAR model estimation
library(vars)
# Assume ts_data is a time series dataset
# Here we use the VAR function for estimation, with the lag order p set to 2
var_model <- VAR(ts_data, p = 2, type = "const")
# View estimation results
summary(var_model)
```
### 2.1.2 Parameter Estimation Methods
Parameter estimation typically uses the Maximum Likelihood Estimation (MLE) method. First, we define the likelihood function:
```
L(Π_1, Π_2, ..., Π_p; y) = ∏_{t=p+1}^T f(y_t | y_{t-1}, ..., y_{t-p})
```
where `f` is the probability density function of the multivariate normal distribution. Maximizing the likelihood function is equivalent to minimizing the negative log-likelihood function, which is the same as minimizing the residual sum of squares (RSS).
#### Code Block Presentation
In R, using the `VAR` function actually calls the maximum likelihood estimation method. Here's how to implement it in code:
```r
# Using the VAR function with default parameters will call the maximum likelihood estimation method
var_model <- VAR(ts_data, p = 2, type = "const")
```
### 2.2 Identification and Testing of VAR Models
#### ***
***mon testing methods include:
- Unit root tests (such as the ADF test)
- KPSS test
#### Code Block Presentation
In R, you can use the `urca` package to perform an ADF test:
```r
library(urca)
# Perform an ADF test on the data to ensure stationarity
adf_test <- ur.df(ts_data, type = "drift", lags = 0)
summary(adf_test)
```
#### 2.2.2 Determining the Lag Order of the Model
The lag order of VAR models is commonly determined using information criteria (such as AIC, BIC) or lag order tests (such as the LR test).
#### Code Block Presentation
Here's how to determine the lag order using the `VARselect` function in R:
```r
# Use the VARselect function to determine the optimal lag order
lag_order <- VARselect(ts_data, type = "const")
lag_order$selection
```
#### 2.2.3 Granger Causality Tests
Granger causality tests are used to determine whether one time series can predict another.
#### Code Block Presentation
In R, you can use the `grangertest` function to perform Granger causality tests:
```r
# Perform Granger causality tests on two time series
granger_test <- grangertest(ts_data[, "series1"], ts_data[, "series2"])
granger_test
```
The identification and testing of VAR models are crucial steps in building an effective model. Only by ensuring the accuracy and applicability of the model can further diagnostic tests, optimization, and subsequent practical applications be carried out.
# Chapter 3: Practical Operation of VAR Models
## 3.1 Implementing VAR Models Using Statistical Software
### 3.1.1 Steps to Implement VAR Models in R Language
Implementing a VAR model in R involves steps such as data preprocessing, model specification, estimation, and validation. This section will detail how to use relevant packages in R to build and analyze VAR models.
First, we need to install and load the `vars` package, which contains various functions for VAR model analysis. Then, follow these steps:
```r
# Install and load the vars package
install.packages("vars")
library(vars)
# Assume we already have time series data ts_data
# Set the lag order of the VAR model
p <- 2 # Taking a 2nd order VAR model as an example
model_var <- VAR(ts_data, p = p, type = "const")
# View the model summary
summary(model_var)
```
In the code block above, `ts_data` is a hypothetical time series data frame. In actual operations, you need to replace this with your data. The `VAR` function is used to estimate the VAR model, where the `p` parameter specifies the lag order of the VAR model, and `type = "const"` indicates that the model includes a constant term.
After model fitting, the `summary` function can be used to view a detailed summary of the model, including coefficient estimates and diagnostic test results. Based on the summary content, further analysis and optimization of the model can be performed.
### 3.1.2 Steps to Implement VAR Models in Python
The `statsmodels` library in Python provides a rich set of tools for handling time series data, including VAR models. Here are the basic steps to implement VAR models using Python:
First, ensure that the `statsmodels` library is installed, and then import the necessary modules:
```python
# Install and import the statsmodels library
import statsmodels.api as sm
# Assume we already have time series data ts_data
# Set the lag order of the VAR model
p = 2 # Taking a 2nd order VAR model as an example
model_var = sm.tsa VAR(ts_data, p)
# Estimate the model
results = model_var.fit(maxlags=p, ic='aic')
# Display the model summary
print(results.summary())
```
In this Python code, `ts_data` is a hypothetical time series dataset. You need to replace this with your actual data. The `VAR` function is used to fit the VAR model, where `maxlags=p` specifies the lag order of the VAR model, and `ic='aic'` is used to select the optimal lag order (options include 'AIC', 'BIC', 'HQIC').
The `summary` method of the `results` object can be used to print detailed summary information of the model for further analysis and validation of the model's applicability.
## 3.2 Model Diagnostic Testing and Optimization
### 3.2.1 Residual Analysis
Residual analysis is an essential step in examining the goodness of fit for time series models. The residuals of VAR models should be close to white noise sequences, meaning there is no autocorrelation between residuals and no heteroskedasticity.
In R language, we can use the `serial.test` function to perform the Ljung-Box test for autocorrelation:
```r
serial.test(model_var, lags.pt=10, type="Ljung-Box")
```
This function tests the autocorrelation of the residual series and reports the Ljung-Box Q statistic. If the p-value is large, the null hypothesis of white noise cannot be rejected.
In Python, the `diagnostics` method is used to perform similar tests:
```python
results.diagnostics()
```
This method returns a chart containing various diagnostic information, which can visually show whether the residual series has issues such as autocorrelation or heteroskedasticity.
### 3.2.2 Model Stability Testing
The stability of VAR models requires that all characteristic roots be inside the unit circle. Stability testing can be achieved by checking the reciprocal of the roots to ensure that the modulus of all reciprocal roots is less than 1.
In R, we can directly use the `roots` function to calculate and test the model's characteristic roots:
```r
roots(model_var)
```
The output results will give the reciprocal of each characteristic root. If the modulus of all reciprocal roots is less than 1, the model is stable.
In Python, although `statsmodels` does not provide a similar function directly, this test can be manually implemented by calculating the eigenvalues and
0
0
相关推荐
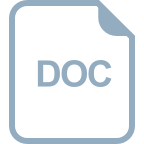
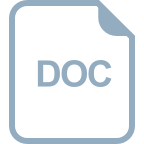
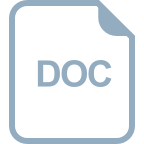
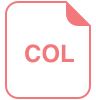
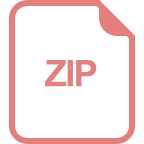
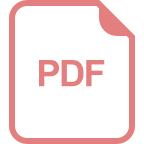
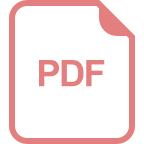
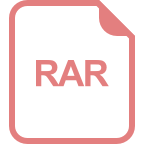
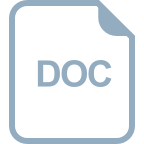