"Random Forest Time Series Forecasting": Theoretical Depth and Practical Guide
发布时间: 2024-09-15 06:48:42 阅读量: 45 订阅数: 42 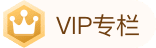
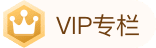
# Random Forest Time Series Forecasting: Theoretical Depth and Practical Guide
## 1. Overview of Random Forest Algorithm
The Random Forest algorithm is an ensemble learning technique composed of multiple decision trees, designed to improve predictive accuracy and prevent overfitting. In this chapter, we will explore the origins of Random Forest, its status in machine learning, and how it handles classification and regression tasks.
### 1.1 Core Concepts of Random Forest
Random Forest enhances a model's generalization capabilities by introducing randomness. The core idea is to create a forest of multiple decision trees, each trained on only a subset of the data. This diversity helps the model exhibit greater robustness when facing new data.
### 1.2 Brief Explanation of Random Forest's Mechanism
Each tree independently learns the relationship between data features and labels, ultimately determining the prediction result through a voting mechanism. This ensemble method not only improves model performance but also simplifies model tuning and interpretation.
### 1.3 Application Domains and Advantages
Random Forest is widely used in financial analysis, bioinformatics, natural language processing, and other fields due to its efficiency and flexibility. It shows unique advantages in dealing with high-dimensional data and interactions between features, making it a powerful tool for data scientists.
The following chapters will delve into the Random Forest algorithm and its applications and optimization strategies in time series forecasting.
## 2. Fundamentals of Time Series Forecasting
Time series analysis is one of the key techniques for understanding and forecasting future events, with widespread applications in economics, finance, meteorology, and technology. This chapter first discusses the basic theory of time series analysis, then introduces how to preprocess time series data, and finally compares different time series forecasting methods.
### 2.1 Theories of Time Series Analysis
#### 2.1.1 Components of a Time Series
A time series is a sequence of data points arranged in chronological order, usually used to represent changes in a variable at different points in time. Time series analysis focuses on the temporal characteristics of the data, which are crucial for forecasting future data points. A time series typically includes the following elements:
- **Trend**: The long-term direction of change in the time series data over time. Trends can be rising, falling, or stable.
- **Seasonality**: Periodic fluctuations that occur within fixed time intervals (such as seasons, months, weeks, etc.).
- **Cyclical**: Fluctuations that do not have a fixed period but typically have a cycle of more than a year.
- **Irregular/Random**: The remaining fluctuations, caused by unexpected events or random disturbances, which are difficult to predict.
Understanding these elements is a prerequisite for time series analysis. For instance, when forecasting a company's quarterly sales, one would consider past sales trends, seasonality (such as increased sales during the holiday season), and potential cyclical changes (such as the impact of economic cycles on sales).
#### 2.1.2 Common Time Series Models
In time series analysis, there are various models that can be used to describe and predict data. These models include:
- **Autoregressive Model (AR)**: Predicts future values using lagged values of the time series itself.
- **Moving Average Model (MA)**: Uses historical disturbances or residuals of the time series to predict future values.
- **Autoregressive Moving Average Model (ARMA)**: Combines the advantages of AR and MA models by considering both the lagged values and historical disturbances of the time series.
- **Autoregressive Integrated Moving Average Model (ARIMA)**: When the time series is non-stationary, it is first transformed into a stationary series, and then the ARMA model is applied.
- **Seasonal Autoregressive Integrated Moving Average Model (SARIMA)**: Adds seasonal component analysis on the basis of ARIMA.
- **Exponential Smoothing Model**: Assigns different weights to historical data, with more recent data being given higher weight.
Each model has its own scenarios and limitations, and choosing the appropriate model is crucial for the accuracy of the forecasts.
### 2.2 Preprocessing Time Series Data
Before conducting time series analysis, it is essential to thoroughly preprocess the data to ensure the accuracy and reliability of the analysis results.
#### 2.2.1 Data Cleaning
Data cleaning involves identifying and addressing inconsistencies, missing values, and outliers within the time series data. Effective data cleaning can improve the accuracy of the model'***mon steps include:
- **Filling Missing Values**: If the amount of missing data is small, methods such as forward-filling, backward-filling, or interpolation can be used to fill in the gaps.
- **Outlier Handling**: Identify outliers in the data and decide whether to remove, correct, or retain these values.
- **Smoothing**: Use moving averages or other methods to smooth data and reduce the impact of random fluctuations.
#### 2.2.2 Data Transformation and Smoothing
To eliminate trends and seasonality or to make the time series平稳, data transformation and smoothing are often necessary. These methods include:
- **Log Transformation**: Reduces the heteroscedasticity of data, making fluctuations more stable.
- **Differencing**: Eliminates trends by calculating the difference between data points and their previous values.
- **Seasonal Differencing**: Conducts differencing over the seasonal period to remove seasonal effects.
- **Moving Average Smoothing**: Calculates the moving average over a window to reduce random fluctuations.
### 2.3 Comparison of Time Series Forecasting Methods
When selecting a time series forecasting method, several factors such as the characteristics of the data, the accuracy of the forecasts, and the complexity of the computations need to be considered.
#### 2.3.1 Statistical Methods vs. Machine Learning Methods
- **Statistical Methods**: Traditional statistical models like ARIMA and exponential smoothing are widely used due to their strong interpretability and relatively low computational complexity. These models perform well on small to medium-sized datasets, especially when the time series data is linear or can be linearized.
- **Machine Learning Methods**: With the development of machine learning technology, models like Random Forest, Support Vector Machines (SVM), and neural networks are also used for time series forecasting. These models excel in capturing non-linear and complex patterns, but they typically require more data and computational resources and have poorer model interpretability.
#### 2.3.2 Factors to Consider in Model Selection
- **Data Scale and Complexity**: Large-scale, non-linear time series data is more suitable for machine learning methods.
- **Forecasting Accuracy**: Machine learning methods usually outperform statistical methods in terms of accuracy, but overfitting risks need to be monitored.
- **Computational Resources and Time**: Statistical methods are computationally more efficient and suitable for environments with limited resources.
- **Model Interpretability**: If the forecast results need to be explained, statistical models may be more appropriate.
The above are some fundamental points of time series forecasting. In the following chapters, we will delve deeper into the Random Forest algorithm and its application in time series forecasting.
# 3. Detailed Explanation of Random Forest Algorithm
As a powerful machine learning method, the Random Forest algorithm has shown excellent performance in handling classification and regression problems. In the field of time series forecasting, it has gradually become a research hotspot. This chapter will delve into the
0
0
相关推荐
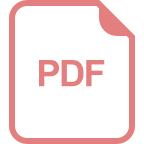
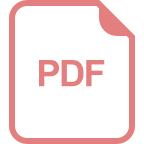
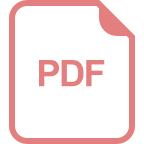





