Time Series Forecasting with Sliding Window Technique: Dynamic Prediction and Case Analysis
发布时间: 2024-09-15 06:53:38 阅读量: 28 订阅数: 43 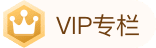
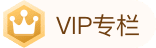
# Time Series Forecasting with Sliding Window Technique: Dynamic Prediction and Case Study
## 1. Theoretical Foundations of Time Series Forecasting
### 1.1 Overview of Time Series Forecasting
Time series forecasting leverages historical time series data to predict values or distributions of values at future points in time. This technology is widely used in finance, economics, meteorology, and other fields. The theoretical foundations include knowledge from statistics, machine learning, and other disciplines, involving core concepts such as data stationarity, periodicity, trendiness, and noise handling.
### 1.2 Statistical Models and Machine Learning Approaches
Statistical models such as ARIMA and seasonal decomposition are classical methods of time series forecasting, focusing on the mathematical properties of data. Machine learning methods like random forests and gradient boosting trees improve prediction accuracy by learning nonlinear relationships in samples. Currently, deep learning models that combine the advantages of statistics and machine learning (such as LSTMs) are also widely used in time series forecasting.
### 1.3 Evaluation Metrics for Time Series Forecasting
The evaluation of time series forecasting models typically involves error measurement metrics such as Mean Squared Error (MSE), Root Mean Squared Error (RMSE), and Mean Absolute Error (MAE). These metrics help quantify the accuracy of model predictions, allowing for comparisons between models and optimization of the model itself.
# 2. Detailed Explanation of the Sliding Window Technique
## 2.1 Concepts and Characteristics of the Sliding Window
### 2.1.1 Basic Definition of the Sliding Window
The sliding window is a technique used for time series analysis and forecasting. It involves dividing time series data into continuous, overlapping segments. Each segment contains a fixed number of observations and slides along the time axis, capturing local data features dynamically and enabling predictive analysis.
In the sliding window, "window" can be considered a fixed-size dataset that slides over the time series data with a set step size. With each slide, a new set of data points is included and an old set is removed. This mechanism allows the sliding window technique to fully utilize the time dependency and local characteristics of time series data, enhancing the precision and efficiency of the prediction model.
### 2.1.2 Applicable Scenarios for the Sliding Window Technique
The sliding window technique is particularly suitable for data with time dependencies, such as stock prices, meteorological data, network traffic, and energy consumption. In these data sets, recent observations often have higher indicative value for future predictions. Therefore, the sliding window can improve the model's ability to predict short-term trends by focusing on recent data segments.
Furthermore, the sliding window technique is widely used in real-time data analysis and online prediction systems. In these scenarios, the model needs to update predictions in real-time based on the latest data. The sliding window can dynamically update the dataset, enabling the model to quickly adapt to changes in data, thereby providing continuous and accurate prediction services.
## 2.2 Operational Methods of the Sliding Window
### 2.2.1 Setting and Adjusting Window Size
Window size is one of the most critical parameters in the sliding window technique. It determines the number of data points in the window, thus affecting the model's ability to capture the temporal characteristics of the data. Setting an appropriate window size is crucial for improving model performance.
The setting of window size depends on the specific application scenario and data characteristics. Generally, the window size should be large enough to capture sufficient data points to grasp the statistical properties of the time series but not too large to introduce excessive noise or irrelevant data. In practice, the choice of window size often needs to be optimized and adjusted through methods such as cross-validation.
### 2.2.2 Strategies and Impacts of Window Sliding
The sliding strategy of the sliding window includes the choice of step size and the determination of the sliding method. The step size determines the number of data points covered with each slide of the window. If the step size is too large, the window may miss important data changes; if the step size is too small, the model may repeatedly process a large amount of the same data.
There are usually two types of sliding methods: one where the window slides over a single data point each time, and another where it covers multiple data points. The choice of method depends on the data sampling frequency and the requirements of the prediction task. In some cases, more complex settings may be needed for the sliding strategy to balance computational efficiency and prediction accuracy.
## 2.3 Combining the Sliding Window with Time Series Models
### 2.3.1 Application of the Sliding Window in ARIMA Models
The Autoregressive Integrated Moving Average (ARIMA) model is one of the most commonly used forecasting models in time series analysis. The sliding window technique can be combined with the ARIMA model by training an ARIMA model within each window to achieve local prediction of time series data.
When applying the sliding window technique, the parameters of the ARIMA model can be dynamically adjusted based on the local characteristics of the data. For example, methods like grid search can be used to optimize the model parameters within each window to improve the prediction accuracy of the model in that window. In this way, the sliding window technique can enhance the ARIMA model's responsiveness to local changes in the time series.
### 2.3.2 Application of the Sliding Window in Machine Learning Models
The sliding window technique can also be applied to various machine learning models, such as random forests, support vector machines (SVM), and neural networks. In these models, the sliding window can be used to generate training samples, where each window provides a set of data features and corresponding labels (i.e., observations at some future point in time) for a specific time range.
Through the sliding window technique, a large number of training samples can be provided to machine learning models, thus training a prediction model capable of capturing the dynamic changes in the time series. Additionally, the sliding window can be used in online learning scenarios where the model continuously receives new data and updates based on the latest data within the sliding window, achieving real-time predictions.
Through the introduction of this chapter, we understand the importance and basic applications of the sliding window technique in time series analysis. The following chapters will detail how to build a dynamic time series forecasting model, which will require a deeper understanding and practice of the sliding window technique and prediction models.
# 3. Building a Dynamic Time Series Forecasting Model
In the field of time series forecasting, dynamic mode
0
0
相关推荐
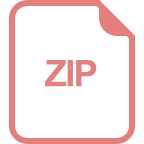
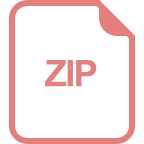
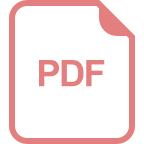
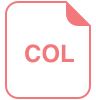
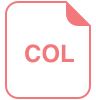
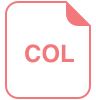
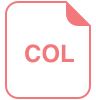

