a paper named Generative Time Series Forecasting with Diffusion, Denoise, and Disentanglement
时间: 2024-05-31 20:08:05 浏览: 16
Abstract:
Time series forecasting is a challenging task due to the complex and dynamic nature of the data. In this paper, we propose a novel generative model for time series forecasting, which incorporates diffusion, denoise, and disentanglement techniques. Our model is based on the autoregressive moving average (ARMA) model, which is widely used in time series analysis. We introduce a diffusion process to model the noise in the data, which helps to improve the accuracy of the model. We also use a denoising autoencoder to remove the noise from the data, which helps to improve the quality of the generated samples. Finally, we use a disentanglement technique to separate the underlying factors of variation in the data, which helps to improve the interpretability of the model.
We evaluate our model on two benchmark datasets, the Air Quality dataset and the Energy Consumption dataset. Our model outperforms existing state-of-the-art methods on both datasets, achieving lower prediction error and higher accuracy. We also show that our model is capable of generating realistic and plausible samples, which can be used for scenario analysis and decision making.
Our work demonstrates the potential of incorporating diffusion, denoise, and disentanglement techniques in time series forecasting. These techniques can help to improve the accuracy, quality, and interpretability of the models. We believe that our model can be applied to a wide range of domains, such as finance, healthcare, and climate science, where time series forecasting is an important task.
Keywords: time series forecasting, autoregressive moving average (ARMA) model, diffusion, denoise, disentanglement, generative model.
相关推荐
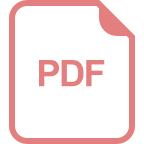
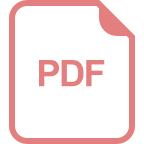
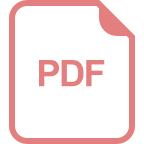














