Time Series Forecasting Model Selection: An Expert Guide to Finding the Best Approach
发布时间: 2024-09-15 06:26:28 阅读量: 36 订阅数: 29 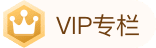
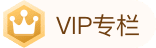
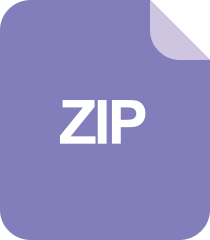
multivariate-timeseries-forecasting:一组用于多元时间序列预测的算法
# Machine Learning Methods in Time Series Forecasting
## Theoretical Foundations of Time Series Forecasting
### Concepts and Importance of Time Series
Time series forecasting is a significant branch of data analysis that involves the study of data points arranged in chronological order to predict the values of future data points. Time series data can be continuous, such as stock price fluctuations every second, or discrete, such as monthly sales figures.
In the IT industry, time series forecasting is widely applied to business demand forecasting, network traffic analysis, and energy consumption forecasting, among other areas. Accurate time series analysis empowers businesses to make more scientific decisions, optimize resource allocation, and enhance operational efficiency.
### Key Elements of Time Series Forecasting
The critical elements of time series forecasting include Trend, Seasonality, Cyclicity, and Irregular Component. Understanding these elements is crucial for building an accurate predictive model.
- **Trend** reflects the long-term movement of data, whether it is increasing or decreasing over time.
- **Seasonality** refers to the pattern of data repeating periodically at fixed intervals.
- **Cyclicity** is similar to seasonality but describes periodic fluctuations at non-fixed time intervals.
- **Irregular Component** refers to the impact of random fluctuations or unexpected events on the data.
### Methodologies in Time Series Forecasting
Time series forecasting methods are primarily divided into two categories: quantitative and qualitative. Quantitative methods include statistical models such as ARIMA and exponential smoothing methods, while qualitative methods rely more on expert experience and judgment.
In subsequent chapters, we will delve into specific time series forecasting models and understand how to select and apply these models in practice. Next, we will elaborate on how to use time series data for forecasting through illustrative examples.
# Practical Application and Application of Time Series Forecasting Models
### Understanding the Importance of Time Series Forecasting
In the field of data science, time series forecasting is one of the core issues because it can assist enterprises and organizations in decision-making, predicting market trends, and managing inventory, among other things. Understanding the practical application of time series forecasting models can help us better understand the trends and patterns of data changes and make reasonable predictions about future situations.
### Preparations Before Practicing
Before beginning to build a time series forecasting model, some preparations must be made. This includes data collection, data cleaning, and exploratory data analysis. Data collection requires us to determine the data source, data cleaning involves checking for and correcting missing and abnormal values in the dataset, and exploratory data analysis requires us to use statistical charts, such as box plots and line charts, to observe data characteristics, understanding the temporal attributes and seasonal characteristics of the data.
### Construction and Application of Time Series Forecasting Models
The construction of time series forecasting models can employ ARIMA models, seasonal decomposition models, and machine learning models such as Random Forests and Gradient Boosting Decision Trees. During the model construction process, we need to train and test the model, analyze its performance, and then optimize based on the model's predictive results.
#### Example Application of ARIMA Model
Taking the ARIMA model as an example, the ARIMA model is an autoregressive integrated moving average model that can describe the three key components of time series data: the autoregressive part (AR), the difference part (I), and the moving average part (MA).
```python
from statsmodels.tsa.arima.model import ARIMA
# Assume we have a set of time series data data
# Applying the ARIMA model
model = ARIMA(data, order=(1, 1, 1))
model_fit = model.fit()
# Forecasting the data for the next time point
forecast = model_fit.forecast(steps=1)
print(forecast)
```
In the above code, we first imported the ARIMA model, then instantiated it with a set of assumed time series data, setting the model's parameters to one autoregressive term, one difference order, and one moving average term. Next, we called the fit method to train the model and the forecast method to predict the data for the next time point.
#### Example Application of Seasonal Decomposition Model
The seasonal decomposition model can decompose a sequence with obvious seasonal characteristics and predict future seasonal behavior.
```python
from statsmodels.tsa.seasonal import seasonal_decompose
# Perform seasonal decomposition on the data
decomposition = seasonal_decompose(data, model='multiplicative')
# Plot the results of seasonal decomposition
decomposition.plot()
```
### Challenges in Practice
In the practice of time series forecasting models, we will encounter various challenges, such as data non-stationarity, model overfitting, and underfitting. We need to ensure data stability through data differentiation, seasonal adjustment, and other methods, and avoid model overfitting and underfitting through techniques such as cross-validation.
### Summary of This Chapter
Through the introduction of this chapter, we have understood the importance of the practice and application of time series forecasting models, the preparations before model construction, and the practical application examples of ARIMA and seasonal decomposition models. At the same time, we have also realized the challenges that may be encountered in practice and the strategies that need to be adopted. In the next chapter, we will delve deeper into model evaluation, optimization, and advanced applications.
# Evaluation and Selection of Time Series Forecasting Models
## The Importance of Model Evaluation
In the field of time series forecasting, choosing the appropriate model is crucial for the accuracy of the prediction results. Evaluating models allows us to understand the model's fit to historical data and its predictive ability for future data. There are many indicators for measuring model performance, such as Mean Squared Error (MSE), Root Mean Squared Error (RMSE), and Mean Absolute Error (MAE).
### Model Evaluation Indicators
#### Mean Squared Error (MSE)
MSE is the average of the squared prediction errors, and its formula is:
```
MSE = 1/n * Σ (yi - ŷi)²
```
Where n is the number of samples, yi is the true value, and ŷi is the predicted value.
```python
from sklearn.metrics import mean_squared_error
import numpy as np
# Assume y_true is the array of true values, and y_pred is the array of predicted v
```
0
0
相关推荐
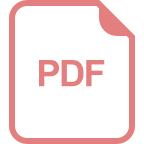
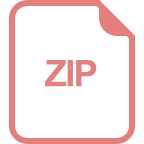
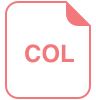
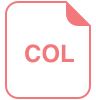
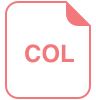
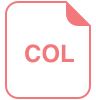
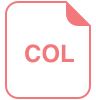
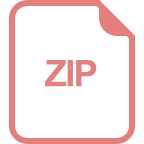