【Advanced】Time Series Forecasting in MATLAB: ARIMA Model
发布时间: 2024-09-13 23:26:52 阅读量: 28 订阅数: 44 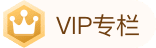
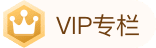
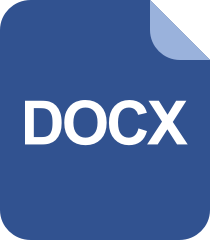
基于STM32单片机的激光雕刻机控制系统设计-含详细步骤和代码
# Advanced篇: Time Series Forecasting in MATLAB: ARIMA Model
## 2.1 Autoregressive Moving Average Model (ARMA)
### 2.1.1 Mathematical Expression of ARMA Model
ARMA is a time series model that represents the time series as a combination of an autoregressive (AR) process and a moving average (MA) process. Its mathematical expression is:
```
X_t = c + ∑(i=1)^p φ_i X_(t-i) + ∑(j=1)^q θ_j ε_(t-j) + ε_t
```
where:
- X_t: the value of the time series at time t
- c: a constant term
- φ_i: the autoregressive coefficients of the AR process, i = 1, 2, ..., p
- θ_j: the moving average coefficients of the MA process, j = 1, 2, ..., q
- ε_t: a white noise term, normally distributed N(0, σ^2)
### 2.1.2 Parameter Estimation of ARMA Model
The parameters (φ_i, θ_j, σ^2) of the ARMA model can be estimated using maximum likelihood estimation (MLE) or least squares estimation (LSE).
**Maximum Likelihood Estimation:**
```
L(φ, θ, σ^2) = -n/2 log(2πσ^2) - 1/2σ^2 ∑(t=1)^n ε_t^2
```
where, ε_t is the residual of the ARMA model.
**Least Squares Estimation:**
```
SSE(φ, θ) = ∑(t=1)^n (X_t - ∑(i=1)^p φ_i X_(t-i) - ∑(j=1)^q θ_j ε_(t-j))^2
```
The parameters of the ARMA model can be estimated by minimizing SSE.
## 2. ARIMA Model Theoretical Foundation
### 2.1 Autoregressive Moving Average Model (ARMA)
#### 2.1.1 Mathematical Expression of ARMA Model
The Autoregressive Moving Average Model (ARMA) is a time series model that represents the time series as a linear combination of past values and error terms. The mathematical expression of the ARMA model is:
```
Y_t = c + ∑(i=1 to p) φ_i * Y_(t-i) + ∑(j=1 to q) θ_j * ε_(t-j) + ε_t
```
where:
- Y_t represents the value of the time series at time t
- c represents the constant term
- p represents the autoregressive order, which is the influence of the past p values on the current value
- q represents the moving average order, which is the influence of the past q error terms on the current value
- φ_i and θ_j represent the autoregressive coefficient and the moving average coefficient, respectively
- ε_t represents the white noise error term, a random variable with mean 0 and variance σ^2
#### 2.1.2 Parameter Estimation of ARMA Model
The parameters of the ARMA model can be estimated using maximum likelihood estimation (MLE) or ordinary least squares (OLS). The MLE method estimates parameters by maximizing the likelihood function, while the OLS method estimates parameters by minimizing the sum of squared residuals.
### 2.2 Autoregressive Integrated Moving Average Model (ARIMA)
#### 2.2.1 Mathematical Expression of ARIMA Model
The Autoregressive Integrated Moving Average Model (ARIMA) is a generalization of the ARMA model, which eliminates non-stationarity by differencing the time series. The mathematical expression of the ARIMA model is:
```
(1 - B)^d * Y_t = c + ∑(i=1 to p) φ_i * (1 - B)^d * Y_(t-i) + ∑(j=1 to q) θ_j * ε_(t-j) + ε_t
```
where:
- B represents the backshift operator, i.e., B * Y_t = Y_(t-1)
- d represents the order of differencing, i.e., (1 - B)^d * Y_t = Y_t - Y_(t-d)
- The other parameters are the same as the ARMA model
#### 2.2.2 Parameter Estimation of ARIMA Model
The parameters of the ARIMA model can be estimated using the same methods as the ARMA model. However, before estimating the parameters, it is necessary to determine the order of differencing d. The order of differencing can be determined by observing the stationarity of the time series.
# 3. ARIMA Model in MATLAB Practice
### 3.1 Identification and Parameter Estimation of ARIMA Model
#### 3.1.1 Using autocorr and pacf Functions to Identify Model
When identifying an ARIMA model, the autocorrelation function (ACF) and partial autocorrelation function (PACF) are typically used to analyze the correlation of time series data.
- **Autocorrelation Function (ACF):** Measures the
0
0
相关推荐
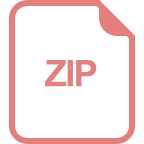