【Advanced】Implementation of Kalman Filter in MATLAB
发布时间: 2024-09-13 23:28:26 阅读量: 60 订阅数: 55 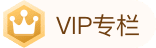
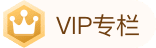
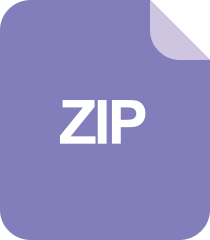
一个基于Qt Creator(qt,C++)实现中国象棋人机对战
# Chapter 1: Theoretical Foundations of the Kalman Filter
The Kalman filter is a recursive algorithm for estimating the state of a dynamic system. It assumes that the system state follows a Markov process and that the measurements are normally distributed. The Kalman filter continuously updates its state estimates through two steps: prediction and update.
In the prediction step, the Kalman filter predicts the current state and its covariance matrix based on the state transition matrix and the process noise covariance matrix. In the update step, the Kalman filter updates the state estimates and their covariance matrix based on the measurements and the measurement noise covariance matrix.
# Chapter 2: Implementation of the Kalman Filter in MATLAB
The Kalman filter algorithm is a recursive estimation method that uses the system state and measurements to estimate the system state. In MATLAB, the Kalman filter can be implemented using the `kalmanfilter` function.
### 2.1 MATLAB Implementation of the Kalman Filter Algorithm
#### 2.1.1 Prediction Step
In the prediction step, the Kalman filter algorithm predicts the current state based on the state estimate from the previous time and the process noise covariance. The implementation code for the prediction step in MATLAB is as follows:
```matlab
% Prediction step
x_pred = A * x_est + B * u;
P_pred = A * P_est * A' + Q;
```
Where:
* `x_pred`: Predicted state estimate
* `x_est`: State estimate from the previous time
* `A`: State transition matrix
* `B`: Control input matrix
* `u`: Control input
* `P_pred`: Predicted state covariance
* `P_est`: State covariance from the previous time
* `Q`: Process noise covariance
#### 2.1.2 Update Step
In the update step, the Kalman filter algorithm updates the current state estimate and its covariance based on the predicted state and measurements. The implementation code for the update step in MATLAB is as follows:
```matlab
% Update step
K = P_pred * C' * inv(C * P_pred * C' + R);
x_est = x_pred + K * (z - C * x_pred);
P_est = (eye(size(P_pred)) - K * C) * P_pred;
```
Where:
* `K`: Kalman gain
* `C`: Measurement matrix
* `z`: Measurement
* `R`: Measurement noise covariance
### 2.2 Selection and Optimization of Kalman Filter Parameters
#### 2.2.1 Determination of State Transition Matrix and Measurement Matrix
The state transition matrix and measurement matrix are two important parameters in the Kalman filter algorithm. The state transition matrix describes the relationship between system states at different times, while the measurement matrix describes the relationship between measurements and system states. The selection and determination of these matrices need to be based on the actual system.
#### 2.2.2 Estimation of Process Noise Covariance and Measurement Noise Covariance
The process noise covariance and measurement noise covariance are two important parameters in the Kalman filter algorithm. The process noise covariance describes the uncertainty of changes in the system state over time, while the measurement noise covariance describes the uncertainty of measurements. The estimation of these covariances needs to be based on the actual system.
# Chapter 3: Applications of the Kalman Filter in MATLAB
### 3.1 Motion Target Tracking
#### 3.1.1 Establishment of Motion Models
Motion target tracking is a classic application scenario of the Kalman filter. In motion target tracking, it is necessary to establish a motion model to describe the motion规律 ***mon motion models include:
- **Constant Velocity Linear Motion Model:** Assumes that the target moves with a constant speed and direction.
- **Constant Acceleration Linear Motion Model:** Assumes that the target moves with a constant acceleration and direction.
- **Constant Acceleration Model:** Assumes that the target's acceleration remains constant at each time step.
#### 3.1.2 Application of the Kalman Filter
After establishing the motion model, the Kalman filter can be used to estimate the target's state (position and velocity). The specific steps of the Kalman filter are as follows:
1. **Prediction Step:** Predict the current state based on the state estimate from the previous time and the process noise covariance.
2. **Update Step:** Update the current state estimate based on the measurements from the current time and the measurement noise covariance.
**Code Block:**
```
% Prediction step
x_pred = x_est + A * u + w;
P_pred = A * P_est * A' + Q;
% Update step
K = P_pred * H' * inv(H * P_pred * H' + R);
x_est = x_pred + K * (z - H * x_pred);
P_est = (eye(n) - K * H) * P_pred;
```
**Code Logic Interpretation:**
- **Prediction Step:**
- `x_pred` represents the predicted state, calculated from the previous state estimate `x_est`, the state transition matrix `A`, the control input `u`, and the process noise `w`.
- `P_pred` represents the predicted covariance, calculated from the previous state covariance `P_est`, the state transition matrix `A`, and the process noise covariance `Q`.
- **Update Step:**
- `K` represents the Kalman gain, calculated from the predicted covariance `P_pred`, the measurement matrix `H`, and the measurement noise covariance `R`.
- `x_est` represents the updated state estimate, calculated
0
0
相关推荐

