[Advanced Chapter] Advanced Numerical Differentiation Techniques: Calculation of Partial Derivatives and Gradients in MATLAB
发布时间: 2024-09-13 23:47:42 阅读量: 10 订阅数: 37 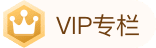
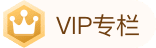
# Advanced Numerical Differentiation Techniques: Partial Derivatives and Gradient Calculations in MATLAB
## 2.1 Forward Difference Method
### 2.1.1 Principle and Formula of the Forward Difference Method
The forward difference method is a numerical differentiation technique used to approximate the derivative of a function at a specific point. It leverages the first-order Taylor series expansion of the function near that point, while ignoring higher-order terms. The specific formula is as follows:
```
f'(x) ≈ (f(x + h) - f(x)) / h
```
Where,
* f(x) is the function value at x
* h is the step size, a small positive number
### 2.1.2 Error Analysis of the Forward Difference Method
The error in the forward difference method is due to the higher-order terms ignored in the Taylor series expansion. The error term is:
```
E = -h^2 * f''(x) / 2
```
Here, f''(x) is the second derivative of the function at x.
The magnitude of the error is proportional to the square of the step size h. Therefore, to reduce the error, a smaller step size should be chosen.
# 2. Numerical Calculation of Partial Derivatives
Partial derivatives are derivatives of multivariate functions with respect to one of the variables. In numerical computation, partial derivatives can be approximated using numerical differentiation methods. This chapter will introduce three commonly used numerical methods for calculating partial derivatives: the forward difference method, central difference method, and composite trapezoidal rule.
### 2.1 Forward Difference Method
#### 2.1.1 Principle and Formula of the Forward Difference Method
The forward difference method is based on a first-order approximation of the Taylor series. It uses the function values at a point and a nearby point to approximate the partial derivative. For the function $f(x, y)$, the forward difference approximation formula for the partial derivative with respect to $x$ at the point $(x_0, y_0)$ is:
```
$$\frac{\partial f}{\partial x}(x_0, y_0) \approx \frac{f(x_0 + h, y_0) - f(x_0, y_0)}{h}$$
```
Where $h$ is a small step size.
#### 2.1.2 Error Analysis of the Forward Difference Method
The error in the forward difference method mainly comes from the truncation error of the Taylor series, with an error order of $O(h)$. Specifically, the error formula for the forward difference method is:
```
$$\frac{\partial f}{\partial x}(x_0, y_0) - \frac{f(x_0 + h, y_0) - f(x_0, y_0)}{h} = \frac{h}{2} \frac{\partial^2 f}{\partial x^2}(\xi, y_0)$$
```
Where $\xi$ is some point between $x_0$ and $x_0 + h$.
### 2.2 Central Difference Method
#### 2.2.1 Principle and Formula of the Central Difference Method
The central difference method is also based on the Taylor series, but it uses the function values at a point and at two nearby points to approximate the partial derivative. For the function $f(x, y)$, the central difference approximation formula for the partial derivative with respect to $x$ at the point $(x_0, y_0)$ is:
```
$$\frac{\partial f}{\partial x}(x_0, y_0) \approx \frac{f(x_0 + h, y_0) - f(x_0 - h, y_0)}{2h}$$
```
#### 2.2.2 Error Analysis of the Central Difference Method
The error in the central difference method comes from the truncation error of the Taylor series, with an error order of $O(h^2)$. Specifically, the error formula for the central difference method is:
```
$$\frac{\partial f}{\partial x}(x_0, y_0) - \frac{f(x_0 + h, y_0) - f(x_0 - h, y_0)}{2h} = -\frac{h^2}{6} \frac{\partial^3 f}{\partial x^3}(\xi, y_0)$$
```
Where $\xi$ is some point between $x_0 - h$ and $x_0 + h$.
### 2.3 Composite Trapezoidal Rule
#### 2.3.1 Principle and Formula of the Composite Trapezoidal Rule
The composite trapezoidal rule is a method that combines the forward difference method and the central difference method. For the function $f(x, y)$, the composite trapezoidal rule approximation formula for the partial derivative with respect to $x$ at the point $(x_0, y_0)$ is:
```
$$\frac{\partial f}{\partial x}(x_0, y_0) \approx \frac{f(x_0 + h, y_0) - f(x_0 - h, y_0)}{2h} + \frac{h^2}{12} \left(\frac{\partial^3 f}{\partial x^3}(x_0 - h, y_0) - \frac{\partial^3 f}{\partial x^3}(x_0 + h, y_0)\right)$$
```
#### 2.3.2 Error Analysis of the Composite Trapezoidal Rule
The error order of the composite trapezoidal rule is $O(h^4)$, and the error formula is:
```
$$\frac{\partial f}{\partial x}(x_0, y_0) - \left(\frac{f(x_0 + h, y_0) - f(x_0 - h, y_0)}{2h} + \frac{h^2}{12} \left(\frac{\partial^3 f}{\partial x^3}(x_0 - h, y_0) - \frac{\partial^3 f}{\partial x^3}(x_0 + h, y_0)\right)\right) = -\frac{h^4}{30} \frac{\partial^5 f}{\partial x^5}(\xi, y_0)$$
```
Where $\xi$ is some point between $x_0 - h$ and $x_0 + h$.
# 3.1 Definition and Properties of the Gradient
**Definition**
The gradient is a vector, each component of which represents the ra
0
0
相关推荐
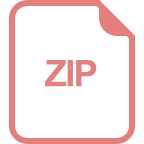
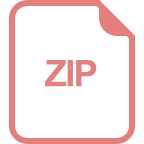





