【Advanced Section】High-Dimensional Data Analysis: Multidimensional Scaling (MDS) in MATLAB
发布时间: 2024-09-13 23:57:26 阅读量: 10 订阅数: 34 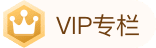
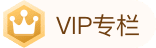
# 2.1 The Principle and Algorithm of MDS
### 2.1.1 Calculation of Distance Matrix
The fundamental idea behind MDS is to project high-dimensional data onto a low-dimensional space, ensuring that the distances between data points after projection are as similar as possible to those in the original high-dimensional data. To achieve this, MDS first needs to compute the distance matrix between the original high-dimensional data points.
The distance matrix is an n×n matrix, ***monly used distance metrics include Euclidean distance, Manhattan distance, and cosine distance.
### 2.1.2 Implementation of Dimensionality Reduction Projection
After calculating the distance matrix, MD***mon dimensionality reduction projection algorithms include Classical Multidimensional Scaling (CMDS) and Non-metric Multidimensional Scaling (NMDS).
The CMDS algorithm is based on Euclidean distance. It seeks the optimal low-dimensional projection by minimizing the sum of the Euclidean distances between projected data points. The NMDS algorithm, on the other hand, is based on any distance metric. It looks for the optimal low-dimensional projection by minimizing the difference between the distances between the projected data points and the distances in the original distance matrix.
# 2. Theoretical Basis of Multidimensional Scaling (MDS)
### 2.1 The Principle and Algorithm of MDS
#### 2.1.1 Calculation of Distance Matrix
The fundamental principle of MDS is to project high-dimensional data onto a low-dimensional space while maintaining the distance relationships within the original data as much as possible. Specifically, ***monly used distance metrics include Euclidean distance, Manhattan distance, cosine distance, etc.
```python
# Calculate Euclidean distance matrix
import numpy as np
from scipy.spatial.distance import pdist, squareform
data = np.array([[1, 2], [3, 4], [5, 6]])
distance_matrix = pdist(data, 'euclidean')
distance_matrix = squareform(distance_matrix)
# Print distance matrix
print(distance_matrix)
```
**Parameter Description:**
* `pdist`: Function to calculate the distance matrix, `'euclidean'` indicates the use of Euclidean distance.
* `squareform`: Converts the distance matrix into a square matrix.
**Code Logic:**
1. Use the `pdist` function to calculate the distance matrix, resulting in a one-dimensional array.
2. Use the `squareform` function to convert the distance matrix into a square matrix for easier processing later.
#### 2.1.2 Implementation of Dimensionality Reduction Projection
After calculating the distance matrix, ***mon dimensionality reduction algorithms include classic MDS, Principal Component Analysis (PCA), and Singular Value Decomposition (SVD).
```python
# Use classic MDS for dimensionality reduction
from sklearn.manifold import MDS
mds = MDS(n_components=2)
low_dim_data = mds.fit_transform(distance_matrix)
# Print the data after dimensionality reduction
print(low_dim_data)
```
**Parameter Description:**
* `n_components`: Target dimension for dimensionality reduction, here is 2.
**Code Logic:**
1. Create an MDS object using the `MDS` class and set the target dimension for dimensionality reduction to 2.
2. Use the `fit_transform` method to perform dimensionality reduction on the distance matrix, obtaining the reduced data.
### 2.2 Advantages and Disadvantages of MDS and Applicable Scenarios
#### 2.2.1 Advantages and Limitations of MDS
The advantage of MDS is that it can maintain the distance relationships in the original data and can handle nonlinear data. However, MDS also has some limitations, such as:
***High computational complexity:** The computational complexity of MDS increases exponentially with the increase in data dimensions.
***Local optimal solution:** The MDS algorithm may fall into a local optimal solution, resulting in an unsatisfactory projection result.
***Sensitivity to outliers:** MDS is sensitive to outliers, which may affect the accuracy of the projection result.
#### 2.2.2 Applicable Data Types and Problems for MDS
MDS is suitable for processing the following types of data:
***High-dimensional data:** MDS is mainly used for high-dimensional data, such as text data, image data, etc.
***Nonlinear data:** MDS can handle nonlinear data, such as data with complex relationships.
***Clear distance information:** MDS requires clear distance information, so it is suitable for data where distance metrics are well-defined.
MDS is commonly used to solve the following problems:
***Data visualizat
0
0
相关推荐
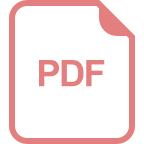
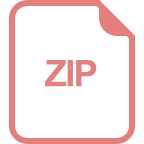
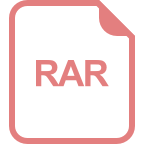





