【Advanced Level】Reinforcement Learning Algorithms: Q-Learning and Policy Gradient Methods in MATLAB
发布时间: 2024-09-14 00:05:25 阅读量: 29 订阅数: 38 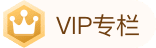
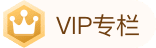
# [Advanced Series] Reinforcement Learning Algorithms: Q-Learning and Policy Gradient Methods in MATLAB
## 1. Basics of Reinforcement Learning**
Reinforcement learning is a paradigm of machine learning that enables agents to learn optimal behavior through interactions with their environment. Unlike supervised learning, reinforcement learning does not require labeled data but instead guides the agent's learning through rewards and penalties.
The core concept of reinforcement learning is the Markov decision process (MDP), which consists of the following elements:
***State (S):** The current state of the agent in the environment.
***Action (A):** The set of actions the agent can take.
***Reward (R):** The reward or penalty the agent receives after performing an action.
***State Transition Probability (P):** The probability of transitioning from one state to another after performing an action.
***Discount Factor (γ):** A factor used to balance immediate rewards with future rewards.
## 2. Q-Learning Algorithm**
### 2.1 Principles and Formulas of Q-Learning
Q-learning is a model-free reinforcement learning algorithm that guides an agent's behavior by learning the state-action value function (Q function). The Q function represents the expected long-term reward for taking a particular action in a given state.
The Q-learning update formula is as follows:
```python
Q(s, a) <- Q(s, a) + α * (r + γ * max_a' Q(s', a') - Q(s, a))
```
Where:
* `s`: Current state
* `a`: Current action
* `r`: Current reward
* `s'`: Next state
* `a'`: Next action
* `α`: Learning rate
* `γ`: Discount factor
### 2.2 Process and Steps of the Q-Learning Algorithm
The process of the Q-learning algorithm is as follows:
1. Initialize the Q function
2. Observe the current state `s`
3. Choose action `a` based on the current Q function
4. Execute action `a` and receive reward `r` and the next state `s'`
5. Update the Q function
6. Repeat steps 2-5 until the termination condition is met
### 2.3 MATLAB Implementation of the Q-Learning Algorithm
The implementation of the Q-learning algorithm in MATLAB is as follows:
```matlab
% Initialize the Q function
Q = zeros(num_states, num_actions);
% Set the learning rate and discount factor
alpha = 0.1;
gamma = 0.9;
% Training loop
for episode = 1:num_episodes
% Initialize state
s = start_state;
% Loop until reaching the terminal state
while ~is_terminal(s)
% Choose action based on the Q function
a = choose_action(s, Q);
% Execute action and receive reward and next state
[s_prime, r] = take_action(s, a);
% Update the Q function
Q(s, a) = Q(s, a) + alpha * (r + gamma * max(Q(s_prime, :)) - Q(s, a));
% Update state
s = s_prime;
end
end
```
*Code Logic Analysis:*
* The `choose_action` function selects an action based on the current Q function.
* The `take_action` function executes the action and receives the reward and next state.
* The `is_terminal` function checks if a state is a terminal state.
* `num_states` and `num_actions` represent the size of the state space and action space respectively.
* The training loop updates the Q function through multiple iterations until the termination condition is met.
## 3. Policy Gradient Methods
### 3.1 Derivation of the Policy Gradient Theorem
**Policy Gradient Theorem** is the foundation of policy gradient methods; it provides a formula for computing policy gradients, which are the gradients of changes in policy parameters with respect to the objective function. The derivation process of the policy gradient theorem is as follows:
**Objective Function:** The objective function in reinforcement learning is typically represented as the expected return:
```
J(θ) = E[R(θ)]
```
Where:
* θ is the policy parameters
* R(θ) is the return under policy θ
**Policy Gradient:** The policy gradient is defined as the gradient of the objective function J(θ) with respect to the policy parameters θ:
```
∇θJ(θ) = E[∇θR(θ)]
```
*Derivation Process:*
1. **Expectation Decomposition:** The expected value E[∇θR(θ)] can be decomposed into the sum of the expected values over all possible states and actions:
```
E[∇θR(θ)] = ∫∇θR(θ) p(s, a | θ) ds da
```
Where:
* p(s, a | θ) is the joint probability of state s and action a under policy θ
2. **Rewrite Joint Probability:** The joint probability p(s, a | θ) can be rewritten as the product of state probability p(s | θ) and action probability p(a | s, θ):
```
p(s, a | θ) = p(s | θ) p(a | s, θ)
```
3. **Substitute Gradient Formula:** Substitute the rewritten joint probability into the policy gradient formula:
```
∇θJ(θ) = ∫∇θR(θ) p(s | θ) p(a | s, θ) ds da
```
4. **Exchange Integral and Gradient:** Since the gradient operator is a linear operator, the integral and gradient can be exchanged:
```
∇θJ(θ) = ∫p(s | θ) ∇θ[R(θ) p(a | s, θ)] ds da
```
5. **Simplify Gradient:** Since R(θ) does not depend on the action a, its gradient is 0. Therefore, the gradient formula can be simplified to:
```
∇θJ(θ) = ∫p(s | θ) ∇θ[p(a | s, θ)] R(θ) ds da
```
*Conclusion:* This is the formula of the policy gradient theorem, which provides a method for computing policy gradients, i.e., the gradient of changes in policy parameters with respect to the objective function.
### 3.2 Variants of Policy Gradient Methods
There are various variants of policy gradient methods, each with its own advantages and disadvantages. Some common variants include:
**REINFORCE Algorithm:** The REINFORCE algorithm is the basic form of policy gradient methods; it directly uses the policy gradient th
0
0
相关推荐
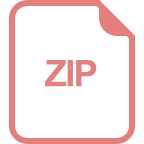
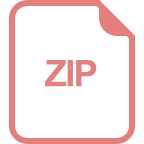
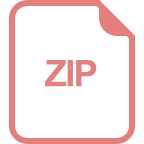
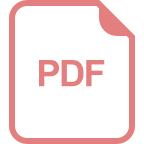
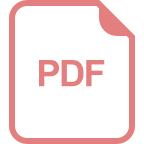
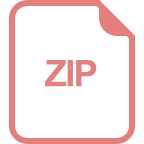
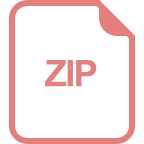
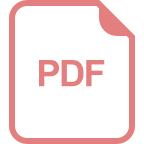
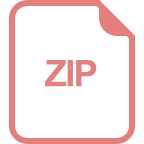