【Advanced】Using MATLAB to Implement Long Short-Term Memory (LSTM) Networks for Classification and Regression Problems
发布时间: 2024-09-13 23:12:31 阅读量: 29 订阅数: 38 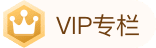
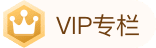
# 2.1 LSTM Network Architecture and Algorithm
### 2.1.1 Composition and Principle of LSTM Units
Long Short-Term Memory (LSTM) is a type of Recurrent Neural Network (RNN) designed specifically for handling sequential data. An LSTM unit consists of an input gate, a forget gate, an output gate, and a cell state.
***Input Gate:** Determines which new information will be added to the cell state.
***Forget Gate:** Determines which existing information will be removed from the cell state.
***Output Gate:** Determines which information will be output from the cell state.
***Cell State:** Stores the memory of the network within the sequence.
The LSTM unit controls the flow of information through these gates, allowing it to learn long-term dependencies that traditional RNNs are unable to do.
### 2.1.2 Structure and Training Process of LSTM Networks
LSTM networks are typically composed of multiple stacked LSTM units, with each unit processing one time step in the sequence. The network's output is generated by the output gate of the last LSTM unit.
Training an LSTM network involves optimizing the network weights to minimize the loss function, which measures the difference between the network output and the target output. The training process employs the backpropagation algorithm, which computes gradients for the weights and updates them to reduce the loss.
# 2. LSTM Implementation in MATLAB
### 2.1 LSTM Network Architecture and Algorithm
#### 2.1.1 Composition and Principle of LSTM Units
Long Short-Term Memory (LSTM) is a type of Recurrent Neural Network (RNN) specifically designed to process time series data. The LSTM unit is the fundamental component of an LSTM network, capable of remembering long-term dependencies, a feat that standard RNNs cannot achieve.
The LSTM unit is composed of four main parts:
- **Forget Gate:** Decides which information from the previous time step to forget.
- **Input Gate:** Decides which new information from the current time step to store in the cell state.
- **Cell State:** Stores long-term dependencies.
- **Output Gate:** Decides which information from the cell state to output at the current time step.
The mathematical formulas for the LSTM unit are as follows:
```
f_t = σ(W_f * [h_{t-1}, x_t] + b_f) # Forget Gate
i_t = σ(W_i * [h_{t-1}, x_t] + b_i) # Input Gate
o_t = σ(W_o * [h_{t-1}, x_t] + b_o) # Output Gate
c_t = f_t * c_{t-1} + i_t * tanh(W_c * [h_{t-1}, x_t] + b_c) # Cell State
h_t = o_t * tanh(c_t) # Output
```
Where:
- σ is the sigmoid function
- W and b are weight and bias parameters
- h is the hidden state
- x is the input data
- c is the cell state
#### 2.1.2 Structure and Training Process of LSTM Networks
An LSTM network is composed of multiple stacked LSTM units. Each unit processes data from one time step and outputs it to the next unit. The structure of an LSTM network typically consists of the following layers:
- **Input Layer:** Receives the input data.
- **LSTM Layer:** Consists of multiple LSTM units that process sequential data.
- **Output Layer:** Produces the final output.
The training process of an LSTM network is similar to that of other neural networks. It involves the following steps:
1. **Forward Propagation:** Passes the input data through the network and computes the loss function.
2. **Backward Propagation:** Computes the gradient of the loss function with respect to the network weights and biases.
3. **Weight Update:** Updates the network weights and biases using gradient descent or other optimization algorithms.
### 2.2 Creation and Training of LSTM in MATLAB
#### 2.2.1 Creation and Configuration of LSTM Layers
In MATLAB, the `deeplearning` toolbox can be used to create and configure LSTM layers. The `lstmLayer` function is used to create an LSTM layer, with the syntax as follows:
```
layer = lstmLayer(hiddenSize, 'OutputMode', 'sequence')
```
Where:
- `hiddenSize` is the size of the hidden state of the LSTM unit.
- `OutputMode` specifies the output mode of the LSTM layer. `sequence` indicates that the output is a time series, while `last` indicates that the output is the hidden state of the last time step.
#### 2.2.2 Data Preprocessing and Model Training
Before training an LSTM model, it is necessary to preprocess the training data. This typically includes:
- **Data Normalization:** Scaling data to the range of [0, 1] or [-1, 1].
- **Sequence Truncation:** Truncating time series to a fixed length.
- **Sequence Padding:** Padding shorter sequences with filler values.
Training an LSTM model involves the following steps:
1. **Create Data Store:** Use the `datastore` function to create training and validation data stores.
2. **Create Network:** Use the `sequenceInputLayer`, `lstmLayer`, and `classificationLayer` functions to create an LSTM network.
3. **Training Options:** Specify training options, such as learning rate, number of training epochs, and validation frequency.
4. **Train Network:** Use the `trainNetwork` function to train the LSTM network.
### 2.3 Evaluation and Optimization of LSTM Models
#### 2.3.1 Model Evaluation Metrics and Methods
The evaluation metrics for LSTM models depend on the task at hand. For classification problems, common metrics include:
- **Accuracy:** The ratio of the number of correctly predicted samples to the total number of samples.
- **Recall:** The ratio of the number of positive ins
0
0
相关推荐
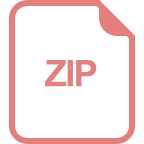
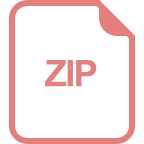
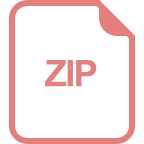





