Feature Engineering for Time Series Forecasting: Experts Guide You in Building Forecasting Gold Standards
发布时间: 2024-09-15 06:30:46 阅读量: 37 订阅数: 42 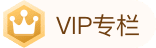
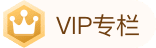
## Chapter 1: Fundamental Theories of Time Series Forecasting
In this chapter, we will delve into the core concepts and theoretical foundations of time series forecasting. Time series forecasting is a process that uses historical data and specific mathematical models to predict data at a certain point in the future or within a period of time. Time series data typically refers to observations ordered in time, such as stock prices, temperature changes, sales records, and more.
Time series analysis focuses on four basic components:
1. Trend: The long-term upward or downward movement.
2. Seasonality: Patterns that repeat at fixed intervals.
3. Cyclical: Fluctuations with non-fixed periods, similar to economic cycles.
4. Irregular: Unpredictable random fluctuations.
Understanding these components is crucial for constructing accurate time series forecasting models. The following chapters will interpret each part in detail and discuss how they are closely integrated with feature engineering, data preprocessing, and model construction and evaluation in practical applications.
## Chapter 2: The Importance of Feature Engineering in Time Series Forecasting
### 2.1 The Relationship Between Feature Engineering and Time Series Forecasting
#### Challenges in Time Series Forecasting
As a branch of data analysis, time series forecasting plays a significant role in business and scientific research. For instance, in economic analysis, stock price prediction, weather forecasting, and supply chain management, time series forecasting is an essential tool for predicting future trends and making decisions. However, time series data is typically noisy, non-stationary, and seasonal, posing many challenges for forecasting.
#### The Role of Feature Engineering
Feature engineering is a critical step in addressing such issues. It involves extracting information from raw data to construct features that assist in the prediction task. In time series forecasting, feature engineering encompasses preprocessing of raw data and includes feature selection, construction, and transformation, aiming to improve model performance. Feature engineering can enhance the signal that the model learns, reduce noise and dimensionality, and thus increase the model's predictive accuracy and generalization ability.
#### The Importance of Feature Engineering
Good features can capture potential regularities and trends in data, allowing the model to better understand and predict the future. An effective feature engineering process can significantly improve model performance, sometimes even more so than model selection and hyperparameter tuning. Through feature engineering, we can extract time dependencies, periodicity, and other characteristics from time series data, providing more useful information for the model.
#### Challenges in Feature Engineering
Feature engineering is an iterative process that requires domain knowledge. It necessitates that analysts have a deep understanding of the dataset, the actual meanings behind the data, and the business logic. Additionally, feature engineering for time series data often involves complex temporal dependencies that may require sophisticated statistical methods and machine learning algorithms to capture. Feature selection and construction not only require professional knowledge but also a significant amount of experimentation and validation to determine.
### 2.2 The Application of Feature Engineering in Different Scenarios
#### Scenario 1: Financial Industry
In the financial industry, time series forecasting is commonly used for stock price prediction and the development of trading strategies. Here, feature engineering might include calculating historical price information, trading volume, moving averages, etc. By analyzing patterns and signals in historical data, feature engineering can help predict future stock trends.
#### Scenario 2: Manufacturing
In manufacturing, time series forecasting can be used to predict equipment failures and maintenance needs. Feature engineering might involve analyzing sensor data such as temperature, pressure, and sound from equipment operation. By analyzing these sensor data, we can extract features indicating the health of the equipment, which can then be used to predict potential failures.
#### Scenario 3: Retail
In retail, time series forecasting is typically used for sales forecasting and inventory management. Feature engineering might include factors such as seasonality, promotional activities, holidays, and other factors that affect sales. By extracting and analyzing these features, retailers can more accurately predict future sales figures, thus effectively managing inventory and formulating sales strategies.
### 2.3 Best Practices in Feature Engineering
#### Best Practices in Feature Selection
Feature selection aims to choose the most helpful subset from a large number of features. This can be achieved through statistical tests, model-based feature importance evaluations, and correlation-based filtering. For example, models like Random Forests or Gradient Boosting Trees can be used to assess feature importance. In addition, considering the correlation between different features and avoiding multicollinearity is an important consideration in feature selection.
#### Best Practices in Feature Construction
Feature construction involves creating new features through mathematical transformations or combining existing ones. This can be accomplished by defining transformations based on domain knowledge, aggregation functions, or interaction terms. For example, in time series, data within a certain time window can be aggregated into averages or sums to create new features; or by considering lag values in the time series to capture dynamic features. When constructing features, it is essential to consider the actual meaning of the data and business logic to ensure that new features are meaningful.
#### Best Practices in Feature Transformation
Feature transformation is used to improve the distribution of features or make them more consistent with the model'***mon transformation methods include standardization, normalization, logarithmic transformation, Box-Cox transformation, etc. For example, in many machine learning models, the scale of features can significantly affect the model's performance. Therefore, standardizing or normalizing features to a certain range can improve the model's convergence speed and prediction accuracy. Furthermore, for positively skewed data, logarithmic transformation can help reduce the impact of extreme values, making the data more normally distributed.
#### Best Practices in Feature Reduction
Feature reduction is the process of reducing the number of features, which helps prevent overfitting, increase the model's interpretability, and reduce the consumption of computational resources. Dimensionality reduction can be achieved through algorithms such as Principal Component Analysis (PCA) and Linear Discriminant Analysis (LDA). In time series forecasting, PCA can identify the most important components of the data, which are summaries of linear combinations of the original features and capture the main variations in the data. Dimensionality reduction techniques are particularly useful in dealing with high-dimensional time series data, significantly enhancing model performance.
### 2.4 The Impact of Feature Engineering on Model Performance
#### Positive Impacts of Feature Engineering on Model Performance
Op
0
0
相关推荐
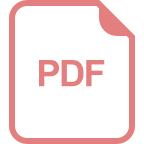
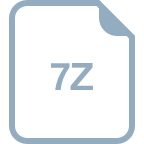
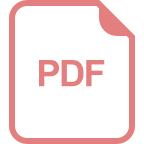





