Cluster Analysis Evaluation: Silhouette Coefficient and Other Internal Metrics
发布时间: 2024-09-15 14:26:23 阅读量: 28 订阅数: 30 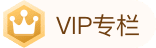
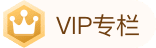
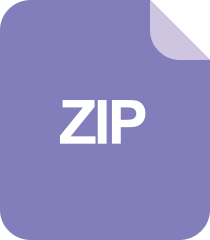
DA-proj3-ventures-cluster-analysis:JHU Decision Analytics课程的小型项目#3
# Cluster Analysis Evaluation: Silhouette Coefficient and Other Internal Metrics
## 1. Overview of Cluster Analysis
### 1.1 Definition and Importance of Cluster Analysis
Cluster Analysis is a vital technique in data mining that aims to divide the samples in a dataset into several clusters based on a similarity measure. These clusters should have high internal similarity and low similarity between each other. Cluster Analysis helps us uncover hidden structures in data and is widely applied in various fields such as market segmentation, social network analysis, organizational biology data, and astronomical data analysis. Due to its unsupervised nature, cluster analysis is particularly valuable when dealing with unlabelled data.
### 1.2 Applications of Cluster Analysis
In practical applications, cluster analysis can be used not only for data preprocessing but also as part of feature extraction, or to aid in data visualization. Additionally, it is often used in pattern recognition, image segmentation, search engines, recommendation systems, and more. It is an indispensable tool in data science. Through clustering, we can conduct preliminary exploration and understanding of the data, laying the groundwork for further data analysis.
### 1.3 Types of Clustering Algorithms and Their Selection
There are various types of clustering algorithms, including partitioning methods (like K-means), hierarchical methods (like AGNES), density-based methods (like DBSCAN), grid-based methods (like STING), and model-based methods (like GMM). Selecting an appropriate clustering algorithm requires consideration of data characteristics such as sample size, feature dimensionality, cluster shape, and distribution. Understanding the principles, advantages, and disadvantages of different clustering algorithms is crucial for obtaining high-quality clustering results.
# 2. Internal Evaluation Metrics for Clustering Algorithms
Internal evaluation metrics for clustering algorithms are used to assess the quality of clustering results. These metrics typically do not rely on external information but evaluate based on the characteristics of the dataset itself. By using these metrics, we can understand the performance of clustering algorithms and make adjustments accordingly. This chapter will focus on the silhouette coefficient and other common internal evaluation metrics.
## 2.1 Principles and Calculation of the Silhouette Coefficient
### 2.1.1 Definition and Significance of the Silhouette Coefficient
The silhouette coefficient is a value between -1 and 1, used to measure the quality of clustering for individual samples. The silhouette coefficient takes into account both the similarity (cohesion) of a sample to other samples within the same cluster and the dissimilarity (separation) to the samples of the nearest cluster.
- **Cohesion** describes the average similarity of a sample to other samples in its own cluster. The higher the cohesion, the more similar the sample is to other samples in the cluster.
- **Separation** describes the average dissimilarity of a sample to the samples of the nearest cluster. The lower the separation, the more dissimilar the sample is to the samples of the nearest cluster.
The formula for calculating the silhouette coefficient is:
\[ s(i) = \frac{b(i) - a(i)}{\max\{a(i), b(i)\}} \]
where, \( s(i) \) is the silhouette coefficient for the \( i \)-th sample, \( a(i) \) is the average distance from sample \( i \) to all other samples in its own cluster (cohesion), and \( b(i) \) is the average distance from sample \( i \) to all samples in the nearest non-self cluster (separation).
### 2.1.2 Method for Calculating the Silhouette Coefficient
Calculating the silhouette coefficient involves the following steps:
1. **Calculate the cohesion \( a(i) \)** for each sample: compute the average distance from each sample to all other samples within the same cluster.
2. **Calculate the separation \( b(i) \)** for each sample: find the average distance from each sample to all samples in the nearest cluster that is not its own.
3. **Calculate the silhouette coefficient \( s(i) \)** using the formula provided.
4. **Summarize all sample silhouette coefficients**: calculate the average silhouette coefficient of all samples to obtain the dataset's overall silhouette coefficient.
To demonstrate specifically, we can use Python's scikit-learn library to calculate the silhouette coefficient:
```python
from sklearn.metrics import silhouette_score
from sklearn.cluster import KMeans
# Assuming we have a dataset X and the number of clusters k
X = ... # dataset
k = 3 # assuming the number of clusters is 3
# Using KMeans algorithm for clustering
kmeans = KMeans(n_clusters=k, random_state=42)
clusters = kmeans.fit_predict(X)
# Calculate the silhouette coefficient
score = silhouette_score(X, clusters)
print(f"Silhouette Coefficient: {score}")
```
In this code, `X` is the dataset, and `k` is the number of clusters we specify. We perform clustering using the KMeans algorithm and calculate the silhouette coefficient for the entire dataset using the `silhouette_score` function.
## 2.2 Other Internal Evaluation Metrics
### 2.2.1 Homogeneity, Completeness, and V-measure
Homogeneity, completeness, and V-measure are metrics used to assess the similarity between clustering results and given true labels.
- **Homogeneity** measures whether each cluster contains only members of a single class.
- **Completeness** measures whether all members of the same class are assigned to the same cluster.
- **V-measure** is the harmonic mean of homogeneity and completeness. A higher value indicates that the clustering result is more consistent with the true labels.
### 2.2.2 Mutual Information and Adjusted Mutual Information
Mutual information (MI) and adjusted mutual information (AMI) are information-theoretic metrics that evaluate the amount of shared information between clustering results and true labels.
- **Mutual information**: assesses clustering quality by calculating the mutual information between clustering results and true labels.
- **Adjusted mutual information**: adjusts MI by considering the randomness of clustering, making it more suitable for comparing results from different clustering methods.
### 2.2.3 Metrics for Estimating Cluster Number: Davies-Bouldin Index and Dunn Index
- **Davies-Bouldin index**: evaluates clustering quality by comparing the ratio of within-cluster distances to between-cluster distances. Generally, the Davies-Bouldin index decreases first and then increases as the number of clusters grows.
- **Dunn index**: defined as the ratio of the farthest distance between clusters to the closest distance within clusters. A higher Dunn index indicates tighter clusters and greater separation between clusters.
By analyzing these metrics, we can better understand the performance of different clustering algorithms and select the most
0
0
相关推荐
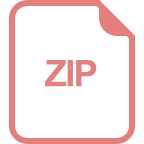
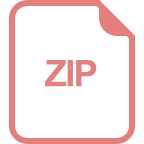
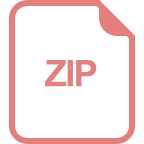
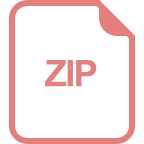
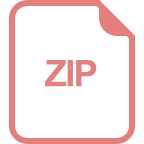
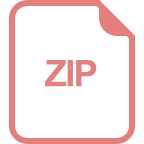


