Visualizing Model Performance: Plotting ROC Curves and AUC Values
发布时间: 2024-09-15 14:04:34 阅读量: 40 订阅数: 38 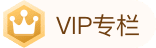
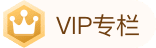
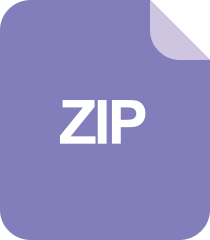
visualizing-git:可视化常见的Git操作如何影响提交图
# Visualizing Model Performance: Plotting ROC Curves and Calculating AUC Values
## 1. The Importance of Model Performance Evaluation
In the process of building machine learning models, evaluating model performance is an indispensable step. Proper performance evaluation helps us understand the model's generalization capability for new data, determine whether the model is overfitting or underfitting, and ultimately choose the most appropriate model. Especially in classification problems, accurately measuring a model's predictive power has become a challenge that data scientists and machine learning engineers must face.
The choice of performance evaluation metrics is crucial for the results of model evaluation. We usually use accuracy, precision, recall, F1 score, and other metrics to evaluate a classification model. These metrics can reflect the performance of the model from different angles, but in some cases, a single metric cannot comprehensively reflect the predictive performance of the model, especially when the class distribution in the dataset is uneven, which limits the application of single metrics. Therefore, ROC curves and AUC values, as comprehensive indicators for measuring the performance of binary classification models, are widely used because they can provide a more comprehensive evaluation perspective.
In this chapter, we will delve into the importance of model performance evaluation, explain why ROC curves and AUC values become indispensable tools in different situations, and their advantages and limitations in different application scenarios. Through in-depth analysis, readers will gain a more comprehensive understanding of model performance evaluation and be able to choose appropriate evaluation methods for different problems.
## 2. The Basic Theory of ROC Curves and AUC Values
ROC curves and AUC values are common tools for evaluating the performance of classification models, especially in binary classification problems with imbalanced datasets. To deeply understand these two concepts, this chapter will start from the basic theory, explain the principles of drawing ROC curves, the statistical significance of AUC values, and their applications in model performance evaluation.
### 2.1 Performance Evaluation Metrics for Binary Classification Problems
In classification problems, the main task of the model is to correctly classify the samples in the dataset into two categories. For binary classification problems, we usually focus on the following performance evaluation metrics.
#### 2.1.1 True Positive Rate and False Positive Rate
The True Positive Rate (TPR) and False Positive Rate (FPR) are basic performance evaluation metrics. They are defined as follows:
- True Positive Rate (TPR): The proportion of correctly predicted positive samples in all positive class samples.
- False Positive Rate (FPR): The proportion of incorrectly predicted positive samples in all negative class samples.
True Positive Rate and False Positive Rate can directly reflect the model's performance in distinguishing between positive and negative classes. The values of these two indicators range from 0 to 1, and the closer to 1, the better the model performs in the corresponding aspect.
#### 2.1.2 Definition and Drawing Principles of ROC Curves
The ROC curve is drawn on the coordinate system of TPR and FPR according to different classification thresholds. Each point represents the TPR and FPR values under a possible classification threshold setting. The specific drawing steps are as follows:
1. Calculate TPR and FPR for each classification threshold;
2. Use FPR as the horizontal coordinate and TPR as the vertical coordinate to plot the corresponding points;
3. Connect these points to form the ROC curve.
The closer the ROC curve is to the upper left corner of the coordinate axis, the better the model performance. The ideal model's ROC curve will present as an abruptly ascending broken line, passing through the point (0, 1).
### 2.2 The Meaning and Calculation Method of AUC Values
The AUC value (Area Under the Curve) is the area under the ROC curve, and its value can measure the average performance of the model under all classification thresholds.
#### 2.2.1 Definition and Statistical Significance of AUC Values
The AUC value represents the probability that a model will rank a positive sample higher than a negative sample when randomly selecting a positive sample and a negative sample. The range of AUC values is [0.5, 1]. When the AUC value is 0.5, it indicates that the model is guessing randomly; when the AUC value is 1, it indicates that the model is perfectly classified.
#### 2.2.2 The Calculation Process of AUC Values
There are various methods to calculate AUC values, such as the trapezoidal rule and interpolation methods. This chapter will introduce the process of calculating AUC values using the trapezoidal rule:
1. Divide the area under the ROC curve into several trapezoids;
2. Calculate the area of each trapezoid and sum them up;
3. The sum of the accumulated areas is the AUC value.
Specifically, in mathematical formula representation, if we take TPR and FPR as the two sides, the area under the ROC curve can be seen as composed of these trapezoids, and then the area of each trapezoid is accumulated to obtain the AUC value.
## 2.3 The Advantages and Disadvantages of ROC Curves and AUC Values
As evaluation metrics, ROC curves and AUC values have a wide range of applications, but they also have some limitations.
### 2.3.1 Comparison with Other Evaluation Metrics
Compared to other evaluation metrics such as accuracy, ROC curves and AUC values perform more stably in imbalanced datasets and can more comprehensively reflect model performance. However, compared to precision and recall, ROC and AUC may not be the ideal choice in certain specific application scenarios, such as situations that require high recall rates.
### 2.3.2 Limitations of ROC Curves and AUC Values
Although ROC curves and AUC values are powerful tools, they still face limitations:
- For multi-class classification problems, ROC curves and AUC values are not directly applicable;
- In some datasets, especially when the sample size is very small, the curve and AUC values may not be stable enough;
- In some cases, the model's predictions may overly rely on data from a particular category.
Understanding these advantages and disadvantages helps us use ROC curves and AUC values more reasonably for model performance evaluation.
In the following chapters, we will delve into how to use Python tools to plot ROC curves and calculate AUC values, and we will also explore the application of these two indicators in different types of problems and imbalanced datasets. This chapter is only the theoretical part, providing a solid theoretical foundation for in-depth application.
# 3. Using Python to Plot ROC Curves and Calculate AUC Values
## 3.1 From Theory to Practice: Preparing Data and Models
### 3.1.1 Data Preprocessing
Before model training, data preprocessing is crucial. Data preprocessing may include data cleaning, missing value handling, data standard
0
0
相关推荐







