Evaluation Methods for Unsupervised Learning: Assessing the Performance of Clustering Algorithms
发布时间: 2024-09-15 14:31:15 阅读量: 24 订阅数: 23 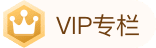
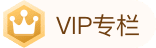
# 1. An Introduction to Unsupervised Learning and Clustering Algorithms
Clustering analysis is an important unsupervised learning method in the fields of data mining and machine learning. It aims to group the samples in a dataset into multiple categories based on their similarities. Unlike supervised learning, unsupervised learning does not require pre-labeled training data to guide the learning process. Clustering algorithms provide solutions for dealing with large amounts of unlabeled data and are widely applied in various fields such as customer segmentation, market analysis, social network analysis, and bioinformatics.
The fundamental idea of clustering algorithms is to assign sample points into categories with high similarity among themselves and low similarity with points in other categories. Depending on different distance measurement criteria, such as Euclidean distance, Manhattan distance, or cosine similarity, sample points are grouped. Clustering methods can be divided into hierarchical clustering, partition-based clustering, density-based clustering, grid-based clustering, and more.
Since clustering is an unguided process, there is no uniform "correct answer." Different clustering algorithms may produce different results, and how to evaluate the effectiveness of clustering results has always been a challenge. Therefore, a deep understanding and mastery of clustering algorithm evaluation methods is crucial for optimizing clustering models and improving the accuracy and reliability of clustering results.
# 2. The Performance Evaluation Theory of Clustering Algorithms
In exploring the world of clustering algorithms, we inevitably need tools to measure our work. This is why performance evaluation plays an indispensable role in the development of clustering algorithms. This chapter will delve into the theory of performance evaluation for clustering algorithms, analyzing how to assess the quality of clustering results and how to judge the stability of clustering algorithms.
## 2.1 Performance Evaluation Metrics for Clustering Algorithms
When we talk about performance evaluation, we inevitably start with evaluation metrics. The performance evaluation metrics for clustering algorithms can be broadly divided into three categories: internal metrics, external metrics, and relative metrics. These metrics provide means to evaluate clustering results from different perspectives.
### 2.1.1 Internal Metrics: Silhouette Coefficient and Davies-Bouldin Index
Internal metrics refer to evaluating the quality of clustering results using only the information from the data itself. Here, we will discuss in detail two commonly used internal metrics: the silhouette coefficient and the Davies-Bouldin index.
#### Silhouette Coefficient
The silhouette coefficient is a metric used to evaluate the goodness of clustering results, with values ranging from -1 to 1. A silhouette coefficient close to 1 indicates very good clustering effects, while a value close to -1 indicates very poor clustering effects. The formula for calculating the silhouette coefficient is:
\[ s = \frac{1}{n} \sum_{i=1}^{n} \frac{b(i) - a(i)}{\max \{a(i), b(i)\}} \]
Here, \( a(i) \) is the average distance from sample \( i \) to other samples in the same cluster, and \( b(i) \) is the average distance from sample \( i \) to all samples in the nearest cluster. The silhouette coefficient considers both the compactness and separation of the cluster.
```python
from sklearn.metrics import silhouette_score
from sklearn.cluster import KMeans
import numpy as np
# Assuming 'data' is the dataset we use for clustering
# Using KMeans for clustering
kmeans = KMeans(n_clusters=3, random_state=42)
clusters = kmeans.fit_predict(data)
# Calculating the silhouette coefficient
silhouette_avg = silhouette_score(data, clusters)
print(f"The average silhouette_score is : {silhouette_avg}")
```
#### Davies-Bouldin Index
The Davies-Bouldin index (DB Index) is another widely used internal metric that is based on the ratio of the dispersion between classes and the compactness within classes. The smaller the value of this index, the better the clustering results. The calculation is as follows:
\[ DB = \frac{1}{n} \sum_{i=1}^{n} \max_{j \neq i} \left( \frac{\sigma_i + \sigma_j}{d(c_i, c_j)} \right) \]
Where, \( \sigma_i \) is the average distance from the samples in cluster \( i \) to the cluster center, and \( d(c_i, c_j) \) is the distance between the centers of two clusters.
Next, we will show how to use the Davies-Bouldin index in Python:
```python
from sklearn.metrics import davies_bouldin_score
from sklearn.cluster import KMeans
# Assuming 'data' is the dataset we use for clustering
# Using KMeans for clustering
kmeans = KMeans(n_clusters=3, random_state=42)
kmeans.fit(data)
# Calculating the Davies-Bouldin index
db_index = davies_bouldin_score(data, kmeans.labels_)
print(f"The Davies-Bouldin index is : {db_index}")
```
### 2.1.2 External Metrics: Rand Index and Jaccard Coefficient
Unlike internal metrics, external metrics require a reference label (usually the true classification label) to evaluate clustering results. In this section, we will discuss two commonly used external metrics: the Rand Index and the Jaccard Coefficient.
#### Rand Index
The Rand Index (RI) is a metric used to measure the similarity between clustering results and reference labels. Its formula is as follows:
\[ RI = \frac{a+b}{a+b+c+d} \]
Where, \( a \) is the number of times two samples are in the same cluster, \( b \) is the number of times two samples are in different clusters, \( c \) is the number of times two samples are in the same cluster but not in the same reference cluster, and \( d \) is the number of times two samples are in different clusters and not in the same reference cluster.
Next, we provide an example of how to implement the Rand Index in Python:
```python
from sklearn.metrics import rand_score
# Assuming 'true_labels' are the true classification labels and 'clusters' are our clustering results
# The 'rand_score' function is used to calculate the Rand Index
rand_index = rand_score(true_labels, clusters)
print(f"The Rand index is : {rand_index}")
```
#### Jaccard Coefficient
The Jaccard Coefficient is another metric used to measure the similarity between clustering results and reference labels, especially useful in clustering problems because it mainly focuses on the intersection between clusters. Its formula is:
\[ J = \frac{|X \cap Y|}{|X \cup Y|} \]
Where, \( X \) and \( Y \) are clusters in the clustering results and reference labels, respectively.
Here is the Python code example to implement the Jaccard Coefficient:
```python
from sklearn.metrics import jaccard_similarity_score
# Assuming 'clusters' are the clustering results and 'true_labels' are the true classification labels
# The 'jaccard_similarity_score' function is used to calculate the Jaccard Coefficient
jaccard_score = jaccard_similarity_score(true_labels, clusters)
print(f"The Jaccard similarity score is : {jaccard_score}")
```
### 2.1.3 Relative Metrics: Adjusted Rand Index and Dice Coefficient
Relative metrics are a form of evaluation that lies between internal metrics and external metrics. They attempt to incorporate information from reference labels and the nature of clustering algorithms. In this section, we will analyze the adjusted Rand Index and the Dice Coefficient.
#### Adjusted Rand Index
The Adjusted Rand Index (ARI) is an adjusted version of the Rand Index, which provides a corrected similarity measure by reducing the expected similarity when randomly assigning clustering results. The formula is:
\[ ARI = \frac{RI - E[RI]}{\max(RI) - E[RI]} \]
Where, \( RI \) is the Rand Index, and \( E[RI] \) is the expected Rand Index when labels are randomly assigned.
Below is a Python code example for implementing ARI:
```python
from sklearn.metrics import adjusted_rand_score
# Assuming 'true_labels' are the true classification labels and 'clusters' are our clustering results
# The 'adjusted_rand_score' function is used to calculate ARI
adjusted_rand = adjusted_rand_score(true_labels, clusters)
print(f"The Adjusted Rand index is : {adjusted_rand}")
```
#### Dice Coefficient
The Dice Coefficient is a set similarity measure function often used to measure the similarity of two sample sets. Its formula is:
\[ D = \frac{2|X \cap Y|}{|X| + |Y|} \]
In clustering evaluation, the Dice Coefficient can help us understand the similarity between two clustering clusters.
Here is the Python code example to implement the Dice Coefficient:
```python
from sklearn.metrics import fowlkes_mallows_score
# Assuming 'clusters' are the clustering results and 'true_labels' are the true classification labels
# The 'fowlkes_mallows_score' function can be used to calculate the Dice Coefficient
dice_score = fowlkes_mallows_score(true_labels, clusters)
print(f"The Dice similarity score is : {dice_score}")
```
## 2.2 Stability Evaluation of Clustering Algorithms
When performing clustering analysis, stability refers to whether the clustering results remain consistent when there are minor disturbances in the input data. Stability is an important aspect of evaluating the performance of clustering algorithms.
### 2.2.1 Concept and Importance of Stability
Stability is a measure of the consistency of clustering results when facing different datasets. A stable clustering algorithm will produce similar clustering results when faced with data disturbances.
### 2.2.2 Stability Evaluation Methods
One method for evaluating stability is to use datasets with noise. We can add noise to the original dataset and then compare the differences in clustering results before and after adding noise. For example, we can measure the stability of clustering results by calculating the silhouette coefficients between different clustering results.
In the next chapter, we will delve into the complexity evaluation of clustering algorithms, including the introduction of time complexity and space complexity, ***plexity evaluation provides us with insight into the efficiency of clustering algorithms, which is important not only for theoretical researchers but also for selection and optimization in practical applications.
# 3. Unsupervised Learning Evaluation Tools and Practice
## 3.1 Introduction to Common Evaluation Tools
### 3.1.1 Scikit-learn Evaluation Module in Python
In the process of evaluating clustering algorithms, Python's scikit-learn library provides a rich set of evaluation tools. Submodules within `sklearn.metrics` include various functions for measuring clustering performance. For example, the sil
0
0
相关推荐
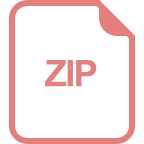
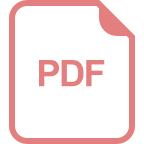
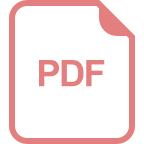
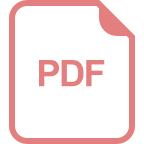
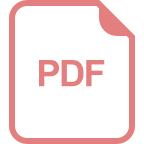
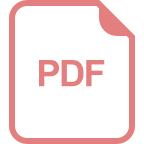
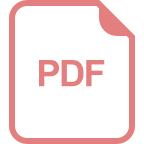
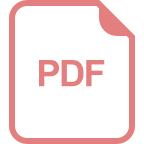
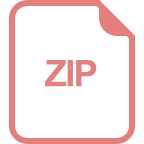