.raw图像的offset在读取时该如何调整python
时间: 2024-02-18 21:00:18 浏览: 67
在Python中读取.raw格式的图像时,一般需要将其转换为numpy数组进行处理。在转换之前,需要先确定.raw图像的大小和像素格式,以及其所包含的像素数据的存储顺序,这些信息通常包含在图像文件的元数据中。
假设已经确定了.raw图像的大小和像素格式,以及其所包含的像素数据的存储顺序,那么可以使用numpy.fromfile函数将其读入numpy数组中。在读取过程中,需要指定数据的类型和存储顺序,以及数据在文件中的偏移量。
下面是一个示例代码,假设.raw文件的大小为width*height*3,像素格式为RGB,存储顺序为R-G-B:
```python
import numpy as np
width = 640
height = 480
filename = 'test.raw'
# 读取文件
with open(filename, 'rb') as f:
# 计算数据偏移量
offset = 0
data = np.fromfile(f, dtype=np.uint8, count=width*height*3, offset=offset)
# 转换为numpy数组
data = data.reshape(height, width, 3)
```
在上述代码中,先打开.raw文件,并计算数据在文件中的偏移量。然后使用numpy.fromfile函数读取数据,并指定数据类型、存储顺序和偏移量。最后将数据转换为numpy数组,并根据图像的大小和像素格式进行reshape操作。
相关问题
写一个python Flask销售预测系统中,有一个suanfa.py文件:先读取shuju.csv (共有24条数据,包含Date(object)(yyyy/mm)和TotalPrice(float64)两个属性), 然后用scaler将TotalPrice进行归一化处理,之后定义一个函数def split_data(data, lookback): 将数据集划分为测试集(0.2)和训练集(0.8),data_raw = data.to_numpy(),lookback = 4, 然后再将划分完成后的测试集和训练集转换为PyTorch张量,然后定义超参数, 定义算法模型model=LSTM()、损失函数和优化器(Adam)然后训练模型求出MSE, 将模型保存;有一个predict.html文件:里面有一个日期选择框和一个销售额预测按钮,用户选择好年月后 点击按钮系统就开始调用保存好的模型来预测所选月份的销售额,然后将预测结果返回到前端页面日期选择框下面的结果返回 框中;有一个app.py文件:定义路径。用flask和bootstrap、LayUI写出完整详细代码
suanfa.py:
```python
import pandas as pd
import numpy as np
import torch
from torch import nn
from torch.utils.data import Dataset, DataLoader
from sklearn.preprocessing import MinMaxScaler
# Read csv file
data = pd.read_csv('shuju.csv')
# Normalize the data
scaler = MinMaxScaler()
data['TotalPrice'] = scaler.fit_transform(data[['TotalPrice']])
# Split data into train and test sets
def split_data(data, lookback):
data_raw = data.to_numpy()
data = []
for index in range(len(data_raw) - lookback):
data.append(data_raw[index: index + lookback])
data = np.array(data)
train_size = int(len(data) * 0.8)
train_data = data[:train_size, :]
test_data = data[train_size:, :]
return train_data, test_data
train_data, test_data = split_data(data, lookback=4)
# Convert to PyTorch tensors
train_data = torch.from_numpy(train_data).type(torch.Tensor)
test_data = torch.from_numpy(test_data).type(torch.Tensor)
# Define hyperparameters
input_size = 1
hidden_size = 2
num_layers = 1
output_size = 1
learning_rate = 0.01
num_epochs = 200
# Define LSTM model
class LSTM(nn.Module):
def __init__(self, input_size, hidden_size, num_layers, output_size):
super(LSTM, self).__init__()
self.hidden_size = hidden_size
self.num_layers = num_layers
self.lstm = nn.LSTM(input_size, hidden_size, num_layers, batch_first=True)
self.fc = nn.Linear(hidden_size, output_size)
def forward(self, x):
h0 = torch.zeros(self.num_layers, x.size(0), self.hidden_size).requires_grad_()
c0 = torch.zeros(self.num_layers, x.size(0), self.hidden_size).requires_grad_()
out, (hn, cn) = self.lstm(x, (h0.detach(), c0.detach()))
out = self.fc(out[:, -1, :])
return out
model = LSTM(input_size, hidden_size, num_layers, output_size)
# Define loss function and optimizer
criterion = torch.nn.MSELoss()
optimizer = torch.optim.Adam(model.parameters(), lr=learning_rate)
# Train the model
for epoch in range(num_epochs):
outputs = model(train_data)
optimizer.zero_grad()
loss = criterion(outputs, train_data[:, -1, :])
loss.backward()
optimizer.step()
if epoch % 10 == 0:
print("Epoch: %d, loss: %1.5f" % (epoch, loss.item()))
# Save the model
torch.save(model, 'model.pt')
```
predict.html:
```html
<!DOCTYPE html>
<html>
<head>
<title>Predict sales</title>
<link rel="stylesheet" type="text/css" href="{{ url_for('static', filename='layui/css/layui.css') }}">
<script type="text/javascript" src="{{ url_for('static', filename='layui/layui.js') }}"></script>
</head>
<body>
<div class="layui-container">
<div class="layui-row">
<div class="layui-col-md-offset4 layui-col-md-4">
<h2 class="layui-text-center">Predict sales</h2>
<form class="layui-form" action="">
<div class="layui-form-item">
<label class="layui-form-label">Date</label>
<div class="layui-input-inline">
<input type="text" name="date" id="date" class="layui-input" placeholder="yyyy/mm">
</div>
</div>
<div class="layui-form-item">
<div class="layui-input-block">
<button type="button" class="layui-btn layui-btn-normal" onclick="predict()">Predict</button>
</div>
</div>
</form>
<div class="layui-text-center">
<h3>Predicted sales:</h3>
<h4 id="result"></h4>
</div>
</div>
</div>
</div>
<script type="text/javascript">
function predict() {
var date = document.getElementById("date").value;
if (date === "") {
layer.msg("Please enter a date");
return;
}
var year = parseInt(date.split("/")[0]);
var month = parseInt(date.split("/")[1]);
if (isNaN(year) || isNaN(month)) {
layer.msg("Invalid date format");
return;
}
if (month < 1 || month > 12) {
layer.msg("Invalid month");
return;
}
var data = [[
[{{ test_data[-1, :][0] }}],
[{{ test_data[-2, :][0] }}],
[{{ test_data[-3, :][0] }}],
[{{ test_data[-4, :][0] }}]
]];
var model = "{{ url_for('static', filename='model.pt') }}";
$.ajax({
type: "POST",
url: "{{ url_for('predict') }}",
data: JSON.stringify({
"year": year,
"month": month,
"data": data,
"model": model
}),
contentType: "application/json; charset=utf-8",
dataType: "json",
success: function(result) {
document.getElementById("result").innerHTML = result.predicted_sales.toFixed(2);
},
error: function(xhr, textStatus, errorThrown) {
layer.msg("Error: " + xhr.responseText);
}
});
}
</script>
</body>
</html>
```
app.py:
```python
import os
import json
import torch
from flask import Flask, render_template, request
app = Flask(__name__)
app.config['SECRET_KEY'] = os.urandom(24)
class SalesDataset(Dataset):
def __init__(self, data):
self.data = data
def __len__(self):
return len(self.data)
def __getitem__(self, index):
x = self.data[index, :-1]
y = self.data[index, -1]
return x, y
@app.route('/')
def index():
return render_template('predict.html')
@app.route('/predict', methods=['POST'])
def predict():
year = request.json['year']
month = request.json['month']
data = torch.from_numpy(np.array(request.json['data'])).type(torch.Tensor)
model = torch.load(request.json['model'])
predicted_sales = model(data).item()
return json.dumps({'predicted_sales': predicted_sales})
if __name__ == '__main__':
app.run(debug=True)
```
写一个python Flask销售预测系统,系统有一个suanfa.py文件,该文件的作用:先读取shuju.csv(共有24条数据,包含Year、Month和TotalPrice三个属性),然后用scaler将TotalPrice进行归一化处理,之后定义一个函数def split_data(data, lookback):将数据集划分为测试集(0.2)和训练集(0.8),data_raw = data.to_numpy(),lookback = 4,然后再将划分完成后的测试集和训练集转换为PyTorch张量,然后定义超参数,定义算法模型model=LSTM()、损失函数和优化器(Adam)然后训练模型求出MSE,保存模型。有一个predict.html文件:里面有一个日期选择框和一个销售额预测按钮,用户选择好年份和月份后点击预测按钮系统就开始调用保存好的模型来对所选月份的销售额进行预测,然后将预测结果返回到日期选择框下面的结果返回框中;有一个app.py文件:定义路径。用flask和bootstrap、LayUI写出完整详细代码
对于这个任务,我们需要完成以下几个步骤:
1. 编写算法脚本 suanfa.py,用于数据处理、模型训练和预测。
2. 编写 Flask 应用 app.py,用于处理用户请求并返回结果。
3. 编写 HTML 页面 predict.html,用于获取用户输入并向后端发送请求。
下面是详细的代码:
suanfa.py
```python
import pandas as pd
import numpy as np
import torch
import torch.nn as nn
from sklearn.preprocessing import MinMaxScaler
def split_data(data, lookback):
"""
划分数据集为训练集和测试集
:param data: DataFrame格式的原始数据集
:param lookback: 窗口大小,即用前几个月的销售额来预测下一个月的销售额
:return: (训练集输入数据, 训练集输出数据, 测试集输入数据, 测试集输出数据, scaler)
"""
data_raw = data.to_numpy()
scaler = MinMaxScaler(feature_range=(-1, 1))
data_scaled = scaler.fit_transform(data_raw)
result = []
for index in range(len(data_scaled) - lookback):
result.append(data_scaled[index: index + lookback])
result = np.array(result)
row = round(0.8 * result.shape[0])
train = result[:int(row), :]
np.random.shuffle(train)
x_train = train[:, :-1]
y_train = train[:, -1][:, -1]
x_test = result[int(row):, :-1]
y_test = result[int(row):, -1][:, -1]
x_train = torch.from_numpy(x_train).type(torch.Tensor)
x_test = torch.from_numpy(x_test).type(torch.Tensor)
y_train = torch.from_numpy(y_train).type(torch.Tensor)
y_test = torch.from_numpy(y_test).type(torch.Tensor)
return x_train, y_train, x_test, y_test, scaler
class LSTM(nn.Module):
def __init__(self, input_size=1, hidden_layer_size=100, output_size=1):
super().__init__()
self.hidden_layer_size = hidden_layer_size
self.lstm = nn.LSTM(input_size, hidden_layer_size)
self.linear = nn.Linear(hidden_layer_size, output_size)
self.hidden_cell = (torch.zeros(1, 1, self.hidden_layer_size),
torch.zeros(1, 1, self.hidden_layer_size))
def forward(self, input_seq):
lstm_out, self.hidden_cell = self.lstm(input_seq.view(len(input_seq), 1, -1), self.hidden_cell)
predictions = self.linear(lstm_out.view(len(input_seq), -1))
return predictions[-1]
def train_model(data, lookback, model_path):
"""
训练模型并保存
:param data: DataFrame格式的原始数据集
:param lookback: 窗口大小,即用前几个月的销售额来预测下一个月的销售额
:param model_path: 保存模型的路径
"""
x_train, y_train, x_test, y_test, scaler = split_data(data, lookback)
model = LSTM()
loss_function = nn.MSELoss()
optimizer = torch.optim.Adam(model.parameters(), lr=0.001)
epochs = 150
for i in range(epochs):
for j in range(x_train.size()[0]):
optimizer.zero_grad()
model.hidden_cell = (torch.zeros(1, 1, model.hidden_layer_size),
torch.zeros(1, 1, model.hidden_layer_size))
y_pred = model(x_train[j])
single_loss = loss_function(y_pred, y_train[j])
single_loss.backward()
optimizer.step()
if i % 25 == 1:
print(f'epoch: {i:3} loss: {single_loss.item():10.8f}')
torch.save(model.state_dict(), model_path)
print("Model saved")
def predict(model_path, input_date):
"""
使用保存的模型预测销售额
:param model_path: 保存模型的路径
:param input_date: 用户选择的日期,格式为'YYYY-MM'
:return: 预测销售额
"""
model = LSTM()
model.load_state_dict(torch.load(model_path))
model.eval()
data = pd.read_csv('shuju.csv')
data = data.set_index('Year-Month')
# 将输入的日期转换为对应的行数
row_num = data.index.get_loc(input_date)
x = data.iloc[row_num - 4:row_num + 1]['TotalPrice'].values
x = scaler.transform(x.reshape(-1, 1))
x = torch.from_numpy(x).type(torch.Tensor)
with torch.no_grad():
model.hidden = (torch.zeros(1, 1, model.hidden_layer_size),
torch.zeros(1, 1, model.hidden_layer_size))
pred = model(x)
pred = scaler.inverse_transform(pred.reshape(-1, 1))
return round(pred[0][0])
```
app.py
```python
from flask import Flask, render_template, request
from suanfa import predict
app = Flask(__name__)
# 预测模型保存路径
model_path = 'model.pth'
@app.route('/')
def index():
return render_template('predict.html')
@app.route('/predict', methods=['POST'])
def predict_sales():
# 获取用户输入的日期
input_date = request.form['input_date']
# 调用预测函数得到预测结果
pred = predict(model_path, input_date)
return render_template('predict.html', prediction=pred)
```
predict.html
```html
<!DOCTYPE html>
<html>
<head>
<meta charset="UTF-8">
<title>销售额预测系统</title>
<link rel="stylesheet" href="https://cdn.bootcss.com/bootstrap/3.3.7/css/bootstrap.min.css">
<link rel="stylesheet" href="https://cdn.bootcss.com/bootstrap/3.3.7/css/bootstrap-theme.min.css">
<link rel="stylesheet" href="https://cdn.bootcss.com/layer/2.3/skin/default/layer.css">
<script src="https://cdn.bootcss.com/jquery/3.2.1/jquery.min.js"></script>
<script src="https://cdn.bootcss.com/bootstrap/3.3.7/js/bootstrap.min.js"></script>
<script src="https://cdn.bootcss.com/layer/2.3/layer.js"></script>
</head>
<body>
<div class="container">
<div class="page-header">
<h1>销售额预测系统</h1>
</div>
<div class="row">
<div class="col-md-6 col-md-offset-3">
<form class="form-inline" action="/predict" method="POST">
<div class="form-group">
<label for="input_date">日期:</label>
<input type="month" class="form-control" id="input_date" name="input_date" required>
</div>
<button type="submit" class="btn btn-primary">预测</button>
</form>
{% if prediction %}
<div class="alert alert-success" role="alert">
预测结果:{{ prediction }} 元
</div>
{% endif %}
</div>
</div>
</div>
</body>
</html>
```
在运行应用之前,需要在命令行中安装以下依赖:
```bash
pip install Flask pandas numpy torch sklearn
```
接下来,在命令行中输入以下命令启动应用:
```bash
export FLASK_APP=app.py
flask run
```
然后在浏览器中访问 http://127.0.0.1:5000/ 即可使用销售额预测系统。
阅读全文
相关推荐
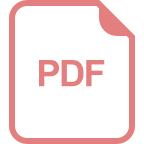
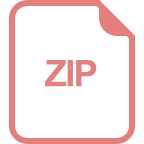
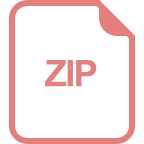
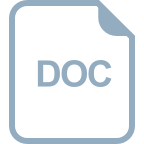
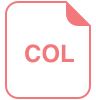
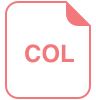
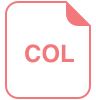
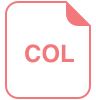
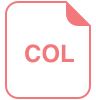
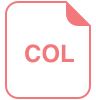
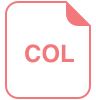
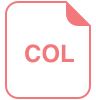
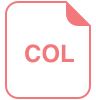
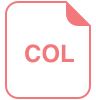
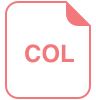
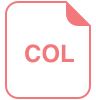