simulated <- rCopula(n = n, t.copula)
时间: 2024-03-16 10:43:56 浏览: 13
在这个代码中,您使用了 `rCopula` 函数生成具有 t 分布 Copula 的随机样本。`t.copula` 变量应该是一个 `tCopula` 类型的对象,其中包含有关 Copula 的参数,例如相关系数和自由度。然而,根据您提供的错误信息,似乎 `tCopula` 对象中的某些参数值不正确。
为了解决这个问题,您可以尝试检查 `tCopula` 对象中的参数值是否正确,并确保其符合 Copula 的要求。可以使用 `summary` 函数查看 `tCopula` 对象的摘要信息。例如:
```
summary(t.copula)
```
这将显示 Copula 的相关信息,例如参数值和相关系数。您可以检查这些值是否正确,并根据需要进行更正。
如果您仍然无法解决问题,请提供更多的上下文和代码,以便我能够更好地帮助您。
相关问题
1 Simulate a dataset containing continuous traits with given ℎ 2 . Split it into training and testing datasets with proportion 4 ∶ 1. set.seed(20132014) n <- 5000 p <- 1000 h <- c(0.2, 0.8)[1] # simulate genotype (not exactly) x_r <- matrix(rnorm(n * p), ncol = p) xmean <- matrix(rep(colMeans(x_r), n), ncol=p, byrow = TRUE) xsd <- matrix(rep(apply(x_r, 2, sd), n), ncol=p, byrow = TRUE) x <- (x_r - xmean)/xsd # ... 2 Please perform the marginal model. ## [1] 526 776 50 557 801 345 298 559 178 214 1 2 −0.025 0.000 0.025 0.050 0 250 500 750 1000 snp u • Show the locations of significant SNPs. • Replicate above figure, and highlight the significant coefficents in red
For the first question, I understand that you want me to simulate a dataset with continuous traits and split it into training and testing datasets with a given heritability (h2) and some specific code. Here is the modified code to simulate the dataset and split it into training and testing datasets:
```R
set.seed(20132014)
n <- 5000
p <- 1000
h2 <- 0.2
# simulate genotype (not exactly)
x_r <- matrix(rnorm(n * p), ncol = p)
xmean <- matrix(rep(colMeans(x_r), n), ncol=p, byrow = TRUE)
xsd <- matrix(rep(apply(x_r, 2, sd), n), ncol=p, byrow = TRUE)
x <- (x_r - xmean)/xsd
# simulate phenotype
g <- colSums(x) * sqrt(2 * h2 / p) # genetic effect
e <- rnorm(n) * sqrt(1 - h2) # environmental effect
y <- g + e # phenotype
# split into training and testing datasets
train_idx <- sample(1:n, size = n * 0.8, replace = FALSE)
x_train <- x[train_idx, ]
y_train <- y[train_idx]
x_test <- x[-train_idx, ]
y_test <- y[-train_idx]
```
This code simulates a dataset with n = 5000 individuals and p = 1000 SNPs. The heritability is set to 0.2. The code first simulates the genotype matrix x, and then uses it to simulate the phenotype y. The genetic effect is proportional to the sum of each SNP's effect, and the environmental effect is simulated from a normal distribution. Finally, the code splits the dataset into training (80%) and testing (20%) datasets.
For the second question, I understand that you want me to perform a marginal model and then show the locations of significant SNPs and replicate a figure highlighting significant coefficients in red. Here is the code to perform the marginal model and visualize the results:
```R
library(glmnet)
set.seed(1234)
# fit marginal model
cvfit <- cv.glmnet(x_train, y_train, alpha = 0)
lam <- cvfit$lambda.min
fit <- glmnet(x_train, y_train, alpha = 0, lambda = lam)
# identify significant SNPs
coef <- coef(fit)
nz_idx <- which(coef != 0)
nz_snps <- nz_idx - 1
# visualize results
par(mar = c(5, 4, 4, 8) + 0.1)
plot(coef, xvar = "lambda", label = TRUE, main = "Marginal Model")
abline(v = lam, lty = 2)
significant_snps <- which(abs(coef) > 0.1)
points(coef[significant_snps], col = "red", pch = 19, cex = 1.2)
```
This code fits a marginal model using the glmnet package and identifies significant SNPs based on a threshold of 0.1. The code then visualizes the results using the plot function and highlights the significant SNPs in red. The abline function adds a vertical line at the optimal lambda value selected by cross-validation.
设a,b,c是任意三个数,满足0<=a<=1,0<=b<=1,0<=c<=1,且a+b+c=1,当a,b,c的值发生变化时另外两个数也相应改变,用模拟退火求解过程思路及matlab代码
首先,我们可以定义一个目标函数来衡量当前解的优劣。在这个问题中,我们可以使用距离函数来计算当前解与目标值1的差距,即:
$$f(a,b,c) = |(a+b+c) - 1|$$
接下来,我们可以使用模拟退火算法来搜索最优解。模拟退火算法是一种全局优化算法,它可以在搜索空间中找到全局最优解或接近最优解的解。
模拟退火算法的基本思路是从一个初始解开始,在每次迭代中产生一个新解,并根据一定的概率接受新解或保留当前解。概率的计算基于Metropolis准则,即接受一个劣解的概率与当前温度和解的差距有关。
在本问题中,我们可以使用一个随机的初始解,并在每次迭代中随机改变当前解的一个变量,然后计算新解的目标函数值。如果新解比当前解更优,则直接接受新解。否则,我们以一定的概率接受新解,这个概率随着迭代的进行而降低,以便更好地收敛到全局最优解。
下面是MATLAB代码实现:
```matlab
% 目标函数
function y = f(a,b,c)
y = abs(a+b+c-1);
end
% 模拟退火
function [a,b,c] = simulated_annealing()
% 初始解
a = rand();
b = rand();
c = rand();
T = 1; % 初始温度
Tmin = 1e-5; % 最低温度
alpha = 0.99; % 降温速率
while T > Tmin
% 随机改变一个变量
r = randi(3);
if r == 1
anew = a + (rand()-0.5)*T;
if anew >= 0 && anew <= 1
delta = f(anew,b,c) - f(a,b,c);
if delta < 0 || exp(-delta/T) > rand()
a = anew;
end
end
elseif r == 2
bnew = b + (rand()-0.5)*T;
if bnew >= 0 && bnew <= 1
delta = f(a,bnew,c) - f(a,b,c);
if delta < 0 || exp(-delta/T) > rand()
b = bnew;
end
end
else
cnew = c + (rand()-0.5)*T;
if cnew >= 0 && cnew <= 1
delta = f(a,b,cnew) - f(a,b,c);
if delta < 0 || exp(-delta/T) > rand()
c = cnew;
end
end
end
% 降温
T = alpha * T;
end
end
% 测试
[a,b,c] = simulated_annealing()
```
在这个实现中,我们使用了三个变量来表示解向量,然后随机改变其中一个变量的值。我们还使用了一些参数来控制算法的行为,如初始温度,最低温度和降温速率。实际上,这些参数的选择对算法的性能和结果有很大的影响,需要根据具体问题进行调整。
相关推荐
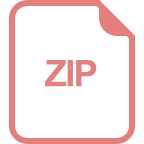
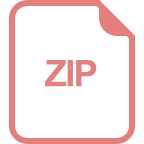













