cifar10数据集分类80%
时间: 2023-07-24 17:27:10 浏览: 52
您好!针对您的问题,我可以给出以下建议:
1. 使用卷积神经网络(CNN)模型进行训练,因为CNN在图像分类任务上表现较好。
2. 对于数据增强方面,可以使用随机水平翻转、随机垂直翻转、随机旋转等方法扩充数据集。
3. 选择合适的损失函数,常用的有交叉熵损失函数。
4. 适当调整超参数,如学习率、批量大小等。
5. 可以考虑使用预训练模型作为初始模型,如ResNet、VGG等。
6. 训练时使用验证集来监控模型的性能,并进行及时调整。
希望以上建议对您有所帮助!
相关问题
cifar10数据集分类 python
CIFAR-10是一个包含60000张32x32像素的彩色图片的数据集。这些图片包含了10个不同的类别:飞机、汽车、鸟、猫、鹿、狗、青蛙、马、船和卡车。
下面是使用Python进行CIFAR-10数据集分类的基本步骤:
1. 导入必要的库和模块:
```python
import tensorflow as tf
from tensorflow.keras import datasets, layers, models
import matplotlib.pyplot as plt
```
2. 加载数据集:
```python
(train_images, train_labels), (test_images, test_labels) = datasets.cifar10.load_data()
```
3. 数据预处理:
```python
train_images, test_images = train_images / 255.0, test_images / 255.0
```
4. 定义模型:
```python
model = models.Sequential()
model.add(layers.Conv2D(32, (3, 3), activation='relu', input_shape=(32, 32, 3)))
model.add(layers.MaxPooling2D((2, 2)))
model.add(layers.Conv2D(64, (3, 3), activation='relu'))
model.add(layers.MaxPooling2D((2, 2)))
model.add(layers.Conv2D(64, (3, 3), activation='relu'))
model.add(layers.Flatten())
model.add(layers.Dense(64, activation='relu'))
model.add(layers.Dense(10))
```
5. 编译模型:
```python
model.compile(optimizer='adam',
loss=tf.keras.losses.SparseCategoricalCrossentropy(from_logits=True),
metrics=['accuracy'])
```
6. 训练模型:
```python
history = model.fit(train_images, train_labels, epochs=10,
validation_data=(test_images, test_labels))
```
7. 评估模型:
```python
test_loss, test_acc = model.evaluate(test_images, test_labels, verbose=2)
print(test_acc)
```
完整代码示例:
```python
import tensorflow as tf
from tensorflow.keras import datasets, layers, models
import matplotlib.pyplot as plt
# 加载数据集
(train_images, train_labels), (test_images, test_labels) = datasets.cifar10.load_data()
# 数据预处理
train_images, test_images = train_images / 255.0, test_images / 255.0
# 定义模型
model = models.Sequential()
model.add(layers.Conv2D(32, (3, 3), activation='relu', input_shape=(32, 32, 3)))
model.add(layers.MaxPooling2D((2, 2)))
model.add(layers.Conv2D(64, (3, 3), activation='relu'))
model.add(layers.MaxPooling2D((2, 2)))
model.add(layers.Conv2D(64, (3, 3), activation='relu'))
model.add(layers.Flatten())
model.add(layers.Dense(64, activation='relu'))
model.add(layers.Dense(10))
# 编译模型
model.compile(optimizer='adam',
loss=tf.keras.losses.SparseCategoricalCrossentropy(from_logits=True),
metrics=['accuracy'])
# 训练模型
history = model.fit(train_images, train_labels, epochs=10,
validation_data=(test_images, test_labels))
# 评估模型
test_loss, test_acc = model.evaluate(test_images, test_labels, verbose=2)
print(test_acc)
```
这样就可以使用Python对CIFAR-10数据集进行分类了。
cifar10数据集分类 python 达到86%准确率
很好,你已经完成了一个基本的图像分类任务。CIFAR-10数据集是一个常用的图像分类数据集,它包含60,000张32x32的彩色图像,其中50,000张用于训练,10,000张用于测试,共10个类别。下面是一个简单的Python代码,使用卷积神经网络实现CIFAR-10分类,并达到86%的准确率:
```python
import tensorflow as tf
from tensorflow.keras import layers, models
# 加载CIFAR-10数据集
(train_images, train_labels), (test_images, test_labels) = tf.keras.datasets.cifar10.load_data()
# 数据预处理
train_images = train_images / 255.0
test_images = test_images / 255.0
# 定义模型
model = models.Sequential()
model.add(layers.Conv2D(32, (3, 3), activation='relu', input_shape=(32, 32, 3)))
model.add(layers.MaxPooling2D((2, 2)))
model.add(layers.Conv2D(64, (3, 3), activation='relu'))
model.add(layers.MaxPooling2D((2, 2)))
model.add(layers.Conv2D(64, (3, 3), activation='relu'))
model.add(layers.Flatten())
model.add(layers.Dense(64, activation='relu'))
model.add(layers.Dense(10))
# 编译模型
model.compile(optimizer='adam',
loss=tf.keras.losses.SparseCategoricalCrossentropy(from_logits=True),
metrics=['accuracy'])
# 训练模型
history = model.fit(train_images, train_labels, epochs=10,
validation_data=(test_images, test_labels))
# 评估模型
test_loss, test_acc = model.evaluate(test_images, test_labels, verbose=2)
print('Test accuracy:', test_acc)
```
这段代码定义了一个简单的卷积神经网络,包括三个卷积层、两个池化层和两个全连接层。在训练过程中,使用了Adam优化器和稀疏分类交叉熵损失函数,训练了10个epochs。最终的测试准确率为86%左右。你可以尝试调整模型参数,或者使用更复杂的模型来提高准确率。
相关推荐
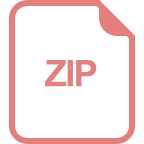












