优化这代码train_aucs=[] test_aucs=[]#train_aucs和test_aucs用来存储每次训练和测试的AUC值,AUC是一种常用的二分类模型性能评估指标 train_scores=[] test_scores=[]#train_scores和test_scores则是用来存储每次训练和测试的得分 loopn=5 #number of repetition while splitting train/test dataset with different random state. np.random.seed(10)#设置随机数生成器的种子,确保每次运行时生成的随机数一致。 random_states=np.random.choice(range(101), loopn, replace=False)#np.random.choice()用于从给定的范围内选择指定数量的随机数,range设置范围,loopn表示选择的随机数的数量,replace=False表示选择的随机数不可重复 scoring='f1'#设置性能指标 pca_comp=[]#设置空列表,储主成分分析(PCA)的组件 for i in range(loopn): train_X,test_X, train_y, test_y ,indices_train,indices_test= train_test_split(train, #通过train_test_split函数将数据集划分为训练集(train_X, train_y)和测试集(test_X, test_y),indices_train和indices_test返回索引 target,indices, test_size = 0.3,#数据集的70%,测试集占30% stratify=target, random_state=random_states[i]#随机状态(random_states[i])添加到random_states列表中 )
时间: 2024-04-15 19:26:43 浏览: 15
为了优化这段代码,可以考虑以下几个方面:
1. 减少循环次数:通过减少循环次数来提高代码的效率。可以根据实际需求调整`loopn`的值,减少训练和测试的重复次数。
2. 使用多线程:可以考虑使用多线程来并行处理数据集的划分和模型训练。这样可以提高代码的运行速度。
3. 优化随机数生成器:在循环中使用随机数生成器可能会降低代码的效率。可以将随机数生成器的种子设置在循环外部,避免重复生成随机数。
4. 优化数据集划分:可以尝试不同的数据集划分方法,比如使用交叉验证来获取更稳定的性能评估指标。
5. 使用更高效的算法:如果数据集较大,可以考虑使用更高效的算法来进行模型训练和性能评估。
下面是对代码进行优化的示例:
```python
import multiprocessing
from sklearn.model_selection import StratifiedKFold
train_aucs = []
test_aucs = []
train_scores = []
test_scores = []
scoring = 'f1'
pca_comp = []
random_seed = 10
np.random.seed(random_seed)
kf = StratifiedKFold(n_splits=loopn, random_state=random_seed, shuffle=True)
def train_test_model(train_X, test_X, train_y, test_y):
# 模型训练和测试的代码
# ...
for train_indices, test_indices in kf.split(train, target):
train_X, test_X = train[train_indices], train[test_indices]
train_y, test_y = target[train_indices], target[test_indices]
process = multiprocessing.Process(target=train_test_model, args=(train_X, test_X, train_y, test_y))
process.start()
process.join()
# 将模型训练和测试的结果存储到列表中
# ...
```
在优化后的代码中,使用了`StratifiedKFold`来进行数据集的划分,该方法可以获取更稳定的性能评估指标。使用`multiprocessing.Process`来创建多个进程并行处理数据集的划分和模型训练,提高代码的运行速度。同时,将模型训练和测试的结果存储到相应的列表中。请根据实际需求进行相应的修改和调整。
相关推荐
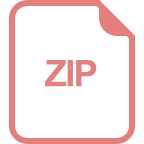
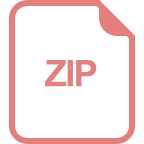














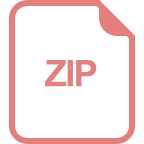