tf.nn.softmax_cross_entropy_with_logits的计算方法
时间: 2023-02-23 18:11:07 浏览: 74
`tf.nn.softmax_cross_entropy_with_logits` 是 TensorFlow 中的一个函数,它可以在一次计算中同时实现 softmax 函数和交叉熵损失函数的计算。
具体而言,这个函数的计算方法如下:
1. 首先将给定的 logits 进行 softmax 函数计算,得到预测概率分布。
2. 然后,计算真实标签(one-hot 编码)与预测概率分布之间的交叉熵。
3. 最终,计算所有样本的交叉熵的平均值作为最终的损失函数。
通过使用 `tf.nn.softmax_cross_entropy_with_logits` 函数,可以避免手动实现 softmax 函数和交叉熵损失函数的过程,并且可以加速计算。
相关问题
tf.nn.softmax_cross_entropy_with_logits
tf.nn.softmax_cross_entropy_with_logits是TensorFlow中用于计算多分类问题的交叉熵损失函数。它计算输入的logits与标签之间的交叉熵,并使用softmax函数将logits转化为概率分布。这个函数可以帮助模型计算分类错误的概率,从而在训练过程中不断优化模型的预测能力。
tf.nn.sparse_softmax_cross_entropy_with_logits
tf.nn.sparse_softmax_cross_entropy_with_logits is a function in the TensorFlow library that computes the softmax cross-entropy loss between the logits and the true labels. It is used for multi-class classification problems where the target labels are integers rather than one-hot encoded vectors. The function takes two arguments: logits and labels. The logits are the unnormalized log probabilities computed by the model, while the labels are the true class labels. The function computes the cross-entropy loss by first applying the softmax function to the logits and then calculating the negative log likelihood of the true labels. The resulting loss is a scalar value that can be used to optimize the model parameters using gradient descent algorithms.
相关推荐
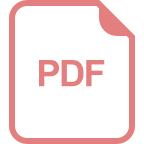
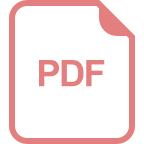
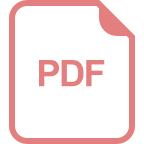













