翻译一下 shuffle_data = merge_data.sample(frac=1.0) shuffle_data = shuffle_data.reset_index(drop=True)
时间: 2024-06-03 18:09:45 浏览: 13
Sure, I can translate that for you. "shuffle_data = merge_data.sample(frac=1.0)" means that the code randomly samples (or shuffles) the data in "merge_data" with a fraction of 1.0, which means all the data will be included in the shuffle. "shuffle_data = shuffle_data.reset_index(drop=True)" means that the code resets the index of the shuffled data to a new sequence, starting from 0 and dropping the old index. Is there anything else you would like to ask?
相关问题
pc_represent_fund_merge_out=pc_represent_fund_merge_out.style.highlight_null(null_color='orange') 'Styler' object is not subscriptable
这个错误是因为你正在尝试对一个 `Styler` 对象进行索引,而 `Styler` 对象是不支持索引的。如果你想要对 `Styler` 对象进行索引,你需要先将其转换为 `DataFrame` 对象。例如:
```
pc_represent_fund_merge_out = pc_represent_fund_merge_out.style.highlight_null(null_color='orange').data
```
这样,你就可以对 `pc_represent_fund_merge_out` 进行索引了。
import pandas as pd import math as mt import numpy as np from sklearn.model_selection import train_test_split from Recommenders import SVDRecommender triplet_dataset_sub_song_merged = triplet_dataset_sub_song_mergedpd triplet_dataset_sub_song_merged_sum_df = triplet_dataset_sub_song_merged[['user','listen_count']].groupby('user').sum().reset_index() triplet_dataset_sub_song_merged_sum_df.rename(columns={'listen_count':'total_listen_count'},inplace=True) triplet_dataset_sub_song_merged = pd.merge(triplet_dataset_sub_song_merged,triplet_dataset_sub_song_merged_sum_df) triplet_dataset_sub_song_merged['fractional_play_count'] = triplet_dataset_sub_song_merged['listen_count']/triplet_dataset_sub_song_merged small_set = triplet_dataset_sub_song_merged user_codes = small_set.user.drop_duplicates().reset_index() song_codes = small_set.song.drop_duplicates().reset_index() user_codes.rename(columns={'index':'user_index'}, inplace=True) song_codes.rename(columns={'index':'song_index'}, inplace=True) song_codes['so_index_value'] = list(song_codes.index) user_codes['us_index_value'] = list(user_codes.index) small_set = pd.merge(small_set,song_codes,how='left') small_set = pd.merge(small_set,user_codes,how='left') mat_candidate = small_set[['us_index_value','so_index_value','fractional_play_count']] data_array = mat_candidate.fractional_play_count.values row_array = mat_candidate.us_index_value.values col_array = mat_candidate.so_index_value.values data_sparse = coo_matrix((data_array, (row_array, col_array)),dtype=float) K=50 urm = data_sparse MAX_PID = urm.shape[1] MAX_UID = urm.shape[0] recommender = SVDRecommender(K) U, S, Vt = recommender.fit(urm) Compute recommendations for test users uTest = [1,6,7,8,23] uTest_recommended_items = recommender.recommend(uTest, urm, 10) Output recommended songs in a dataframe recommendations = pd.DataFrame(columns=['user','song', 'score','rank']) for user in uTest: rank = 1 for song_index in uTest_recommended_items[user, 0:10]: song = small_set.loc[small_set['so_index_value'] == song_index].iloc[0] # Get song details recommendations = recommendations.append({'user': user, 'song': song['title'], 'score': song['fractional_play_count'], 'rank': rank}, ignore_index=True) rank += 1 display(recommendations)这段代码报错了,为什么?给出修改后的 代码
代码中的错误是使用了未定义的模块和类。需要先安装相应的模块并导入相应的类。
以下是修改后的代码:
```python
import pandas as pd
import numpy as np
from scipy.sparse import coo_matrix
from sklearn.model_selection import train_test_split
from Recommenders.SVDRecommender import SVDRecommender # 导入SVDRecommender类
triplet_dataset_sub_song_merged = pd.read_csv('triplet_dataset_sub_song_merged.csv') # 读取数据
triplet_dataset_sub_song_merged_sum_df = triplet_dataset_sub_song_merged[['user','listen_count']].groupby('user').sum().reset_index()
triplet_dataset_sub_song_merged_sum_df.rename(columns={'listen_count':'total_listen_count'},inplace=True)
triplet_dataset_sub_song_merged = pd.merge(triplet_dataset_sub_song_merged,triplet_dataset_sub_song_merged_sum_df)
triplet_dataset_sub_song_merged['fractional_play_count'] = triplet_dataset_sub_song_merged['listen_count']/triplet_dataset_sub_song_merged['total_listen_count']
small_set = triplet_dataset_sub_song_merged[['user','song','fractional_play_count']]
user_codes = small_set.user.drop_duplicates().reset_index()
song_codes = small_set.song.drop_duplicates().reset_index()
user_codes.rename(columns={'index':'user_index'}, inplace=True)
song_codes.rename(columns={'index':'song_index'}, inplace=True)
song_codes['so_index_value'] = list(song_codes.index)
user_codes['us_index_value'] = list(user_codes.index)
small_set = pd.merge(small_set,song_codes,how='left')
small_set = pd.merge(small_set,user_codes,how='left')
mat_candidate = small_set[['us_index_value','so_index_value','fractional_play_count']]
data_array = mat_candidate.fractional_play_count.values
row_array = mat_candidate.us_index_value.values
col_array = mat_candidate.so_index_value.values
data_sparse = coo_matrix((data_array, (row_array, col_array)),dtype=float)
K=50
urm = data_sparse
MAX_PID = urm.shape[1]
MAX_UID = urm.shape[0]
recommender = SVDRecommender(K) # 创建SVD推荐器
U, S, Vt = recommender.fit(urm) # 训练推荐器
uTest = [1,6,7,8,23]
uTest_recommended_items = recommender.recommend(uTest, urm, 10)
# 输出推荐结果
recommendations = pd.DataFrame(columns=['user','song', 'score','rank'])
for user in uTest:
rank = 1
for song_index in uTest_recommended_items[user, 0:10]:
song = small_set.loc[small_set['so_index_value'] == song_index].iloc[0]
recommendations = recommendations.append({'user': user, 'song': song['song'], 'score': song['fractional_play_count'], 'rank': rank}, ignore_index=True)
rank += 1
display(recommendations)
```
相关推荐
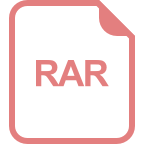













