resnet-34与unet结合的代码
时间: 2024-02-04 15:02:37 浏览: 114
这里是一个简单的ResNet-34与U-Net结合的代码示例,用于图像分割任务:
``` python
import torch
import torch.nn as nn
import torch.nn.functional as F
class ResNetBlock(nn.Module):
def __init__(self, in_channels, out_channels):
super(ResNetBlock, self).__init__()
self.conv1 = nn.Conv2d(in_channels, out_channels, kernel_size=3, padding=1, bias=False)
self.bn1 = nn.BatchNorm2d(out_channels)
self.conv2 = nn.Conv2d(out_channels, out_channels, kernel_size=3, padding=1, bias=False)
self.bn2 = nn.BatchNorm2d(out_channels)
self.relu = nn.ReLU(inplace=True)
def forward(self, x):
identity = x
out = self.conv1(x)
out = self.bn1(out)
out = self.relu(out)
out = self.conv2(out)
out = self.bn2(out)
out += identity
out = self.relu(out)
return out
class ResNetEncoder(nn.Module):
def __init__(self, in_channels, out_channels):
super(ResNetEncoder, self).__init__()
self.conv1 = nn.Conv2d(in_channels, out_channels, kernel_size=7, stride=2, padding=3, bias=False)
self.bn1 = nn.BatchNorm2d(out_channels)
self.relu = nn.ReLU(inplace=True)
self.maxpool = nn.MaxPool2d(kernel_size=3, stride=2, padding=1)
self.layer1 = nn.Sequential(
ResNetBlock(out_channels, out_channels),
ResNetBlock(out_channels, out_channels),
ResNetBlock(out_channels, out_channels),
)
self.layer2 = nn.Sequential(
ResNetBlock(out_channels, out_channels*2),
ResNetBlock(out_channels*2, out_channels*2),
ResNetBlock(out_channels*2, out_channels*2),
)
self.layer3 = nn.Sequential(
ResNetBlock(out_channels*2, out_channels*4),
ResNetBlock(out_channels*4, out_channels*4),
ResNetBlock(out_channels*4, out_channels*4),
ResNetBlock(out_channels*4, out_channels*4),
ResNetBlock(out_channels*4, out_channels*4),
ResNetBlock(out_channels*4, out_channels*4),
)
self.layer4 = nn.Sequential(
ResNetBlock(out_channels*4, out_channels*8),
ResNetBlock(out_channels*8, out_channels*8),
ResNetBlock(out_channels*8, out_channels*8),
)
def forward(self, x):
x = self.conv1(x)
x = self.bn1(x)
x = self.relu(x)
x = self.maxpool(x)
x1 = self.layer1(x)
x2 = self.layer2(x1)
x3 = self.layer3(x2)
x4 = self.layer4(x3)
return x1, x2, x3, x4
class ResNetDecoder(nn.Module):
def __init__(self, in_channels, out_channels):
super(ResNetDecoder, self).__init__()
self.layer1 = nn.Sequential(
ResNetBlock(in_channels*8 + out_channels*8, out_channels*4),
ResNetBlock(out_channels*4, out_channels*4),
ResNetBlock(out_channels*4, out_channels*4),
)
self.layer2 = nn.Sequential(
ResNetBlock(in_channels*4 + out_channels*4, out_channels*2),
ResNetBlock(out_channels*2, out_channels*2),
ResNetBlock(out_channels*2, out_channels*2),
)
self.layer3 = nn.Sequential(
ResNetBlock(in_channels*2 + out_channels*2, out_channels),
ResNetBlock(out_channels, out_channels),
ResNetBlock(out_channels, out_channels),
)
self.layer4 = nn.Sequential(
ResNetBlock(in_channels + out_channels, out_channels),
ResNetBlock(out_channels, out_channels),
ResNetBlock(out_channels, out_channels),
)
self.conv = nn.Conv2d(out_channels, 1, kernel_size=1)
def forward(self, x1, x2, x3, x4):
x = F.interpolate(x4, scale_factor=2)
x = torch.cat([x, x3], dim=1)
x = self.layer1(x)
x = F.interpolate(x, scale_factor=2)
x = torch.cat([x, x2], dim=1)
x = self.layer2(x)
x = F.interpolate(x, scale_factor=2)
x = torch.cat([x, x1], dim=1)
x = self.layer3(x)
x = F.interpolate(x, scale_factor=2)
x = self.layer4(x)
x = self.conv(x)
return x
class ResUNet(nn.Module):
def __init__(self, in_channels, out_channels):
super(ResUNet, self).__init__()
self.encoder = ResNetEncoder(in_channels, out_channels)
self.decoder = ResNetDecoder(in_channels, out_channels)
def forward(self, x):
x1, x2, x3, x4 = self.encoder(x)
out = self.decoder(x1, x2, x3, x4)
return out
```
在这个示例中,我们使用了ResNet-34结构作为编码器,并将其与U-Net结构的解码器相结合。该模型接受大小为(in_channels, H, W)的图像作为输入,并输出大小为(1, H, W)的二进制掩模。
阅读全文
相关推荐
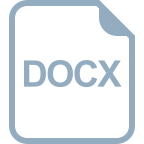
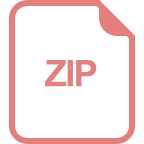
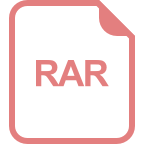

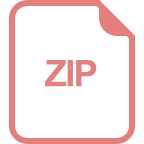
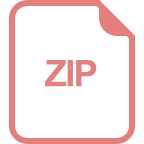
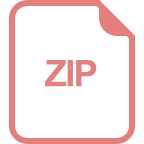
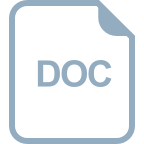
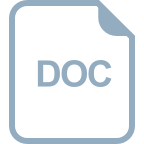
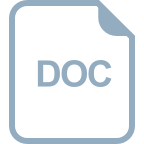
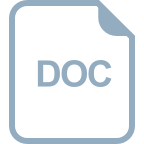
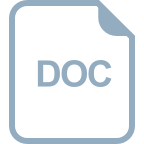
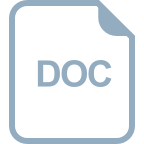
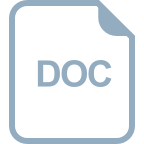
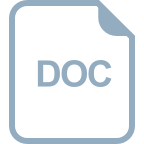
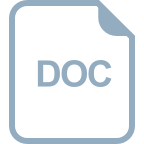
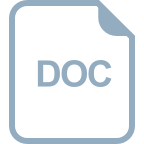
