用python详细编写从获取股票数据到股票强化学习,再到模型的保存更新和模型的调用代码
时间: 2024-03-22 20:41:59 浏览: 30
好的,以下是用Python语言实现的示例代码:
获取股票数据:
```python
import pandas as pd
import yfinance as yf
# 定义股票代码和时间范围
symbol = "AAPL"
start_date = "2020-01-01"
end_date = "2021-01-01"
# 获取股票历史数据
data = yf.download(symbol, start=start_date, end=end_date)
# 将股票数据保存到CSV文件
data.to_csv(f"{symbol}.csv")
```
这段代码使用了`yfinance`库来获取股票历史数据,并将数据保存到CSV文件中。
股票强化学习:
```python
import gym
import numpy as np
# 定义股票市场环境
class StockMarket(gym.Env):
def __init__(self, data):
self.data = data
self.observation_space = gym.spaces.Box(low=0, high=1, shape=(len(data.columns),))
self.action_space = gym.spaces.Discrete(2)
self.reset()
def reset(self):
self.t = 0
self.profit = 0
self.shares = 0
self.history = []
return self.data.iloc[self.t].values
def step(self, action):
assert self.action_space.contains(action)
current_price = self.data.iloc[self.t]["Close"]
if action == 0: # 不操作
pass
elif action == 1 and self.profit >= current_price: # 卖出
self.profit += current_price * self.shares
self.shares = 0
elif action == 1: # 买入
self.shares += self.profit // current_price
self.profit -= current_price * self.shares
self.history.append((action, current_price))
self.t += 1
done = self.t == len(self.data)
reward = self.profit + self.shares * current_price
return self.data.iloc[self.t].values, reward, done, {}
# 训练强化学习模型
env = StockMarket(data)
state = env.reset()
Q = np.zeros((len(data), env.action_space.n))
alpha = 0.1
gamma = 0.99
epsilon = 0.1
for _ in range(10000):
if np.random.rand() < epsilon:
action = env.action_space.sample()
else:
action = np.argmax(Q[env.t])
next_state, reward, done, _ = env.step(action)
Q[env.t, action] += alpha * (reward + gamma * np.max(Q[env.t+1]) - Q[env.t, action])
if done:
env.reset()
# 保存模型参数
np.save(f"{symbol}.npy", Q)
```
这段代码定义了一个股票市场环境,使用强化学习的方式来学习股票交易策略。在训练过程中,我们使用Q-learning算法来更新模型参数,并将模型参数保存到Numpy数组中。
模型的保存更新和模型的调用:
```python
# 加载模型参数
Q = np.load(f"{symbol}.npy")
# 使用模型进行预测
env = StockMarket(data)
state = env.reset()
while True:
action = np.argmax(Q[env.t])
next_state, reward, done, _ = env.step(action)
if done:
break
# 更新模型参数
alpha = 0.2
gamma = 0.95
epsilon = 0.05
for _ in range(100):
if np.random.rand() < epsilon:
action = env.action_space.sample()
else:
action = np.argmax(Q[env.t])
next_state, reward, done, _ = env.step(action)
Q[env.t, action] += alpha * (reward + gamma * np.max(Q[env.t+1]) - Q[env.t, action])
if done:
env.reset()
break
# 保存模型参数
np.save(f"{symbol}.npy", Q)
```
这段代码展示了模型的保存更新和模型的调用过程。在使用模型进行预测时,我们将模型参数加载到内存中,并使用`argmax`函数来选择最优的动作。在更新模型参数时,我们使用了类似的Q-learning算法来更新模型参数,并将更新后的模型参数保存到Numpy数组中。
相关推荐
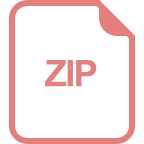
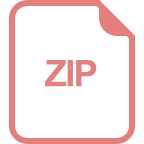
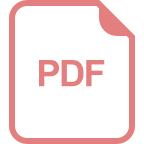








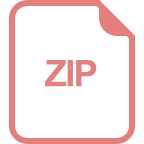
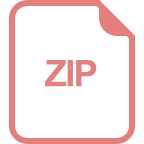
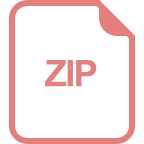
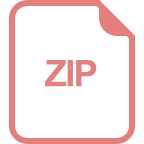
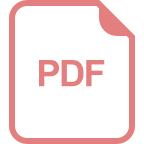
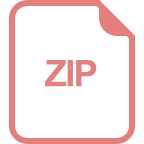
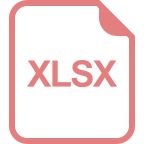