将Unet ++和门结构结合的PyTorch 代码
时间: 2023-05-24 09:07:14 浏览: 194
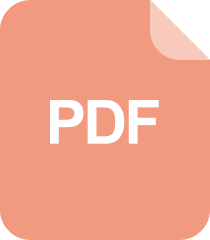
U-net结构图及其Pytorch实现
以下是将Unet和门结构结合的PyTorch代码:
```
import torch
import torch.nn as nn
import torch.nn.functional as F
class ConvBlock(nn.Module):
def __init__(self, in_channels, out_channels):
super(ConvBlock, self).__init__()
self.conv1 = nn.Conv2d(in_channels, out_channels, kernel_size=3, padding=1)
self.bn1 = nn.BatchNorm2d(out_channels)
self.conv2 = nn.Conv2d(out_channels, out_channels, kernel_size=3, padding=1)
self.bn2 = nn.BatchNorm2d(out_channels)
def forward(self, x):
x = self.conv1(x)
x = self.bn1(x)
x = F.relu(x)
x = self.conv2(x)
x = self.bn2(x)
x = F.relu(x)
return x
class UNet(nn.Module):
def __init__(self, in_channels=3, out_channels=1):
super(UNet, self).__init__()
self.down1 = ConvBlock(in_channels, 64)
self.pool1 = nn.MaxPool2d(kernel_size=2, stride=2)
self.down2 = ConvBlock(64, 128)
self.pool2 = nn.MaxPool2d(kernel_size=2, stride=2)
self.down3 = ConvBlock(128, 256)
self.pool3 = nn.MaxPool2d(kernel_size=2, stride=2)
self.down4 = ConvBlock(256, 512)
self.pool4 = nn.MaxPool2d(kernel_size=2, stride=2)
self.bottom = ConvBlock(512, 1024)
self.up4 = nn.ConvTranspose2d(1024, 512, kernel_size=2, stride=2)
self.upconv4 = ConvBlock(1024, 512)
self.up3 = nn.ConvTranspose2d(512, 256, kernel_size=2, stride=2)
self.upconv3 = ConvBlock(512, 256)
self.up2 = nn.ConvTranspose2d(256, 128, kernel_size=2, stride=2)
self.upconv2 = ConvBlock(256, 128)
self.up1 = nn.ConvTranspose2d(128, 64, kernel_size=2, stride=2)
self.upconv1 = ConvBlock(128, 64)
self.outconv = nn.Conv2d(64, out_channels, kernel_size=1)
# 定义门结构的两个分支
self.branch1 = nn.Sequential(
nn.Conv2d(1024, 1024, kernel_size=3, padding=1),
nn.BatchNorm2d(1024),
nn.ReLU(inplace=True),
nn.Conv2d(1024, 1, kernel_size=1)
)
self.branch2 = nn.Sequential(
nn.Conv2d(1024, 1024, kernel_size=3, padding=1),
nn.BatchNorm2d(1024),
nn.ReLU(inplace=True),
nn.Conv2d(1024, 1, kernel_size=1)
)
def forward(self, x):
# 下采样
x1 = self.down1(x)
x2 = self.pool1(x1)
x3 = self.down2(x2)
x4 = self.pool2(x3)
x5 = self.down3(x4)
x6 = self.pool3(x5)
x7 = self.down4(x6)
x8 = self.pool4(x7)
# 中间部分
x9 = self.bottom(x8)
# 上采样
x10 = self.up4(x9)
x10 = torch.cat([x10, x7], dim=1)
x11 = self.upconv4(x10)
x12 = self.up3(x11)
x12 = torch.cat([x12, x5], dim=1)
x13 = self.upconv3(x12)
x14 = self.up2(x13)
x14 = torch.cat([x14, x3], dim=1)
x15 = self.upconv2(x14)
x16 = self.up1(x15)
x16 = torch.cat([x16, x1], dim=1)
x17 = self.upconv1(x16)
# 输出结果
x18 = self.outconv(x17)
# 计算门控信号
branch1 = self.branch1(x9)
branch2 = self.branch2(x9)
gate = F.sigmoid(branch2)
x18 = gate*x18 + (1-gate)*branch1
return x18
```
上述代码定义了一个Unet模型和一个门结构模块,并将它们结合起来。具体来说,Unet模型由一系列卷积块和反卷积块组成,使用了下采样和上采样操作实现了图像分割。门结构模块由两个分支组成,它们分别计算了输入特征图的重要程度,用sigmoid函数将它们结合起来得到一个门控信号,然后将这个信号应用于Unet模型输出的结果和门结构模块的输出结果来获得最终的输出。
阅读全文
相关推荐
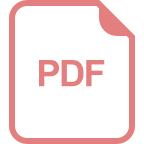
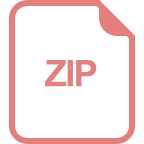
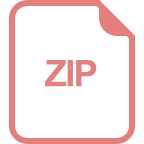
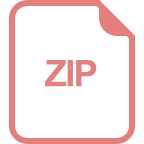
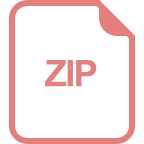
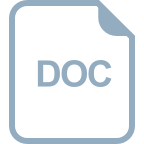
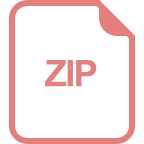
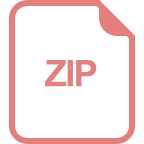
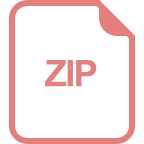
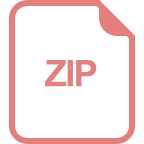
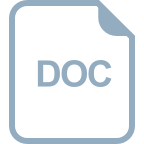
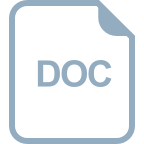
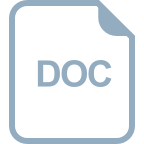
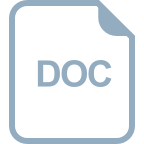
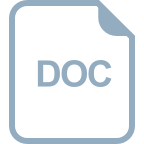
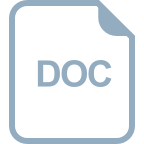
