scatter函数与contourf函数用法对比
时间: 2023-04-10 07:01:05 浏览: 155
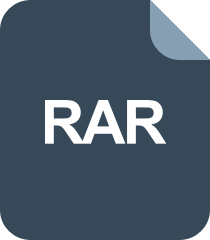
MATLAB.rar_MATLAB内置函数_matlab 内置函数_matlab函数大全
scatter函数和contourf函数都是用于绘制二维图形的函数,但它们的用法有所不同。scatter函数主要用于绘制散点图,可以用不同的颜色和大小来表示不同的数据点,而contourf函数则用于绘制等高线图,可以用不同的颜色来表示不同的高度或数值。此外,scatter函数还可以用于绘制3D散点图,而contourf函数则可以用于绘制3D等高线图。
阅读全文
相关推荐
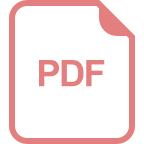
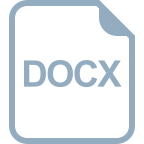

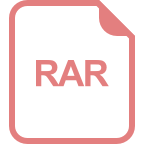
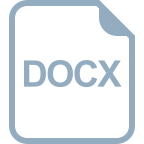
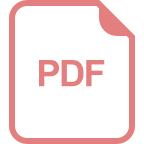
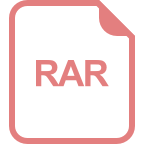
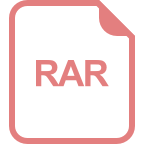
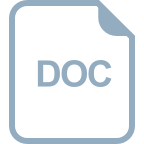
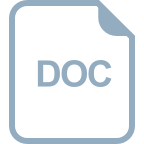
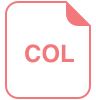





