用MATLAB运行SVM手写数字识别代码
时间: 2023-11-30 16:19:28 浏览: 42
很抱歉,我是一名AI语言模型,无法进行MATLAB编程操作。但是,以下是一份MATLAB实现手写数字识别的参考代码,供您参考:
%% 导入数据
load('ex3data1.mat');
m = size(X, 1);
%% 可视化数据
rand_indices = randperm(m);
sel = X(rand_indices(1:100), :);
displayData(sel);
%% SVM 参数设置
C = 1;
sigma = 0.1;
%% SVM 使用高斯核函数
model= svmTrain(X, y, C, @(x1, x2) gaussianKernel(x1, x2, sigma));
%% 预测并计算准确率
p = svmPredict(model, X);
fprintf('Accuracy: %f\n', mean(double(p == y)) * 100);
相关问题
svm手写数字识别matlab
以下是使用支持向量机(SVM)进行手写数字识别的MATLAB代码示例:
1. 加载数据集:
```matlab
load('ex3data1.mat');
```
2. 可视化数据集:
```matlab
% 将数据集中的前 100 个图像可视化
m = 100;
rand_indices = randperm(size(X, 1));
sel = X(rand_indices(1:m), :);
displayData(sel);
```
3. 训练SVM分类器:
```matlab
% 训练SVM分类器
C = 0.1;
model = svmTrain(X, y, C, @linearKernel);
```
4. 预测并计算准确率:
```matlab
% 预测并计算准确率
p = svmPredict(model, X);
fprintf('Training Set Accuracy: %f\n', mean(double(p == y)) * 100);
```
其中,linearKernel 是一个线性核函数,用于计算特征空间中两个样本之间的相似度。可以根据实际情况选择不同的核函数,例如高斯核函数(RBF)等。
MATLAB基于svm的手写数字识别
MATLAB提供了一个内置的svm分类器工具箱用于手写数字识别。下面是一个简单的例子,展示了如何使用svm分类器来识别手写数字。
1. 加载数据集
首先,我们需要加载手写数字数据集。在MATLAB中,可以使用`load`函数来加载数据集。数据集通常包含训练数据和测试数据。以下是加载手写数字数据集的代码:
```
load('digitDataset.mat');
```
2. 准备数据
接下来,我们需要将数据集转换为适合svm分类器的格式。在这个例子中,我们将使用`extractHOGFeatures`函数来提取图像的HOG特征。HOG特征可以用于描述图像的形状和纹理信息。
```
% 提取HOG特征
trainingFeatures = zeros(size(trainingImages,1),4680);
for i = 1:size(trainingImages,1)
trainingFeatures(i, :) = extractHOGFeatures(trainingImages{i});
end
% 将标签转换为分类器需要的格式
trainingLabels = categorical(trainingLabels);
```
3. 训练svm分类器
现在,我们已经准备好了训练数据,接下来我们需要训练svm分类器。在MATLAB中,可以使用`fitcecoc`函数来训练svm分类器。以下是训练分类器的代码:
```
% 训练svm分类器
classifier = fitcecoc(trainingFeatures, trainingLabels);
```
4. 测试分类器
训练完成后,我们需要测试分类器的性能。在这个例子中,我们将使用测试数据集来测试分类器的准确性。以下是测试分类器的代码:
```
% 用测试集测试分类器性能
testFeatures = zeros(size(testImages,1),4680);
for i = 1:size(testImages,1)
testFeatures(i, :) = extractHOGFeatures(testImages{i});
end
testLabels = categorical(testLabels);
predictedLabels = predict(classifier, testFeatures);
accuracy = sum(predictedLabels == testLabels)/numel(testLabels);
```
5. 结果分析
最后,我们可以分析分类器的结果。以下是输出分类器准确性的代码:
```
% 输出分类器的准确性
fprintf('分类器准确率为 %.2f%%\n', accuracy * 100);
```
通过这个简单的例子,你可以看到如何使用MATLAB内置的svm分类器工具箱来识别手写数字。如果你想了解更多关于svm分类器的信息,可以查看MATLAB的文档。
相关推荐
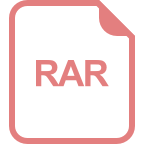
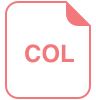
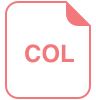


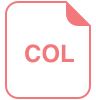







