为以下代码写注释:class TransformerClassifier(torch.nn.Module): def __init__(self, num_labels): super().__init__() self.bert = BertForSequenceClassification.from_pretrained('bert-base-chinese', num_labels=num_labels) # print(self.bert.config.hidden_size) #768 self.dropout = torch.nn.Dropout(0.1) self.classifier1 = torch.nn.Linear(640, 256) self.classifier2 = torch.nn.Linear(256, num_labels) self.regress1 = torch.nn.Linear(640, 256) self.regress2 = torch.nn.Linear(256, 2) self.regress3 = torch.nn.Linear(640, 256) self.regress4 = torch.nn.Linear(256, 2) # self.regress3 = torch.nn.Linear(64, 1) # self.regress3 = torch.nn.Linear(640, 256) # self.regress4 = torch.nn.Linear(256, 1) # self.soft1 = torch.nn.Softmax(dim=1) def forward(self, input_ids, attention_mask, token_type_ids): # outputs = self.bert(input_ids, attention_mask=attention_mask, token_type_ids=token_type_ids) # pooled_output = outputs.logits # # pooled_output = self.dropout(pooled_output) # # logits = self.classifier(pooled_output) outputs = self.bert(input_ids, attention_mask=attention_mask, token_type_ids=token_type_ids) logits = outputs.logits clas = F.relu(self.classifier1(logits)) clas = self.classifier2(clas) death = F.relu(self.regress1(logits)) # xingqi = F.relu(self.regress2(xingqi)) death = self.regress2(death) life = F.relu(self.regress3(logits)) # xingqi = F.relu(self.regress2(xingqi)) life = self.regress4(life) # fakuan = F.relu(self.regress3(logits)) # fakuan = self.regress4(fakuan) # print(logits.shape) # logits = self.soft1(logits) # print(logits) # print(logits.shape) return clas,death,life
时间: 2023-12-12 18:02:24 浏览: 174
注释如下:
```
class TransformerClassifier(torch.nn.Module): # 定义一个名为TransformerClassifier的继承自nn.Module的类
def __init__(self, num_labels): # 初始化函数,num_labels为标签数
super().__init__() # 调用父类的构造函数
self.bert = BertForSequenceClassification.from_pretrained('bert-base-chinese', num_labels=num_labels) # 用预训练的中文Bert模型初始化self.bert, 输出维度为num_labels
# print(self.bert.config.hidden_size) #768
self.dropout = torch.nn.Dropout(0.1) # 定义dropout层,丢弃概率为0.1
self.classifier1 = torch.nn.Linear(640, 256) # 定义一个全连接层,输入维度为640,输出维度为256
self.classifier2 = torch.nn.Linear(256, num_labels) # 定义一个全连接层,输入维度为256,输出维度为num_labels
self.regress1 = torch.nn.Linear(640, 256) # 定义一个全连接层,输入维度为640,输出维度为256
self.regress2 = torch.nn.Linear(256, 2) # 定义一个全连接层,输入维度为256,输出维度为2
self.regress3 = torch.nn.Linear(640, 256) # 定义一个全连接层,输入维度为640,输出维度为256
self.regress4 = torch.nn.Linear(256, 2) # 定义一个全连接层,输入维度为256,输出维度为2
def forward(self, input_ids, attention_mask, token_type_ids): # 前向传播函数,输入参数分别为input_ids、attention_mask、token_type_ids
outputs = self.bert(input_ids, attention_mask=attention_mask, token_type_ids=token_type_ids) # 将输入传入self.bert中,得到输出
logits = outputs.logits # 从输出中得到logits
clas = F.relu(self.classifier1(logits)) # 将logits输入到self.classifier1中,经过relu函数后得到clas
clas = self.classifier2(clas) # 将clas输入到self.classifier2中,得到分类结果
death = F.relu(self.regress1(logits)) # 将logits输入到self.regress1中,经过relu函数后得到death
death = self.regress2(death) # 将death输入到self.regress2中,得到死亡概率
life = F.relu(self.regress3(logits)) # 将logits输入到self.regress3中,经过relu函数后得到life
life = self.regress4(life) # 将life输入到self.regress4中,得到生存概率
return clas, death, life # 返回分类结果、死亡概率、生存概率
阅读全文
相关推荐
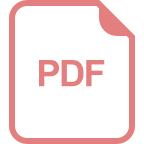
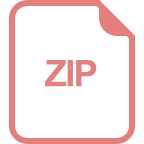
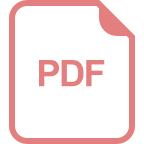
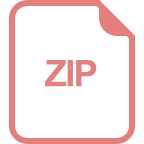
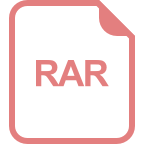













