使用bert将txt文件分类的代码
时间: 2023-08-06 16:09:33 浏览: 42
下面是使用BERT模型进行文本分类的代码示例。其中,我们使用了Hugging Face的transformers库,该库提供了方便的BERT预训练模型和文本分类任务的接口。
```python
import torch
from torch.utils.data import TensorDataset, DataLoader, RandomSampler, SequentialSampler
from transformers import BertTokenizer, BertForSequenceClassification, AdamW, get_linear_schedule_with_warmup
import numpy as np
from sklearn.metrics import f1_score, accuracy_score
# 定义一些超参数
MAX_LEN = 128
BATCH_SIZE = 32
LEARNING_RATE = 2e-5
NUM_EPOCHS = 3
EPSILON = 1e-8
# 加载数据
with open('train.txt', 'r', encoding='utf-8') as f:
train_data = f.readlines()
with open('test.txt', 'r', encoding='utf-8') as f:
test_data = f.readlines()
# 分离文本和标签
train_texts = [line.split('\t')[0] for line in train_data]
train_labels = [int(line.split('\t')[1].strip()) for line in train_data]
test_texts = [line.split('\t')[0] for line in test_data]
test_labels = [int(line.split('\t')[1].strip()) for line in test_data]
# 加载BERT分词器
tokenizer = BertTokenizer.from_pretrained('bert-base-chinese', do_lower_case=True)
# 对训练集和测试集进行分词、编码和填充
train_encodings = tokenizer.batch_encode_plus(train_texts, truncation=True, padding=True, max_length=MAX_LEN)
test_encodings = tokenizer.batch_encode_plus(test_texts, truncation=True, padding=True, max_length=MAX_LEN)
train_input_ids = train_encodings['input_ids']
train_attention_masks = train_encodings['attention_mask']
train_labels = torch.tensor(train_labels)
test_input_ids = test_encodings['input_ids']
test_attention_masks = test_encodings['attention_mask']
test_labels = torch.tensor(test_labels)
# 创建数据集和数据加载器
train_dataset = TensorDataset(train_input_ids, train_attention_masks, train_labels)
train_sampler = RandomSampler(train_dataset)
train_dataloader = DataLoader(train_dataset, sampler=train_sampler, batch_size=BATCH_SIZE)
test_dataset = TensorDataset(test_input_ids, test_attention_masks, test_labels)
test_sampler = SequentialSampler(test_dataset)
test_dataloader = DataLoader(test_dataset, sampler=test_sampler, batch_size=BATCH_SIZE)
# 加载BERT模型
model = BertForSequenceClassification.from_pretrained('bert-base-chinese', num_labels=2)
model.cuda()
# 定义优化器和学习率调度器
optimizer = AdamW(model.parameters(), lr=LEARNING_RATE, eps=EPSILON)
total_steps = len(train_dataloader) * NUM_EPOCHS
scheduler = get_linear_schedule_with_warmup(optimizer, num_warmup_steps=0, num_training_steps=total_steps)
# 训练模型
for epoch in range(NUM_EPOCHS):
print('Epoch:', epoch + 1)
model.train()
total_loss = 0
for step, batch in enumerate(train_dataloader):
batch_input_ids = batch[0].cuda()
batch_attention_masks = batch[1].cuda()
batch_labels = batch[2].cuda()
optimizer.zero_grad()
outputs = model(batch_input_ids, attention_mask=batch_attention_masks, labels=batch_labels)
loss = outputs[0]
total_loss += loss.item()
loss.backward()
torch.nn.utils.clip_grad_norm_(model.parameters(), 1.0)
optimizer.step()
scheduler.step()
avg_train_loss = total_loss / len(train_dataloader)
print('Average training loss:', avg_train_loss)
# 在验证集上评估模型
model.eval()
preds = []
true_labels = []
for batch in test_dataloader:
batch_input_ids = batch[0].cuda()
batch_attention_masks = batch[1].cuda()
batch_labels = batch[2].numpy()
with torch.no_grad():
outputs = model(batch_input_ids, attention_mask=batch_attention_masks)
logits = outputs[0]
batch_preds = np.argmax(logits.detach().cpu().numpy(), axis=1)
preds.extend(batch_preds)
true_labels.extend(batch_labels)
f1 = f1_score(true_labels, preds)
acc = accuracy_score(true_labels, preds)
print('F1 score:', f1)
print('Accuracy:', acc)
```
在这个示例中,我们使用了一个包含两个类别的文本分类任务作为演示。你可以根据自己的需要修改代码中的变量和超参数。
相关推荐
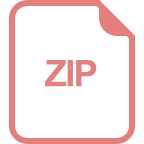
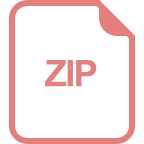
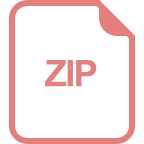









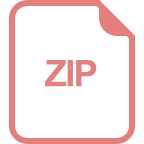
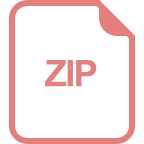
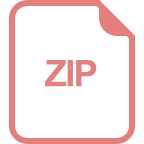
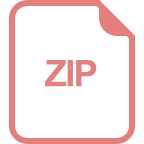
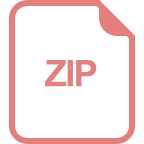
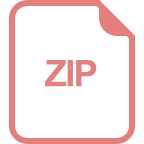