Derivative函数在python中的用法
时间: 2023-06-22 22:17:45 浏览: 97
在Python中,可以使用Sympy库来进行符号计算,包括求导操作。Sympy中的Derivative函数可以用来表示函数的导数,使用方法如下:
首先,需要导入Sympy库和符号变量x:
```python
import sympy as sp
x = sp.Symbol('x')
```
然后,可以使用Derivative函数来表示函数的导数,例如:
```python
f = sp.Function('f')(x)
df = sp.Derivative(f, x)
```
其中,f表示原函数,df表示f对x的导数。
最后,可以使用doit()方法对Derivative对象进行求导:
```python
df.doit()
```
这将返回f对x的一阶导数。如果需要求高阶导数,可以在Derivative函数中指定求导的次数,例如:
```python
d2f = sp.Derivative(f, x, 2)
d2f.doit()
```
这将返回f对x的二阶导数。
相关问题
一个bp神经网络的测试函数用python
一个BP神经网络的测试函数可以用Python编写,如下所示:
```python
import numpy as np
def sigmoid(x):
return 1 / (1 + np.exp(-x))
def sigmoid_derivative(x):
return x * (1 - x)
def train(inputs, outputs, epochs, learning_rate):
input_layer_size = inputs.shape
hidden_layer_size = 4
output_layer_size = 1
weights_hidden = np.random.uniform(size=(input_layer_size, hidden_layer_size))
weights_output = np.random.uniform(size=(hidden_layer_size, output_layer_size))
for epoch in range(epochs):
# Forward pass
hidden_layer_input = np.dot(inputs, weights_hidden)
hidden_layer_output = sigmoid(hidden_layer_input)
output_layer_input = np.dot(hidden_layer_output, weights_output)
output_layer_output = sigmoid(output_layer_input)
# Backward pass
output_error = outputs - output_layer_output
output_delta = output_error * sigmoid_derivative(output_layer_output)
hidden_error = np.dot(output_delta, weights_output.T)
hidden_delta = hidden_error * sigmoid_derivative(hidden_layer_output)
# Update weights
weights_output += np.dot(hidden_layer_output.T, output_delta) * learning_rate
weights_hidden += np.dot(inputs.T, hidden_delta) * learning_rate
return weights_hidden, weights_output
def predict(inputs, weights_hidden, weights_output):
hidden_layer_input = np.dot(inputs, weights_hidden)
hidden_layer_output = sigmoid(hidden_layer_input)
output_layer_input = np.dot(hidden_layer_output, weights_output)
output_layer_output = sigmoid(output_layer_input)
return output_layer_output
# Example usage:
inputs = np.array([[0, 0], [0, 1], [1, 0], [1, 1]])
outputs = np.array([, , , ])
weights_hidden, weights_output = train(inputs, outputs, epochs=10000, learning_rate=0.1)
predictions = predict(inputs, weights_hidden, weights_output)
print(predictions)
```
这个测试函数包含了一个简单的BP神经网络的训练和预测过程。首先定义了激活函数(sigmoid)及其导数,然后实现了训练函数(train)和预测函数(predict)。在示例用法中,我们创建了一个简单的异或(XOR)问题的输入和输出数据,并使用训练好的神经网络进行预测。
Python中实现自适应PID算法
在Python中实现自适应PID算法可以使用一些现有的库,如`python-control`或`scipy`。这些库提供了一些功能强大的工具和函数来支持PID控制算法的实现。下面是一个使用`python-control`库实现自适应PID算法的示例:
```python
import control
import numpy as np
class AdaptivePID:
def __init__(self, Kp, Ki, Kd):
self.Kp = Kp
self.Ki = Ki
self.Kd = Kd
self.integral = 0.0
self.last_error = 0.0
self.last_output = 0.0
def update(self, setpoint, feedback):
error = setpoint - feedback
self.integral += error
derivative = error - self.last_error
# 定义自适应参数的更新规则
self.Kp += self.Kp * 0.01 * error
self.Ki += self.Ki * 0.01 * self.integral
self.Kd += self.Kd * 0.01 * derivative
output = self.Kp * error + self.Ki * self.integral + self.Kd * derivative
self.last_error = error
self.last_output = output
return output
# 使用示例
pid = AdaptivePID(0.5, 0.1, 0.2)
setpoint = 10.0
feedback = 0.0
while True:
output = pid.update(setpoint, feedback)
# 将output应用于控制系统中
# 获取新的feedback值
```
这个示例中使用了一个简单的自适应规则来更新PID参数,即在每次控制循环中,通过乘以一个小的学习率(0.01)将误差、积分项和微分项的大小调整反馈给PID参数,从而实现自适应。
请注意,这只是一个简单的示例,实际的自适应PID算法可能需要更复杂的规则和策略来根据系统响应调整参数。具体的方案需要根据实际问题和需求进行设计和调整。
相关推荐
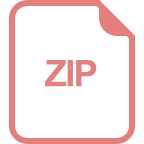
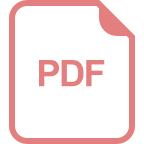
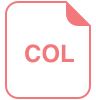
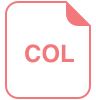
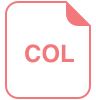
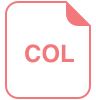
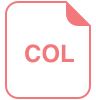







