以下代码似乎出现了死循环,导致运行了30min也没有结果,请进行修改:from sklearn.svm import SVC from sklearn.ensemble import BaggingClassifier from sklearn.model_selection import GridSearchCV from sklearn.preprocessing import StandardScaler # 数据预处理 sc = StandardScaler() X_std = sc.fit_transform(X) # 定义模型 svc = SVC() bagging = BaggingClassifier(svc) # 定义参数空间 param_grid = {'n_estimators': [10, 50, 100, 200, 500], 'base_estimator__kernel': ['linear', 'poly', 'rbf', 'sigmoid'], 'base_estimator__C': [0.1, 1, 10, 100], 'base_estimator__gamma': ['scale', 'auto', 0.1, 1, 10, 100]} # 定义网格搜索对象 clf = GridSearchCV(bagging, param_grid=param_grid, cv=5) # 训练模型 clf.fit(X_std, y) # 输出最优参数 print("Best parameters:", clf.best_params_)
时间: 2024-01-21 17:03:44 浏览: 78
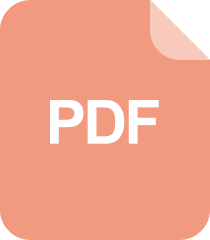
sklearn.svm.SVC 参数说明 - 学习、思考、总结 - 博客频道 - CSDN1
可以尝试使用以下方法对代码进行修改,避免死循环的出现:
1. 增加 GridSearchCV() 函数中的 n_jobs 参数,使用多线程运行网格搜索,以提高计算效率。例如将 n_jobs 设置为 -1,表示使用所有可用的 CPU 核心来运行网格搜索。
2. 减少参数空间的大小,以减少计算量。可以根据经验或者先前的研究结果,选择一些较为重要的参数进行调整,而将其他参数设置为默认值。
3. 修改 BaggingClassifier() 函数中的参数,例如将 n_estimators 设置为较小的值,以减少计算时间。
修改后的代码如下:
```
from sklearn.svm import SVC
from sklearn.ensemble import BaggingClassifier
from sklearn.model_selection import GridSearchCV
from sklearn.preprocessing import StandardScaler
# 数据预处理
sc = StandardScaler()
X_std = sc.fit_transform(X)
# 定义模型
svc = SVC()
bagging = BaggingClassifier(svc, n_estimators=10, max_samples=0.5, max_features=0.5)
# 定义参数空间
param_grid = {'base_estimator__kernel': ['linear', 'rbf'],
'base_estimator__C': [0.1, 1, 10],
'base_estimator__gamma': ['scale', 'auto'],
'n_estimators': [10, 50, 100]}
# 定义网格搜索对象
clf = GridSearchCV(bagging, param_grid=param_grid, cv=5, n_jobs=-1)
# 训练模型
clf.fit(X_std, y)
# 输出最优参数
print("Best parameters:", clf.best_params_)
```
这里修改了 BaggingClassifier() 函数的参数,将 n_estimators 设置为 10,max_samples 和 max_features 设置为 0.5,以减少计算量。同时,减小了参数空间,只调整了 base_estimator__kernel、base_estimator__C、base_estimator__gamma 和 n_estimators 四个参数。最后,使用 n_jobs=-1 参数来加快网格搜索。
阅读全文
相关推荐
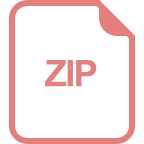
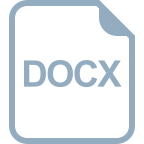








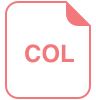


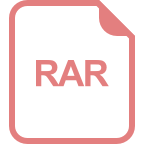
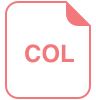
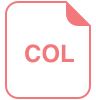

