Using Hidden Markov Models for the accurate linguistic analysis of process model activity labels
时间: 2024-05-23 10:10:48 浏览: 20
Sure, I can provide some information on using Hidden Markov Models (HMMs) for linguistic analysis of process model activity labels. HMMs are a type of statistical model commonly used in natural language processing (NLP) to analyze sequences of words or tokens. In the context of process models, activity labels are typically short phrases or sentences that describe the actions or steps involved in a particular process.
One application of HMMs in this context is to identify the underlying grammatical structure of activity labels, such as verb phrases, noun phrases, and prepositional phrases. This can be done by training the HMM on a large corpus of labeled activity labels, where each label is annotated with its grammatical structure. The HMM can then be used to automatically assign grammatical labels to new activity labels based on their similarity to the training data.
Another application of HMMs is to identify patterns or regularities in the way activity labels are used within a process model. For example, an HMM could be trained to recognize common sequences of activity labels that tend to occur together within a particular type of process. This could be used to identify best practices or common pitfalls in process design, or to automatically generate new process models based on existing patterns.
Overall, HMMs are a powerful tool for linguistic analysis of process model activity labels, and can help to improve the accuracy and efficiency of process modeling and analysis.
相关推荐
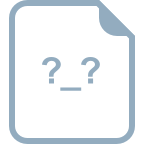












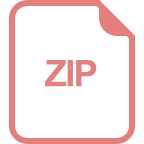