给出做nlp基于pytorch的RNN代码
时间: 2023-09-17 13:11:26 浏览: 50
以下是一个基于PyTorch实现的RNN模型的示例代码:
```python
import torch
import torch.nn as nn
class RNN(nn.Module):
def __init__(self, input_size, hidden_size, output_size):
super(RNN, self).__init__()
self.hidden_size = hidden_size
self.i2h = nn.Linear(input_size + hidden_size, hidden_size)
self.i2o = nn.Linear(input_size + hidden_size, output_size)
self.softmax = nn.LogSoftmax(dim=1)
def forward(self, input, hidden):
combined = torch.cat((input, hidden), 1)
hidden = self.i2h(combined)
output = self.i2o(combined)
output = self.softmax(output)
return output, hidden
def initHidden(self):
return torch.zeros(1, self.hidden_size)
```
该模型使用一个单向RNN层来对输入进行处理,其中`input_size`表示输入向量的大小,`hidden_size`表示隐藏层的大小,`output_size`表示输出向量的大小。在模型的构造函数中,我们使用PyTorch内置的`nn.Linear`函数定义了两个全连接层`i2h`和`i2o`,以及一个`nn.LogSoftmax`层,用于对输出进行归一化处理。
在`forward`函数中,我们首先将输入和隐藏层的状态拼接在一起,然后通过`i2h`和`i2o`层分别计算得到新的隐藏状态和输出向量。最后,我们使用`nn.LogSoftmax`层对输出向量进行归一化处理,并返回输出向量和新的隐藏状态。
在训练过程中,我们可以使用该模型来对序列数据进行处理,并在每个时间步上计算损失函数。同时,我们可以使用PyTorch内置的优化器对模型进行反向传播和更新。
相关推荐
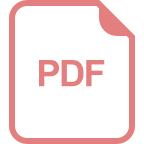
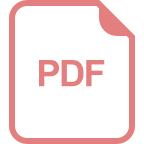
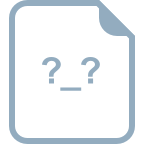














