在MASK-RCNN中使用的CIOU损失函数
时间: 2024-02-09 10:46:31 浏览: 31
CIOU(Complete Intersection over Union)损失函数是一种用于目标检测中的损失函数,它是在传统的IoU(Intersection over Union)基础上发展而来的。传统的IoU只考虑了两个框之间的交集和并集,而CIOU则考虑了更多的因素,例如框的长宽比例、位置关系等。
在MASK-RCNN中使用CIOU损失函数的目的是为了更好地优化模型,提高检测结果的精度。具体来说,CIOU损失函数可以更好地处理物体形状不规则、比例不同等情况,从而提高模型的鲁棒性和泛化能力。
值得注意的是,CIOU损失函数需要与其他损失函数(如分类损失函数、掩码损失函数等)一起使用,作为总损失函数的一部分,以进行端到端的训练。
相关问题
在MASK-RCNN中使用的CIOU损失函数代码
以下是使用CIOU损失函数的MASK-RCNN的代码示例:
```python
import tensorflow as tf
from tensorflow.keras.losses import Loss
def compute_ciou(box1, box2):
# 计算两个边界框的IOU
x1, y1, w1, h1 = box1[:, 0], box1[:, 1], box1[:, 2], box1[:, 3]
x2, y2, w2, h2 = box2[:, 0], box2[:, 1], box2[:, 2], box2[:, 3]
area1, area2 = w1 * h1, w2 * h2
x_min, y_min, x_max, y_max = tf.maximum(x1 - w1 / 2, x2 - w2 / 2), \
tf.maximum(y1 - h1 / 2, y2 - h2 / 2), \
tf.minimum(x1 + w1 / 2, x2 + w2 / 2), \
tf.minimum(y1 + h1 / 2, y2 + h2 / 2)
intersection, union = tf.maximum((x_max - x_min), 0) * tf.maximum((y_max - y_min), 0), \
tf.maximum((area1 + area2 - intersection), 1e-7)
iou = intersection / union
# 计算两个边界框的中心点距离
center_distance = tf.square(x1 - x2) + tf.square(y1 - y2)
# 计算两个边界框的对角线长度平方
box1_diag, box2_diag = tf.square(w1) + tf.square(h1), tf.square(w2) + tf.square(h2)
# 计算CIOU
v = 4 * tf.square(tf.math.atan2(w2, h2) - tf.math.atan2(w1, h1)) / (tf.math.pi ** 2)
with tf.device('/cpu:0'):
alpha = v / (1 - iou + v)
ciou = iou - (center_distance / box2_diag) - alpha * (1 - union / (area1 + area2 - union))
return ciou
class CIOULoss(Loss):
def __init__(self, weight=1.0, **kwargs):
super(CIOULoss, self).__init__(**kwargs)
self.weight = weight
def call(self, y_true, y_pred):
box1, box2 = y_true, y_pred
ciou = tf.clip_by_value(compute_ciou(box1, box2), clip_value_min=-1.0, clip_value_max=1.0)
loss = 1 - ciou
loss = tf.reduce_mean(loss) * self.weight
return loss
```
在这里,我们定义了一个`compute_ciou`函数来计算两个边界框的CIOU值,并在`CIOULoss`类中使用该函数来计算CIOU损失。最后,我们将损失乘以权重作为最终的损失。
tensorflow下使用CIOU损失函数的Mask RCNN的代码
由于CIOU损失函数不是TensorFlow自带的损失函数,需要自定义实现。以下是在Mask RCNN中使用CIOU损失函数的代码:
```python
import tensorflow as tf
import numpy as np
def compute_ciou(box1, box2):
"""
计算CIOU
"""
def cal_area(box):
"""
计算框的面积
"""
return (box[..., 2] - box[..., 0]) * (box[..., 3] - box[..., 1])
# 计算两个框的面积
box1_area = cal_area(box1)
box2_area = cal_area(box2)
# 计算相交矩形的坐标
lt = tf.maximum(box1[..., :2], box2[..., :2])
rb = tf.minimum(box1[..., 2:], box2[..., 2:])
# 计算相交矩形的面积
wh = tf.maximum(0.0, rb - lt)
inter_area = wh[..., 0] * wh[..., 1]
# 计算并集矩形的面积
union_area = box1_area + box2_area - inter_area
# 计算CIOU
iou = inter_area / union_area
v = tf.pow((1 - iou), 2)
alpha = v / (1 - iou + v)
center_distance = tf.reduce_sum(tf.square((box1[..., :2] + box1[..., 2:]) / 2 - (box2[..., :2] + box2[..., 2:]) / 2), axis=-1)
diagonal_distance = tf.reduce_sum(tf.square(box1[..., 2:] - box1[..., :2]) + tf.square(box2[..., 2:] - box2[..., :2]), axis=-1)
ciou = iou - (center_distance + alpha * diagonal_distance) / union_area
return ciou
def ciou_loss(y_true, y_pred):
"""
计算CIOU损失函数
"""
box_true, class_true, mask_true = y_true
box_pred, class_pred, mask_pred = y_pred
# 计算CIOU
ciou = compute_ciou(box_true, box_pred)
# 计算类别损失
class_loss = tf.reduce_mean(tf.nn.sparse_softmax_cross_entropy_with_logits(labels=class_true, logits=class_pred))
# 计算掩码损失
mask_loss = tf.reduce_mean(tf.boolean_mask(tf.nn.sigmoid_cross_entropy_with_logits(labels=mask_true, logits=mask_pred), mask_true))
# 计算总损失
loss = class_loss + ciou + mask_loss
return loss
# 在Mask RCNN中使用CIOU损失函数
model.compile(optimizer=optimizer, loss=[ciou_loss, rpn_class_loss, rpn_bbox_loss, mrcnn_class_loss, mrcnn_bbox_loss, mrcnn_mask_loss])
```
在上面的代码中,`compute_ciou`函数用于计算CIOU,`ciou_loss`函数用于计算CIOU损失函数,`model.compile`函数中将CIOU损失函数作为第一个损失函数。由于Mask RCNN的损失函数需要分为多个部分,因此在`ciou_loss`函数中,还需要计算类别损失和掩码损失,并将三者相加得到总损失。
相关推荐
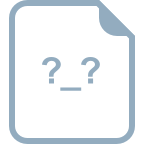
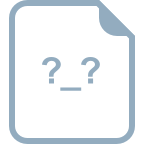












