bi-lstm的Python代码
时间: 2023-03-14 07:33:18 浏览: 129
Bi-LSTM(双向长期短期记忆)是一种深度学习模型,它可以捕捉序列数据中的长期依赖关系。下面是一段Python代码,用来实现Bi-LSTM:model = Sequential()
model.add(Bidirectional(LSTM(128, return_sequences=True), input_shape=(max_len, embedding_dim)))
model.add(Bidirectional(LSTM(128)))
model.add(Dense(64, activation='relu'))
model.add(Dense(1, activation='sigmoid'))
model.compile(loss='binary_crossentropy', optimizer='adam', metrics=['accuracy'])
相关问题
bi-lstm python代码
Bi-LSTM是一种双向长短期记忆神经网络模型,能够有效地处理时序数据,比如自然语言处理、语音识别等任务。在Python中,我们可以使用Keras或者TensorFlow等库来实现Bi-LSTM模型。
以下是一个简单的Python代码示例,用于构建和训练一个Bi-LSTM模型:
```python
import numpy as np
from keras.models import Sequential
from keras.layers import Bidirectional, LSTM, Dense
# 生成训练数据
X_train = np.random.random((1000, 10, 100)) # 1000个样本,每个样本10个时序数据,每个时序数据有100个特征
y_train = np.random.randint(2, size=(1000, 1)) # 二分类标签
# 构建Bi-LSTM模型
model = Sequential()
model.add(Bidirectional(LSTM(64, return_sequences=True), input_shape=(10, 100)))
model.add(Bidirectional(LSTM(64)))
model.add(Dense(1, activation='sigmoid'))
# 编译模型
model.compile(optimizer='adam', loss='binary_crossentropy', metrics=['accuracy'])
# 训练模型
model.fit(X_train, y_train, batch_size=32, epochs=10, validation_split=0.2)
```
在这个示例中,我们首先使用numpy生成了一个模拟的训练数据集。然后使用Keras库构建了一个包含两个双向LSTM层和一个全连接层的Bi-LSTM模型。接着编译了模型,并使用训练数据进行模型训练。
需要注意的是,这只是一个简单的示例代码,实际应用中可能需要根据具体的任务和数据进行模型的调参和优化。希望这个例子能够帮助你更好地了解如何在Python中实现Bi-LSTM模型。
Bi-LSTM模型代码
Bi-LSTM(双向长短时记忆网络)是一种常用的深度学习模型,常用于自然语言处理和序列标注任务。其结构类似于LSTM,但引入了一个额外的反向LSTM来处理输入序列的反向信息。
以下是一个简单的Bi-LSTM模型代码示例:
```python
import torch
import torch.nn as nn
class BiLSTM(nn.Module):
def __init__(self, input_dim, hidden_dim, output_dim):
super().__init__()
self.hidden_dim = hidden_dim
self.lstm = nn.LSTM(input_dim, hidden_dim, bidirectional=True)
self.fc = nn.Linear(hidden_dim*2, output_dim)
def forward(self, text):
lstm_out, _ = self.lstm(text)
# 将前向和后向的LSTM输出拼接在一起
lstm_out = torch.cat((lstm_out[:, :, :self.hidden_dim], lstm_out[:, :, self.hidden_dim:]), dim=2)
# 取最后一个时间步的输出作为句子表示
final_out = lstm_out[:, -1, :]
# 经过全连接层得到输出
out = self.fc(final_out)
return out
```
此代码实现了一个简单的Bi-LSTM模型,包含一个双向LSTM层和一个全连接层。在前向传播过程中,输入序列首先经过双向LSTM层,得到前向和后向的输出。然后将这两个输出拼接在一起,并取最后一个时间步的输出作为句子表示。最后经过全连接层得到输出。
阅读全文
相关推荐
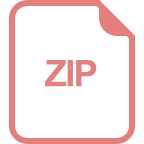
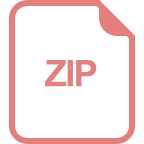
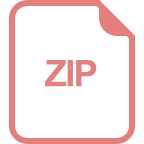













