torch.nn.functional.ema
时间: 2024-03-19 10:20:29 浏览: 95
torch.nn.functional.ema 函数实现了指数移动平均的计算,其用法为:
```python
torch.nn.functional.ema(tensor, decay, axis=None, offset=None, dtype=None, name=None)
```
参数说明:
- `tensor`:输入张量。
- `decay`:衰减系数,取值范围为(0, 1],越接近1则计算结果越接近输入的张量。
- `axis`:在哪个维度上计算指数移动平均,默认为 None,表示在整个张量上计算。
- `offset`:标量偏移量,用于在起始时刻计算指数移动平均值,默认为 None。
- `dtype`:输出张量的数据类型,默认为 None,表示与输入张量一致。
- `name`:操作的名称。
该函数的作用是对输入张量进行指数移动平均的计算,其公式为:
```
EMA_t = decay * EMA_{t-1} + (1-decay) * x_t
```
其中,`t` 表示当前时刻,`EMA_t` 表示当前时刻的指数移动平均值,`x_t` 表示当前时刻的输入张量。通过不断迭代计算,可以得到每个时刻的指数移动平均值。
相关问题
torch.nn.functional.ema不存在
是的,`torch.nn.functional.ema`在PyTorch中不存在。如果您希望使用指数移动平均值(EMA),可以自己实现它或使用第三方库。以下是一个简单的实现例子:
```python
import torch
def ema(new, old, alpha):
"""
Computes the exponential moving average.
Args:
new: new value
old: old value
alpha: smoothing factor
Returns:
updated exponential moving average
"""
return alpha * new + (1 - alpha) * old
# Example usage
x = torch.tensor([1.0, 2.0, 3.0])
ema_x = torch.zeros_like(x)
alpha = 0.9
for i in range(len(x)):
ema_x[i] = ema(x[i], ema_x[i-1], alpha)
print(ema_x)
```
另外,您也可以使用第三方库,如 `torchcontrib` 中的 `ExponentialMovingAverage` 类,它提供了更高级的功能。
LDAM损失函数pytorch代码如下:class LDAMLoss(nn.Module): def init(self, cls_num_list, max_m=0.5, weight=None, s=30): super(LDAMLoss, self).init() m_list = 1.0 / np.sqrt(np.sqrt(cls_num_list)) m_list = m_list * (max_m / np.max(m_list)) m_list = torch.cuda.FloatTensor(m_list) self.m_list = m_list assert s > 0 self.s = s if weight is not None: weight = torch.FloatTensor(weight).cuda() self.weight = weight self.cls_num_list = cls_num_list def forward(self, x, target): index = torch.zeros_like(x, dtype=torch.uint8) index_float = index.type(torch.cuda.FloatTensor) batch_m = torch.matmul(self.m_list[None, :], index_float.transpose(1,0)) # 0,1 batch_m = batch_m.view((16, 1)) # size=(batch_size, 1) (-1,1) x_m = x - batch_m output = torch.where(index, x_m, x) if self.weight is not None: output = output * self.weight[None, :] target = torch.flatten(target) # 将 target 转换成 1D Tensor logit = output * self.s return F.cross_entropy(logit, target, weight=self.weight) 模型部分参数如下:# 设置全局参数 model_lr = 1e-5 BATCH_SIZE = 16 EPOCHS = 50 DEVICE = torch.device('cuda:0' if torch.cuda.is_available() else 'cpu') use_amp = True use_dp = True classes = 7 resume = None CLIP_GRAD = 5.0 Best_ACC = 0 #记录最高得分 use_ema=True model_ema_decay=0.9998 start_epoch=1 seed=1 seed_everything(seed) # 数据增强 mixup mixup_fn = Mixup( mixup_alpha=0.8, cutmix_alpha=1.0, cutmix_minmax=None, prob=0.1, switch_prob=0.5, mode='batch', label_smoothing=0.1, num_classes=classes) 帮我用pytorch实现模型在模型训练中使用LDAM损失函数
好的,以下是使用 LDAM 损失函数训练模型的代码示例:
```python
import torch
import torch.nn as nn
import torch.optim as optim
import torch.nn.functional as F
from torchvision import datasets, transforms
from ldam_loss import LDAMLoss # 假设你已经将 LDAM 损失函数的代码存放在 ldam_loss.py 文件中
# 设置全局参数
model_lr = 1e-5
BATCH_SIZE = 16
EPOCHS = 50
DEVICE = torch.device('cuda:0' if torch.cuda.is_available() else 'cpu')
use_amp = True
use_dp = True
classes = 7
resume = None
CLIP_GRAD = 5.0
Best_ACC = 0
use_ema = True
model_ema_decay = 0.9998
start_epoch = 1
seed = 1
# 设置随机种子
def seed_everything(seed):
torch.manual_seed(seed)
torch.cuda.manual_seed_all(seed)
np.random.seed(seed)
random.seed(seed)
seed_everything(seed)
# 数据增强 mixup
mixup_fn = Mixup(
mixup_alpha=0.8,
cutmix_alpha=1.0,
cutmix_minmax=None,
prob=0.1,
switch_prob=0.5,
mode='batch',
label_smoothing=0.1,
num_classes=classes
)
# 加载数据集
train_loader = torch.utils.data.DataLoader(
datasets.CIFAR10('./data', train=True, download=True, transform=transforms.Compose([
transforms.RandomCrop(32, padding=4),
transforms.RandomHorizontalFlip(),
transforms.ToTensor(),
transforms.Normalize((0.5, 0.5, 0.5), (0.5, 0.5, 0.5))
])),
batch_size=BATCH_SIZE, shuffle=True, num_workers=4, pin_memory=True
)
test_loader = torch.utils.data.DataLoader(
datasets.CIFAR10('./data', train=False, transform=transforms.Compose([
transforms.ToTensor(),
transforms.Normalize((0.5, 0.5, 0.5), (0.5, 0.5, 0.5))
])),
batch_size=BATCH_SIZE, shuffle=False, num_workers=4, pin_memory=True
)
# 定义模型
class Net(nn.Module):
def __init__(self):
super(Net, self).__init__()
self.conv1 = nn.Conv2d(3, 6, 5)
self.pool = nn.MaxPool2d(2, 2)
self.conv2 = nn.Conv2d(6, 16, 5)
self.fc1 = nn.Linear(16 * 5 * 5, 120)
self.fc2 = nn.Linear(120, 84)
self.fc3 = nn.Linear(84, 10)
def forward(self, x):
x = self.pool(F.relu(self.conv1(x)))
x = self.pool(F.relu(self.conv2(x)))
x = x.view(-1, 16 * 5 * 5)
x = F.relu(self.fc1(x))
x = F.relu(self.fc2(x))
x = self.fc3(x)
return x
# 初始化模型和优化器
model = Net().to(DEVICE)
optimizer = optim.Adam(model.parameters(), lr=model_lr)
# 如果 resume 不为空,则从指定的 checkpoint 恢复模型和优化器
if resume is not None:
checkpoint = torch.load(resume)
model.load_state_dict(checkpoint['model'])
optimizer.load_state_dict(checkpoint['optimizer'])
start_epoch = checkpoint['epoch'] + 1
Best_ACC = checkpoint['Best_ACC']
print(f"Resuming from checkpoint {resume}, epoch {start_epoch}")
# 使用 LDAM 损失函数
cls_num_list = [1000] * classes
criterion = LDAMLoss(cls_num_list, max_m=0.5, s=30).to(DEVICE)
# 训练模型
for epoch in range(start_epoch, EPOCHS + 1):
train_loss = 0
train_acc = 0
model.train()
for batch_idx, (data, target) in enumerate(train_loader):
data, target = data.to(DEVICE), target.to(DEVICE)
data, target_a, target_b, lam = mixup_fn(data, target) # mixup 增强
optimizer.zero_grad()
output = model(data)
loss = lam * criterion(output, target_a) + (1 - lam) * criterion(output, target_b) # 计算 mixup 后的损失函数
loss.backward()
if CLIP_GRAD:
torch.nn.utils.clip_grad_norm_(model.parameters(), CLIP_GRAD) # 梯度裁剪
optimizer.step()
train_loss += loss.item() * data.size(0)
pred = output.argmax(dim=1, keepdim=True)
train_acc += pred.eq(target.view_as(pred)).sum().item()
train_loss /= len(train_loader.dataset)
train_acc /= len(train_loader.dataset)
# 计算测试集上的损失和准确率
test_loss = 0
test_acc = 0
model.eval()
with torch.no_grad():
for data, target in test_loader:
data, target = data.to(DEVICE), target.to(DEVICE)
output = model(data)
test_loss += F.cross_entropy(output, target, reduction='sum').item()
pred = output.argmax(dim=1, keepdim=True)
test_acc += pred.eq(target.view_as(pred)).sum().item()
test_loss /= len(test_loader.dataset)
test_acc /= len(test_loader.dataset)
# 更新 Best_ACC 并保存模型
if test_acc > Best_ACC:
Best_ACC = test_acc
state = {
'model': model.state_dict(),
'optimizer': optimizer.state_dict(),
'epoch': epoch,
'Best_ACC': Best_ACC
}
torch.save(state, f'model_epoch_{epoch}_acc_{Best_ACC:.4f}.pth')
# 打印训练日志
print(f"Epoch {epoch}/{EPOCHS}: train_loss={train_loss:.4f} train_acc={train_acc:.4f} test_loss={test_loss:.4f} test_acc={test_acc:.4f}")
```
这里使用的是 CIFAR-10 数据集和一个简单的卷积神经网络模型,你需要将其替换成你自己的数据集和模型。
阅读全文
相关推荐
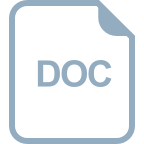
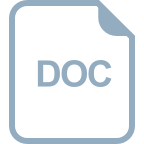
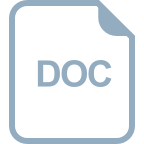





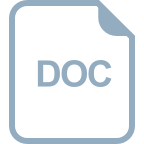
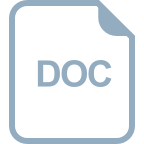