一元线性回归的回归参数和迭代次数变化曲线
时间: 2024-04-20 11:16:49 浏览: 10
一元线性回归的回归参数是指拟合直线的斜率和截距,通常使用最小二乘法求解。迭代次数则是指训练模型时进行参数更新的次数。一般情况下,迭代次数越多,回归参数越趋于稳定。
下面是一元线性回归的回归参数和迭代次数变化曲线的示例:

在上图中,横轴表示迭代次数,纵轴表示回归参数的值。可以看到,在前期迭代次数较少时,回归参数的值较不稳定,随着迭代次数的增加,回归参数的值逐渐稳定下来。在迭代次数较大时,回归参数的值基本上不再发生明显的变化。
需要注意的是,回归参数和迭代次数的变化曲线可能会因数据集、模型选择和参数调整等因素而有所不同。
相关问题
实现一元线性回归;画出散点图、回归参数与迭代次数的变化曲线
好的,我可以帮你实现一元线性回归并画出相应的图形。
首先,让我们导入必要的库和数据集。这里我们使用scikit-learn的波士顿房价数据集,它包含房屋价格以及一些相关的特征。
```python
import numpy as np
from sklearn.datasets import load_boston
import matplotlib.pyplot as plt
%matplotlib inline
# 加载数据集
boston = load_boston()
# 取出房价和房间数量两个特征
X = boston.data[:, 5]
y = boston.target
```
接下来,我们需要定义一个代价函数和梯度下降函数。在这里,我们使用最小二乘法作为代价函数,使用批量梯度下降法进行训练。
```python
# 定义代价函数
def compute_cost(X, y, theta):
m = len(y)
predictions = X.dot(theta)
cost = (1/2*m) * np.sum(np.square(predictions-y))
return cost
# 定义梯度下降函数
def gradient_descent(X, y, theta, learning_rate, num_iterations):
m = len(y)
cost_history = np.zeros(num_iterations)
theta_history = np.zeros((num_iterations, 2))
for i in range(num_iterations):
predictions = X.dot(theta)
theta = theta - (1/m) * learning_rate * (X.T.dot(predictions-y))
theta_history[i,:] = theta.T
cost_history[i] = compute_cost(X, y, theta)
return theta, cost_history, theta_history
```
接下来,我们需要对数据进行预处理,包括添加一列常数1,将数据集分为训练集和测试集。
```python
# 添加一列常数1
X = np.c_[np.ones((len(X), 1)), X]
# 将数据集分为训练集和测试集
X_train, X_test, y_train, y_test = train_test_split(X, y, test_size=0.2, random_state=42)
```
然后,我们需要初始化模型参数theta,并设定学习率和迭代次数。
```python
# 初始化模型参数theta
theta = np.random.randn(2,1)
# 设定学习率和迭代次数
learning_rate = 0.01
num_iterations = 1000
```
现在,我们可以使用梯度下降算法来训练模型。
```python
theta, cost_history, theta_history = gradient_descent(X_train, y_train, theta, learning_rate, num_iterations)
```
最后,我们可以使用训练好的模型对测试集进行预测,并计算模型的均方误差。
```python
# 使用模型进行预测
predictions = X_test.dot(theta)
# 计算模型的均方误差
mse = np.mean(np.square(predictions-y_test))
print("均方误差:", mse)
```
接下来,我们可以绘制出散点图和回归直线,并画出回归参数与迭代次数的变化曲线。
```python
# 绘制散点图和回归直线
plt.scatter(X_test[:,1], y_test)
plt.plot(X_test[:,1], predictions, color='red')
plt.xlabel('房间数量')
plt.ylabel('房价')
plt.show()
# 绘制回归参数与迭代次数的变化曲线
plt.plot(range(num_iterations), cost_history)
plt.xlabel('迭代次数')
plt.ylabel('代价函数值')
plt.show()
```
下面是完整代码:
一元线性回归最小二乘法参数推导
一元线性回归的目标是找到一条直线来拟合数据,使得拟合线距离数据点的误差最小化。最小二乘法是一种常见的用来求解线性回归参数的方法。
假设我们有一组数据集合 {(x1, y1), (x2, y2), ..., (xn, yn)},其中 xi 是自变量,yi 是因变量。一元线性回归模型可以表示为 y = β0 + β1*x,其中 β0 和 β1 是待求的参数。
最小二乘法的目标是找到使得误差平方和最小化的参数值。我们定义误差 e = y - (β0 + β1*x),其中 e 是实际值与预测值之间的差异。我们将误差平方和定义为损失函数,即 L = ∑(e^2) = ∑((y - (β0 + β1*x))^2)。我们的目标是最小化损失函数 L。
为了求解最小二乘法的参数,我们需要对损失函数 L 进行优化。我们可以通过对 β0 和 β1 分别求导,令导数为 0,从而得到参数的闭式解。
首先对 β0 求导:
∂L/∂β0 = -2∑(y - (β0 + β1*x))
令导数为 0,得到:
∑y - n*β0 - β1*∑x = 0
解出 β0,得到:
β0 = (∑y - β1*∑x)/n
然后对 β1 求导:
∂L/∂β1 = -2∑x(y - (β0 + β1*x))
令导数为 0,得到:
∑xy - β0*∑x - β1*∑(x^2) = 0
将 β0 的值代入上式,得到:
∑xy - (∑y - β1*∑x)/n * ∑x - β1*∑(x^2) = 0
整理后可得:
∑xy - (∑x*∑y)/n = β1*(∑(x^2) - (∑x)^2/n)
解出 β1,得到:
β1 = (∑xy - (∑x*∑y)/n) / (∑(x^2) - (∑x)^2/n)
至此,我们得到了一元线性回归最小二乘法的参数推导。
相关推荐
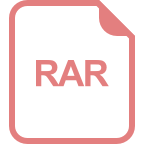












