WARNING:tensorflow:Model was constructed with shape (None, 100, 1) for input KerasTensor(type_spec=TensorSpec(shape=(None, 100, 1), dtype=tf.float32, name='input_1'), name='input_1', description="created by layer 'input_1'"), but it was called on an input with incompatible shape (None, 88, 1). WARNING:tensorflow:Model was constructed with shape (None, 100, 1) for input KerasTensor(type_spec=TensorSpec(shape=(None, 100, 1), dtype=tf.float32, name='input_2'), name='input_2', description="created by layer 'input_2'"), but it was called on an input with incompatible shape (None, 137, 1).
时间: 2024-04-26 11:20:36 浏览: 9
这是一个 TensorFlow 的警告信息,提示模型的输入与实际输入的维度不匹配。根据警告信息,模型的输入应该是一个形状为 (None, 100, 1) 的张量,但是实际输入的张量形状不一致,分别是 (None, 88, 1) 和 (None, 137, 1)。这可能是由于模型的输入设置不正确或者实际输入数据的维度不符合要求导致的。如果想要解决这个问题,需要检查模型的输入设置以及实际输入数据的维度是否匹配。
相关问题
WARNING:tensorflow:Model was constructed with shape (128, 24, 2) for input KerasTensor(type_spec=TensorSpec(shape=(128, 24, 2), dtype=tf.float32, name='RealData'), name='RealData', description="created by layer 'RealData'"), but it was called on an input with incompatible shape (6, 24, 2). WARNING:tensorflow:Model was constructed with shape (128, 24, 2) for input KerasTensor(type_spec=TensorSpec(shape=(128, 24, 2), dtype=tf.float32, name='RealData'), name='RealData', description="created by layer 'RealData'"), but it was called on an input with incompatible shape (6, 24, 2).
_dim = hidden_dim // num_heads
self.query_linear = nn.Linear(hidden_dim, hidden_dim)
self.key_linear = nn.Linear(hidden_dim, hidden_dim)
self.value_linear = nn.Linear(hidden_dim, hidden_dim)
self.out_linear = nn.Linear(hidden_dim, hidden这些警告表明模型在使用时遇到了输入形状不匹配的问题。警告中提到的_dim)
def forward(self, query, key, value):
batch_size = query.size(0)
query = self.query_linear(query两个形状`(128, 24, 2)`和`(6, 24, 2)`分别表示模型定义)
key = self.key_linear(key)
value = self.value_linear(value)
query = query.view(batch_size, -1, self时期望的输入形状和实际传入的输入形状。
要解决这个问题,需要确保输入数据.num_heads, self.head_dim).transpose(1, 2)
key = key.view(batch_size, -1, self.num_heads,的形状与模型定义时期望的形状一致。在这个例子中,期望的形状是 self.head_dim).transpose(1, 2)
value = value.view(batch_size, -1, self.num_heads, self.head_dim`(128, 24, 2)`,但实际传入的形状是`(6, 24, 2)`,).transpose(1, 2)
scores = torch.matmul(query, key.transpose(-2, -1)) / torch.sqrt(torch.tensor(self.head_dim))
attention_weights = nn.functional.softmax(scores, dim=-1)
attended_values = torch.matmul(attention_weights, value)
所以它们不匹配。
可能的解决方案包括:
1. 检查数据预处理部分的代码, attended_values = attended_values.transpose(1, 2).contiguous().view(batch_size, -1, self.num_heads * self.head确保输入数据被正确地转换为期望的形状。
2. 检查数据加载部分的代码,确保_dim)
output = self.out_linear(attended_values)
return output
# 定义前馈神经网络模块
class Feed正确加载具有期望形状的数据。
3. 检查模型定义部分的代码,确保模型的输入Forward(nn.Module):
def __init__(self, hidden_dim):
super(FeedForward, self).__init__()
self.linear1 =层与期望的形状一致。
通过解决输入形状不匹配的问题,警告应该会消失 nn.Linear(hidden_dim, hidden_dim * 4)
self.linear2 = nn.Linear(hidden_dim * 4, hidden_dim)
def。如果问题仍然存在,请提供更多代码和详细信息,以便更好地帮助你解决问题。
上面的代码改进后出现WARNING:tensorflow:Model was constructed with shape (None, 224, 224, 3) for input KerasTensor(type_spec=TensorSpec(shape=(None, 224, 224, 3), dtype=tf.float32, name='input_5'), name='input_5', description="created by layer 'input_5'"), but it was called on an input with incompatible shape (None, 100, 125, 3).
这个警告是因为在构建模型时,我们定义了输入张量的形状为 (None, 224, 224, 3),但实际使用时输入张量的形状为 (None, 100, 125, 3),两者不匹配。这是因为我们将 MobileNetV2 模型的输入形状定义为 (224, 224, 3),而在新模型中我们定义了新的输入形状为 (100, 125, 3)。
虽然这个警告不会影响模型的训练和预测,但为了消除警告,我们可以将 MobileNetV2 模型的输入形状与新模型的输入形状匹配。具体来说,我们可以使用 `tf.keras.layers.experimental.preprocessing` 中的 `Resizing` 层将输入张量的大小调整为 (224, 224)。修改后的代码如下所示:
```
from tensorflow.keras import layers, models
from tensorflow.keras.layers.experimental.preprocessing import Resizing
# Define your new input layer with the desired shape
new_input = layers.Input(shape=(100, 125, 3))
# Load the MobileNetV2 model with the top layer removed
mobile = tf.keras.applications.MobileNetV2(input_shape=(224, 224, 3), include_top=False, weights='imagenet')
# Freeze the weights of the MobileNetV2 layers
for layer in mobile.layers:
layer.trainable = False
# Resize the input to match the input shape of the MobileNetV2 model
resized_input = Resizing(224, 224)(new_input)
# Get the output of the MobileNetV2 model
x = mobile(resized_input)
# Add a global average pooling layer and a dense layer for classification
x = layers.GlobalAveragePooling2D()(x)
x = layers.Dense(128, activation='relu')(x)
predictions = layers.Dense(10, activation='softmax')(x)
# Create the new model
new_model = models.Model(inputs=new_input, outputs=predictions)
# Compile the new model
new_model.compile(optimizer='adam', loss='categorical_crossentropy', metrics=['accuracy'])
# Print the summary of the new model
new_model.summary()
```
在这个修改后的代码中,我们使用 `Resizing` 层将输入张量的大小调整为 (224, 224),然后将调整后的张量作为 MobileNetV2 模型的输入。这样可以保证输入形状的匹配,并消除警告。
相关推荐
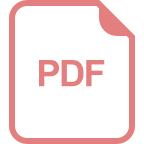








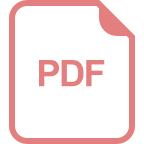
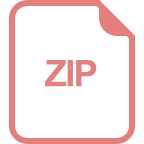
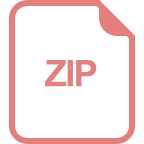
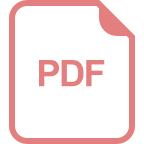
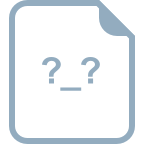