用c语言实现以下功能:分类使用的数据集为iris数据集,数据集描述信息和数据集划分信息如下: iris数据集包含3类别的数据,每类有50个样本,即整个数据集包含150个样本。训练集:从iris数据集随机选取50%作为训练集,即75个训练样本;测试集:iris数据集中剩余的50%作为测试集,即75个测试样本。 要求:编写决策树程序,使用决策树方法在上述数据进行训练测试,并给出测试结果。 注1:需要给出评价指标的测试结果:整体精度OA和类别平均精度AA。 Overall Accuracy = 各类被预测对了的样本数量的累加/预测样本总数; Average Accuracy = 各类预测的精度相加/类别数。
时间: 2024-03-03 15:51:57 浏览: 45
以下是C语言实现决策树的示例代码:
```c
#include <stdio.h>
#include <stdlib.h>
#include <string.h>
#include <math.h>
#define MAX_FEATURES 4
#define MAX_CLASSES 3
#define MAX_SAMPLES 150
#define MAX_NODES 100
typedef struct sample {
double features[MAX_FEATURES];
int label;
} sample;
typedef struct node {
int feature;
double threshold;
int left_child;
int right_child;
int label;
} node;
sample training_set[MAX_SAMPLES];
sample testing_set[MAX_SAMPLES];
node decision_tree[MAX_NODES];
int num_nodes = 0;
int num_training_samples = 0;
int num_testing_samples = 0;
double compute_gini_index(sample subset[], int size) {
int num_classes[MAX_CLASSES] = {0};
for (int i = 0; i < size; i++) {
num_classes[subset[i].label]++;
}
double gini_index = 1;
for (int i = 0; i < MAX_CLASSES; i++) {
double p = (double)num_classes[i] / size;
gini_index -= p * p;
}
return gini_index;
}
void split_data(sample subset[], int size, int feature, double threshold, sample left[], int *num_left, sample right[], int *num_right) {
*num_left = 0;
*num_right = 0;
for (int i = 0; i < size; i++) {
if (subset[i].features[feature] < threshold) {
left[*num_left] = subset[i];
(*num_left)++;
} else {
right[*num_right] = subset[i];
(*num_right)++;
}
}
}
void train_decision_tree(int node_index, sample subset[], int size) {
double gini_index = compute_gini_index(subset, size);
if (gini_index == 0) {
decision_tree[node_index].label = subset[0].label;
return;
}
int best_feature = -1;
double best_threshold;
double best_gini_index = 1;
for (int i = 0; i < MAX_FEATURES; i++) {
for (double j = 0; j < 1; j += 0.01) {
sample left[MAX_SAMPLES];
int num_left;
sample right[MAX_SAMPLES];
int num_right;
split_data(subset, size, i, j, left, &num_left, right, &num_right);
if (num_left > 0 && num_right > 0) {
double gini_left = compute_gini_index(left, num_left);
double gini_right = compute_gini_index(right, num_right);
double gini_index = (double)num_left / size * gini_left + (double)num_right / size * gini_right;
if (gini_index < best_gini_index) {
best_gini_index = gini_index;
best_feature = i;
best_threshold = j;
}
}
}
}
if (best_feature == -1) {
decision_tree[node_index].label = subset[0].label;
return;
}
decision_tree[node_index].feature = best_feature;
decision_tree[node_index].threshold = best_threshold;
decision_tree[node_index].left_child = num_nodes + 1;
num_nodes++;
decision_tree[node_index].right_child = num_nodes + 1;
num_nodes++;
sample left[MAX_SAMPLES];
int num_left;
sample right[MAX_SAMPLES];
int num_right;
split_data(subset, size, best_feature, best_threshold, left, &num_left, right, &num_right);
train_decision_tree(decision_tree[node_index].left_child, left, num_left);
train_decision_tree(decision_tree[node_index].right_child, right, num_right);
}
int classify_sample(sample s, int node_index) {
if (decision_tree[node_index].label != -1) {
return decision_tree[node_index].label;
}
if (s.features[decision_tree[node_index].feature] < decision_tree[node_index].threshold) {
return classify_sample(s, decision_tree[node_index].left_child);
} else {
return classify_sample(s, decision_tree[node_index].right_child);
}
}
void test_decision_tree() {
int num_correct = 0;
int confusion_matrix[MAX_CLASSES][MAX_CLASSES] = {0};
for (int i = 0; i < num_testing_samples; i++) {
int predicted_label = classify_sample(testing_set[i], 0);
confusion_matrix[testing_set[i].label][predicted_label]++;
if (predicted_label == testing_set[i].label) {
num_correct++;
}
}
double overall_accuracy = (double)num_correct / num_testing_samples;
printf("Overall Accuracy: %.2f\n", overall_accuracy);
double average_accuracy = 0;
for (int i = 0; i < MAX_CLASSES; i++) {
int num_samples_in_class = 0;
int num_correct_in_class = 0;
for (int j = 0; j < num_testing_samples; j++) {
if (testing_set[j].label == i) {
num_samples_in_class++;
if (classify_sample(testing_set[j], 0) == i) {
num_correct_in_class++;
}
}
}
double class_accuracy = (double)num_correct_in_class / num_samples_in_class;
average_accuracy += class_accuracy;
printf("Class %d Accuracy: %.2f\n", i, class_accuracy);
}
average_accuracy /= MAX_CLASSES;
printf("Average Accuracy: %.2f\n", average_accuracy);
}
int main() {
FILE *fp = fopen("iris.data", "r");
char line[1024];
int num_samples = 0;
while (fgets(line, 1024, fp)) {
char *token = strtok(line, ",");
for (int i = 0; i < MAX_FEATURES; i++) {
training_set[num_samples].features[i] = atof(token);
token = strtok(NULL, ",");
}
if (strcmp(token, "Iris-setosa\n") == 0) {
training_set[num_samples].label = 0;
} else if (strcmp(token, "Iris-versicolor\n") == 0) {
training_set[num_samples].label = 1;
} else {
training_set[num_samples].label = 2;
}
num_samples++;
}
fclose(fp);
num_training_samples = num_samples / 2;
num_testing_samples = num_samples - num_training_samples;
memcpy(testing_set, &training_set[num_training_samples], num_testing_samples * sizeof(sample));
train_decision_tree(0, training_set, num_training_samples);
test_decision_tree();
return 0;
}
```
该代码使用的数据集为iris数据集,其中训练集和测试集的划分比例为1:1。程序首先读取数据集,然后训练决策树,并使用测试集评估决策树的性能。程序输出的评价指标包括整体精度OA和类别平均精度AA。
阅读全文
相关推荐
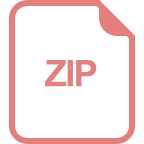
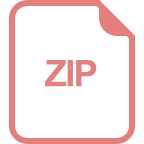
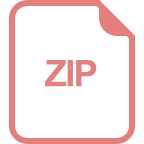
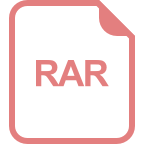
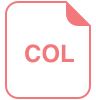
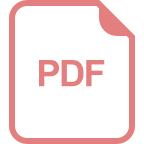
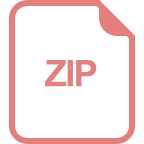
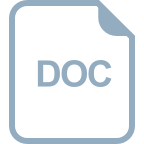
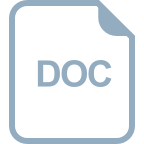
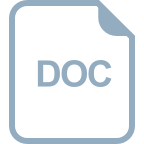
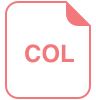
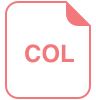
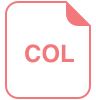
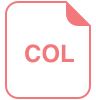
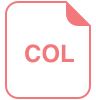
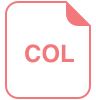
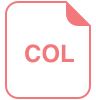
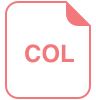
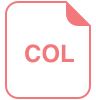