assert torch.cuda.is_available() and torch.cuda.device_count() >= len(device.replace(',', '')), \
时间: 2023-11-13 07:36:01 浏览: 235
这行代码的作用是检查当前计算机是否支持 CUDA 并且是否有足够数量的 GPU 设备可供使用。在这行代码中,我们使用了 assert 语句来断言这个条件,如果条件不成立(即当前计算机不支持 CUDA 或者可用的 GPU 设备数量不足),那么程序将会抛出 AssertionError 异常。其中,torch.cuda.is_available() 函数用于检查计算机是否支持 CUDA,torch.cuda.device_count() 函数用于获取当前计算机上可用的 GPU 设备数量,而 len(device.replace(',', '')) 则是用来将逗号分隔的 GPU 设备编号字符串转化为对应的设备数量。
相关问题
def use_cuda(enabled, device_id=0):#CUDA检测 if enabled: assert torch.cuda.is_available(), 'CUDA is not available' torch.cuda.set_device(device_id)
这是一个Python函数,用于启用/禁用CUDA加速,并选择使用哪个CUDA设备。如果enabled为True,则启用CUDA加速,并使用device_id指定的设备(默认为0)。如果enabled为False,则禁用CUDA加速。
try: import thop except ImportError: thop = None logger = logging.getLogger(__name__) @contextmanager def torch_distributed_zero_first(local_rank: int): if local_rank not in [-1, 0]: torch.distributed.barrier() yield if local_rank == 0: torch.distributed.barrier() def init_torch_seeds(seed=0): torch.manual_seed(seed) if seed == 0: cudnn.benchmark, cudnn.deterministic = False, True else: cudnn.benchmark, cudnn.deterministic = True, False def select_device(device='', batch_size=None): s = f'YOLOv5 🚀 {git_describe() or date_modified()} torch {torch.__version__} ' cpu = device.lower() == 'cpu' if cpu: os.environ['CUDA_VISIBLE_DEVICES'] = '-1' elif device: # non-cpu device requested os.environ['CUDA_VISIBLE_DEVICES'] = device assert torch.cuda.is_available(), f'CUDA unavailable, invalid device {device} requested' cuda = not cpu and torch.cuda.is_available() if cuda: n = torch.cuda.device_count() if n > 1 and batch_size: # check that batch_size is compatible with device_count assert batch_size % n == 0, f'batch-size {batch_size} not multiple of GPU count {n}' space = ' ' * len(s) for i, d in enumerate(device.split(',') if device else range(n)): p = torch.cuda.get_device_properties(i) s += f"{'' if i == 0 else space}CUDA:{d} ({p.name}, {p.total_memory / 1024 ** 2}MB)\n" s += 'CPU\n' logger.info(s.encode().decode('ascii', 'ignore') if platform.system() == 'Windows' else s) # emoji-safe return torch.device('cuda:0' if cuda else 'cpu') def time_synchronized(): if torch.cuda.is_available(): torch.cuda.synchronize() return time.time()
这段代码是一个Python脚本,包含了一些用于PyTorch训练的实用函数。具体来说,它包含了以下函数:
- import thop:导入PyTorch的模块thop,用于计算模型的FLOPs和参数数量。
- torch_distributed_zero_first:用于在分布式训练中同步所有进程的执行顺序。
- init_torch_seeds:用于初始化PyTorch的随机数种子。
- select_device:用于选择PyTorch的设备,可以选择CPU或GPU。
- time_synchronized:用于在CPU和GPU之间同步时间。
这些函数都是用于方便PyTorch训练的实用函数,可以大大简化代码编写。
阅读全文
相关推荐
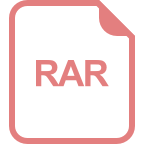
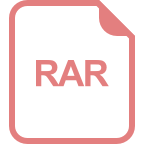
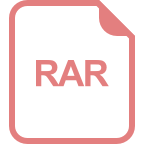










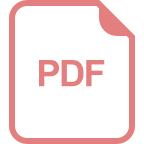
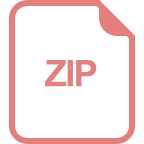
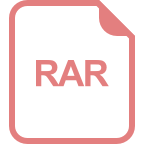
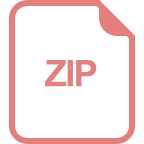