适用于离散动作空间的MADDPG代码
时间: 2023-07-02 14:16:05 浏览: 260
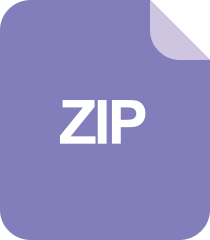
matlab_Actor-Critic简单应用例子,连续状态空间,离散动作

以下是适用于离散动作空间的MADDPG代码:
```
import torch
import torch.nn.functional as F
import numpy as np
from collections import deque, namedtuple
import random
device = torch.device("cuda" if torch.cuda.is_available() else "cpu")
BUFFER_SIZE = int(1e6) # replay buffer size
BATCH_SIZE = 128 # minibatch size
GAMMA = 0.99 # discount factor
TAU = 1e-3 # for soft update of target parameters
LR_ACTOR = 1e-3 # learning rate of the actor
LR_CRITIC = 1e-3 # learning rate of the critic
WEIGHT_DECAY = 0 # L2 weight decay
class MADDPG:
def __init__(self, state_size, action_size, num_agents, seed):
self.state_size = state_size
self.action_size = action_size
self.num_agents = num_agents
self.seed = random.seed(seed)
# Actor Network (w/ Target Network)
self.actor_local = Actor(state_size, action_size, seed).to(device)
self.actor_target = Actor(state_size, action_size, seed).to(device)
self.actor_optimizer = optim.Adam(self.actor_local.parameters(), lr=LR_ACTOR)
# Critic Network (w/ Target Network)
self.critic_local = Critic(state_size, action_size, num_agents, seed).to(device)
self.critic_target = Critic(state_size, action_size, num_agents, seed).to(device)
self.critic_optimizer = optim.Adam(self.critic_local.parameters(), lr=LR_CRITIC, weight_decay=WEIGHT_DECAY)
# Noise process
self.noise = OUNoise((num_agents, action_size), seed)
# Replay memory
self.memory = ReplayBuffer(action_size, BUFFER_SIZE, BATCH_SIZE, seed)
def act(self, states, add_noise=True):
states = torch.from_numpy(states).float().to(device)
actions = np.zeros((self.num_agents, self.action_size))
self.actor_local.eval()
with torch.no_grad():
for agent_idx, state in enumerate(states):
action = self.actor_local(state.unsqueeze(0)).cpu().data.numpy()
actions[agent_idx, :] = action
self.actor_local.train()
if add_noise:
actions += self.noise.sample()
return np.clip(actions, -1, 1)
def reset(self):
self.noise.reset()
def learn(self, experiences, gamma):
states, actions, rewards, next_states, dones = experiences
# ---------------------------- update critic ---------------------------- #
# Get predicted next-state actions and Q values from target models
actions_next = torch.zeros_like(actions)
for agent_idx, state in enumerate(next_states):
actions_next[agent_idx, :] = self.actor_target(state.unsqueeze(0)).detach()
actions_next = actions_next.view(-1, self.num_agents * self.action_size)
with torch.no_grad():
Q_targets_next = self.critic_target(next_states.view(-1, self.num_agents * self.state_size), actions_next)
Q_targets = rewards.view(-1, self.num_agents) + (gamma * Q_targets_next * (1 - dones.view(-1, self.num_agents)))
Q_expected = self.critic_local(states.view(-1, self.num_agents * self.state_size), actions.view(-1, self.num_agents * self.action_size))
critic_loss = F.mse_loss(Q_expected, Q_targets)
self.critic_optimizer.zero_grad()
critic_loss.backward()
torch.nn.utils.clip_grad_norm_(self.critic_local.parameters(), 1)
self.critic_optimizer.step()
# ---------------------------- update actor ---------------------------- #
actions_pred = torch.zeros_like(actions)
for agent_idx, state in enumerate(states):
actions_pred[agent_idx, :] = self.actor_local(state.unsqueeze(0))
actions_pred = actions_pred.view(-1, self.num_agents * self.action_size)
actor_loss = -self.critic_local(states.view(-1, self.num_agents * self.state_size), actions_pred).mean()
self.actor_optimizer.zero_grad()
actor_loss.backward()
self.actor_optimizer.step()
# ----------------------- update target networks ----------------------- #
self.soft_update(self.critic_local, self.critic_target, TAU)
self.soft_update(self.actor_local, self.actor_target, TAU)
def soft_update(self, local_model, target_model, tau):
"""Soft update model parameters."""
for target_param, local_param in zip(target_model.parameters(), local_model.parameters()):
target_param.data.copy_(tau*local_param.data + (1.0-tau)*target_param.data)
class Actor(nn.Module):
def __init__(self, state_size, action_size, seed):
super(Actor, self).__init__()
self.seed = torch.manual_seed(seed)
self.fc1 = nn.Linear(state_size, 64)
self.fc2 = nn.Linear(64, 64)
self.fc3 = nn.Linear(64, action_size)
self.bn1 = nn.BatchNorm1d(64)
self.bn2 = nn.BatchNorm1d(64)
def forward(self, state):
x = F.relu(self.bn1(self.fc1(state)))
x = F.relu(self.bn2(self.fc2(x)))
return torch.tanh(self.fc3(x))
class Critic(nn.Module):
def __init__(self, state_size, action_size, num_agents, seed):
super(Critic, self).__init__()
self.seed = torch.manual_seed(seed)
self.fcs1 = nn.Linear(num_agents*state_size, 64)
self.fc2 = nn.Linear(64+num_agents*action_size, 64)
self.fc3 = nn.Linear(64, 1)
self.bn1 = nn.BatchNorm1d(64)
self.bn2 = nn.BatchNorm1d(64)
def forward(self, state, action):
xs = F.relu(self.bn1(self.fcs1(state)))
x = torch.cat((xs, action), dim=1)
x = F.relu(self.bn2(self.fc2(x)))
return self.fc3(x)
class OUNoise:
"""Ornstein-Uhlenbeck process."""
def __init__(self, size, seed, mu=0., theta=0.15, sigma=0.2):
"""Initialize parameters and noise process."""
self.mu = mu * np.ones(size)
self.theta = theta
self.sigma = sigma
self.seed = random.seed(seed)
self.reset()
def reset(self):
"""Reset the internal state (= noise) to mean (mu)."""
self.state = np.copy(self.mu)
def sample(self):
"""Update internal state and return it as a noise sample."""
x = self.state
dx = self.theta * (self.mu - x) + self.sigma * np.random.standard_normal(self.mu.shape)
self.state = x + dx
return self.state
class ReplayBuffer:
"""Fixed-size buffer to store experience tuples."""
def __init__(self, action_size, buffer_size, batch_size, seed):
"""Initialize a ReplayBuffer object.
Params
======
action_size (int): dimension of each action
buffer_size (int): maximum size of buffer
batch_size (int): size of each training batch
seed (int): random seed
"""
self.action_size = action_size
self.memory = deque(maxlen=buffer_size) # internal memory (deque)
self.batch_size = batch_size
self.experience = namedtuple("Experience", field_names=["state", "action", "reward", "next_state", "done"])
self.seed = random.seed(seed)
def add(self, state, action, reward, next_state, done):
"""Add a new experience to memory."""
e = self.experience(state, action, reward, next_state, done)
self.memory.append(e)
def sample(self):
"""Randomly sample a batch of experiences from memory."""
experiences = random.sample(self.memory, k=self.batch_size)
states = torch.from_numpy(np.vstack([e.state for e in experiences if e is not None])).float().to(device)
actions = torch.from_numpy(np.vstack([e.action for e in experiences if e is not None])).float().to(device)
rewards = torch.from_numpy(np.vstack([e.reward for e in experiences if e is not None])).float().to(device)
next_states = torch.from_numpy(np.vstack([e.next_state for e in experiences if e is not None])).float().to(device)
dones = torch.from_numpy(np.vstack([e.done for e in experiences if e is not None]).astype(np.uint8)).float().to(device)
return (states, actions, rewards, next_states, dones)
def __len__(self):
"""Return the current size of internal memory."""
return len(self.memory)
```
在上述代码中,我们定义了一个名为“MADDPG”的类,该类包含了用于多智能体强化学习的所有组件,包括Actor和Critic神经网络、经验回放缓冲区、Ornstein-Uhlenbeck噪声过程等。
我们还定义了Actor和Critic神经网络模型,这些模型的结构与单智能体强化学习中的模型类似,但是我们需要为每个智能体定义一个单独的Actor和Critic。此外,我们还定义了一个Ornstein-Uhlenbeck噪声过程,用于添加随机性。
在主循环中,我们使用ReplayBuffer来存储智能体的经验,并定期从中随机抽取一个批次进行训练。在训练中,我们使用MADDPG算法更新Critic和Actor模型,从而使智能体能够逐渐优化其策略。
阅读全文
相关推荐
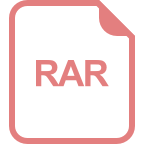
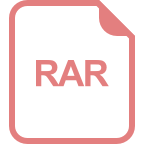
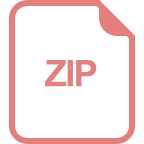
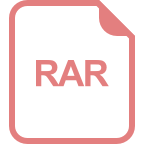
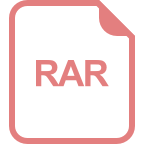
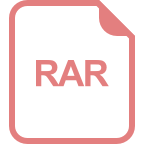
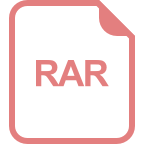
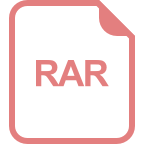
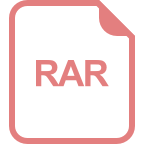
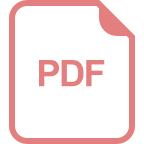