用jupyter读取csv文件,用sklearn实现随机森林分类,分析特征的重要性
时间: 2023-07-15 09:15:26 浏览: 98
首先,我们需要安装必要的库,包括`pandas`、`numpy`、`sklearn`等。使用以下命令进行安装:
```
!pip install pandas numpy sklearn
```
假设我们的csv文件名为`data.csv`,首先我们需要读取该文件并将其转换为pandas的DataFrame格式:
```python
import pandas as pd
df = pd.read_csv('data.csv')
```
接着,我们需要对数据进行预处理,包括将类别型变量进行独热编码等。这里为了简化示例,我们假设数据已经进行了预处理。
然后,我们可以将数据分成训练集和测试集:
```python
from sklearn.model_selection import train_test_split
X = df.drop(['label'], axis=1)
y = df['label']
X_train, X_test, y_train, y_test = train_test_split(X, y, test_size=0.2)
```
接下来,我们可以使用sklearn中的`RandomForestClassifier`来实现随机森林分类:
```python
from sklearn.ensemble import RandomForestClassifier
clf = RandomForestClassifier(n_estimators=100, max_depth=5, random_state=42)
clf.fit(X_train, y_train)
```
最后,我们可以使用`feature_importances_`属性来分析特征的重要性:
```python
importance = clf.feature_importances_
for i,v in enumerate(importance):
print('Feature: %0d, Score: %.5f' % (i,v))
```
完整代码如下:
```python
import pandas as pd
from sklearn.model_selection import train_test_split
from sklearn.ensemble import RandomForestClassifier
# 读取数据
df = pd.read_csv('data.csv')
# 分割训练集和测试集
X = df.drop(['label'], axis=1)
y = df['label']
X_train, X_test, y_train, y_test = train_test_split(X, y, test_size=0.2)
# 随机森林分类
clf = RandomForestClassifier(n_estimators=100, max_depth=5, random_state=42)
clf.fit(X_train, y_train)
# 分析特征重要性
importance = clf.feature_importances_
for i,v in enumerate(importance):
print('Feature: %0d, Score: %.5f' % (i,v))
```
阅读全文
相关推荐
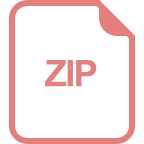
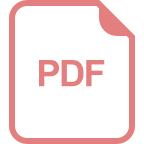
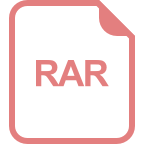
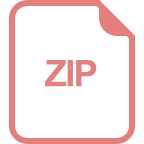
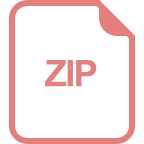
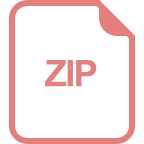
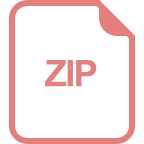
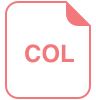
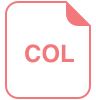
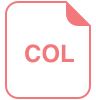
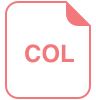
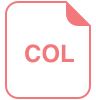
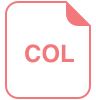
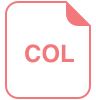
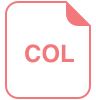
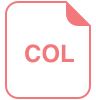
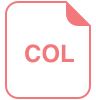
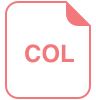
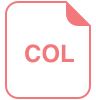