Feature Representation Learning for Unsupervised Cross-domain Image Retrieval
时间: 2024-05-22 18:11:18 浏览: 27
Feature representation learning for unsupervised cross-domain image retrieval refers to the process of extracting meaningful features from images in one domain (e.g., paintings) and using those features to retrieve similar images in another domain (e.g., photographs). This is typically done in an unsupervised manner, meaning that no explicit labels or annotations are provided to guide the learning process.
One common approach to unsupervised feature representation learning is to use deep neural networks, such as convolutional neural networks (CNNs), to extract high-level features from images. These features can then be used to train a retrieval model that maps images from one domain to the other based on their similarity in feature space.
There are several challenges to unsupervised cross-domain image retrieval, including differences in image content, style, and quality between domains. To address these challenges, researchers have developed a variety of techniques, such as domain adaptation, data augmentation, and adversarial training, to improve the performance of feature representation learning for cross-domain image retrieval.
Overall, feature representation learning for unsupervised cross-domain image retrieval is an important area of research with many potential applications, including art history, fashion, and product recommendation.
相关推荐
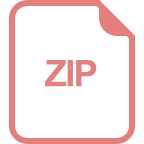
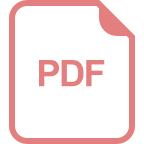
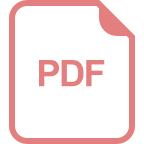














