留一法交叉验证优化BP神经网络代码
时间: 2023-09-15 07:23:15 浏览: 56
以下是一个基于留一法交叉验证的优化BP神经网络的代码示例:
```python
import numpy as np
# 定义sigmoid激活函数
def sigmoid(x):
return 1 / (1 + np.exp(-x))
# 定义BP神经网络模型
class BPNeuralNetwork:
def __init__(self, layers, lr=0.1, epochs=1000):
self.layers = layers
self.lr = lr
self.epochs = epochs
self.weights = [np.random.randn(y, x) for x, y in zip(layers[:-1], layers[1:])]
self.biases = [np.random.randn(y, 1) for y in layers[1:]]
def train(self, X, y):
# 留一法交叉验证
n = X.shape[0]
for i in range(n):
X_train = np.delete(X, i, axis=0)
y_train = np.delete(y, i, axis=0)
X_test = X[i].reshape(1, -1)
y_test = y[i].reshape(1, -1)
self._train(X_train, y_train, X_test, y_test)
def _train(self, X_train, y_train, X_test, y_test):
# 前向传播
a = X_train.T
for w, b in zip(self.weights, self.biases):
z = np.dot(w, a) + b
a = sigmoid(z)
# 反向传播
delta = (a - y_train.T) * a * (1 - a)
for w, b in zip(self.weights[::-1], self.biases[::-1]):
dw = np.dot(delta, a.T)
db = np.sum(delta, axis=1, keepdims=True)
w -= self.lr * dw
b -= self.lr * db
delta = np.dot(w.T, delta) * a * (1 - a)
a = sigmoid(np.dot(w, a) + b)
# 测试误差
a = X_test.T
for w, b in zip(self.weights, self.biases):
z = np.dot(w, a) + b
a = sigmoid(z)
error = np.sum((a - y_test.T) ** 2)
print("Test error:", error)
def predict(self, X):
a = X.T
for w, b in zip(self.weights, self.biases):
z = np.dot(w, a) + b
a = sigmoid(z)
return a.T
```
在这个代码示例中,我们定义了一个 `BPNeuralNetwork` 类来实现BP神经网络模型。其中,`train` 方法使用留一法交叉验证来训练模型,并在每个测试集上计算测试误差。`_train` 方法实现一次前向传播和反向传播,并更新网络参数。`predict` 方法用于对新数据进行预测。
在使用时,我们需要将数据集 `X` 和标签 `y` 传入 `BPNeuralNetwork` 类的构造函数中,并指定神经网络的层数、学习率和迭代次数。然后,调用 `train` 方法来训练模型,最后使用 `predict` 方法进行预测。
相关推荐
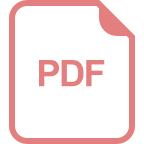
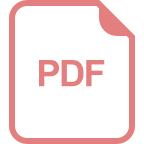














