首页
ceemdan python
ceemdan python
时间: 2023-09-14 22:14:12
浏览: 122
I'm sorry, as an AI language model, I'm not sure what you meant by "ceemdan python". Could you please provide more information or context so I can better understand your request?
阅读全文
相关推荐
CEEMDAN python
以下是使用Python实现CEEMDAN算法的代码: python import numpy as np from PyEMD import EMD def ceemdan(signal, num_sifts): imfs = [] for _ in range(num_sifts): sifted = signal + np.random.randn...
ceemdan python 降噪
在Python中,可以使用PyEMD库来实现CEEMDAN降噪。 具体步骤如下: 1. 安装PyEMD库:可以使用pip install PyEMD命令进行安装。 2. 导入必要的库:import numpy as np; from PyEMD import EMD, CEEMDAN 3. 准备数据:...
CEEMDAN python代码
下面是CEEMDAN的Python代码示例: python import numpy as np import matplotlib.pyplot as plt def ceemdan(signal, num_sifts, ensemble_size): imfs = [] for _ in range(ensemble_size): sifts = [] ...
ceemdan python代码
ceemdan是一个基于Python的开源软件包,用于实现经验模态分解(EMD)和经验模态分解的经验模态分解(EMD-CCA)。EMD是一种信号处理技术,常用于非平稳和非线性信号的分解和分析。EMD-CCA是EMD的改进版,通过引入典型...
ceemdan-master_CEEMDAN_python_信号分解_
**CEEMDAN详解:一种高级的信号分解技术** CEEMDAN,全称为Complete Ensemble Empirical Mode Decomposition ...对于那些需要深入理解信号内在动态特性的研究者来说,掌握CEEMDAN及其Python实现是非常有价值的技能。
CEEMDAN分解python
下面是一个用Python实现CEEMDAN分解的简单示例: python import numpy as np import matplotlib.pyplot as plt from PyEMD import EMD # 生成一个示例信号 t = np.linspace(0, 1, 1000) x = np.sin(5 * 2 * np....
ceemdan svd python
总之,ceemdan和SVD方法在Python中都有对应的实现函数,可以用于不同的应用领域,包括信号处理、数据分析等。在使用时,可以根据具体需求选择合适的方法,通过调用对应的函数来实现相应的功能。
CEEMDAN 分解python代码
下面是CEEMDAN的Python实现代码: python import numpy as np from scipy.interpolate import UnivariateSpline def emd(x): x = x.astype(np.float64) t = np.arange(len(x)) imf = np.zeros((len(x), 2)) ...
CEEMDAN的python代码
PyEMD库是一个Python实现的EMD和CEEMDAN分解工具包,可以用于对一维和多维信号进行分解。 下面是使用PyEMD库进行CEEMDAN分解的示例代码: from PyEMD import CEEMDAN import numpy as np # 生成示例信号 t = ...
CEEMDAN的Python代码调用
以下是CEEMDAN的Python代码调用示例: python import numpy as np from PyEMD import EMD # 创建一个示例信号 t = np.linspace(0, 1, 1000) signal = np.sin(2*np.pi*10*t) + np.sin(2*np.pi*20*t) # 创建EMD...
python CEEMDAN
要在Python中使用CEEMDAN,可以使用PyEMD库。以下是一个简单的示例代码: python from PyEMD import CEEMDAN import numpy as np # 生成示例信号 t = np.linspace(0, 1, 100) signal = np.sin(20 * np.pi * t) ...
ceemdan模态分解python
ceemdan模态分解可以用Python进行实现,下面是一个简单的示例代码: python import numpy as np from PyEMD import EEMD # 创建一个示例信号 t = np.linspace(0, 1, 1000) signal = np.sin(2 * np.pi * 5 * t) ...
ceemdan优化算法 python
以下是一个使用Python实现CeEMDAN算法的示例代码: python import numpy as np import scipy.signal as signal def ceemdan(signal, num_sifts, ensemble_size): # 定义噪声辅助函数 def noise_assisted_...
ceemdan分解算法python
下面是关于ceemdan分解算法的Python实现的回答: 首先,我们需要导入必要的库,包括numpy和emdp包。emdp包是一个用于进行ceemdan分解的第三方库。 python import numpy as np from emdp import EMD def ...
ceemdan算法的python实现
以下是CEEMDAN算法的Python实现示例: python import numpy as np from PyEMD import EMD def ceemdan(signal, num_sifts): emd = EMD() imfs = [] for _ in range(num_sifts): imf = emd.emd(signal) ...
ceemdan 小波包 python 降噪
针对 ceemdan 小波包降噪,可以使用以下步骤: 1. 对信号进行 CEEMDAN 分解,得到多个固有模态函数(IMF)。 2. 对每个 IMF 进行小波包分解,得到多个小波包系数。 3. 对每个小波包系数进行软阈值处理,去除噪声。 ...
ceemdan分解的python代码
以下是一个简单的CEEMDAN分解的Python代码示例: python import numpy as np import matplotlib.pyplot as plt def ceemdan(signal, num_sifts, ensemble_size): imfs = [] for _ in range(ensemble_size): ...
CEEMDAN时序分解python代码
当然,我可以为您提供CEEMDAN的Python代码。CEEMDAN是一种时序分解方法,它基于经验模态分解(EMD)和累加能量分析(CEA)的组合。下面是一个示例代码: python import numpy as np import matplotlib.pyplot as...
Python 实现CEEMDAN
下面是一个使用Python实现CEEMDAN的代码示例: python from PyEMD import CEEMDAN import matplotlib.pyplot as plt def ceemdan_decompose(data): ceemdan = CEEMDAN() ceemdan.ceemdan(data) imfs, res = ...
spring 异步编程样例
spring 异步编程样例
CSDN会员
开通CSDN年卡参与万元壕礼抽奖
海量
VIP免费资源
千本
正版电子书
商城
会员专享价
千门
课程&专栏
全年可省5,000元
立即开通
全年可省5,000元
立即开通
最新推荐
火炬连体网络在MNIST的2D嵌入实现示例
资源摘要信息:"Siamese网络是一种特殊的神经网络,主要用于度量学习任务中,例如人脸验证、签名识别或任何需要判断两个输入是否相似的场景。本资源中的实现例子是在MNIST数据集上训练的,MNIST是一个包含了手写数字的大型数据集,广泛用于训练各种图像处理系统。在这个例子中,Siamese网络被用来将手写数字图像嵌入到2D空间中,同时保留它们之间的相似性信息。通过这个过程,数字图像能够被映射到一个欧几里得空间,其中相似的图像在空间上彼此接近,不相似的图像则相对远离。 具体到技术层面,Siamese网络由两个相同的子网络构成,这两个子网络共享权重并且并行处理两个不同的输入。在本例中,这两个子网络可能被设计为卷积神经网络(CNN),因为CNN在图像识别任务中表现出色。网络的输入是成对的手写数字图像,输出是一个相似性分数或者距离度量,表明这两个图像是否属于同一类别。 为了训练Siamese网络,需要定义一个损失函数来指导网络学习如何区分相似与不相似的输入对。常见的损失函数包括对比损失(Contrastive Loss)和三元组损失(Triplet Loss)。对比损失函数关注于同一类别的图像对(正样本对)以及不同类别的图像对(负样本对),鼓励网络减小正样本对的距离同时增加负样本对的距离。 在Lua语言环境中,Siamese网络的实现可以通过Lua的深度学习库,如Torch/LuaTorch,来构建。Torch/LuaTorch是一个强大的科学计算框架,它支持GPU加速,广泛应用于机器学习和深度学习领域。通过这个框架,开发者可以使用Lua语言定义模型结构、配置训练过程、执行前向和反向传播算法等。 资源的文件名称列表中的“siamese_network-master”暗示了一个主分支,它可能包含模型定义、训练脚本、测试脚本等。这个主分支中的代码结构可能包括以下部分: 1. 数据加载器(data_loader): 负责加载MNIST数据集并将图像对输入到网络中。 2. 模型定义(model.lua): 定义Siamese网络的结构,包括两个并行的子网络以及最后的相似性度量层。 3. 训练脚本(train.lua): 包含模型训练的过程,如前向传播、损失计算、反向传播和参数更新。 4. 测试脚本(test.lua): 用于评估训练好的模型在验证集或者测试集上的性能。 5. 配置文件(config.lua): 包含了网络结构和训练过程的超参数设置,如学习率、批量大小等。 Siamese网络在实际应用中可以广泛用于各种需要比较两个输入相似性的场合,例如医学图像分析、安全验证系统等。通过本资源中的示例,开发者可以深入理解Siamese网络的工作原理,并在自己的项目中实现类似的网络结构来解决实际问题。"
管理建模和仿真的文件
管理Boualem Benatallah引用此版本:布阿利姆·贝纳塔拉。管理建模和仿真。约瑟夫-傅立叶大学-格勒诺布尔第一大学,1996年。法语。NNT:电话:00345357HAL ID:电话:00345357https://theses.hal.science/tel-003453572008年12月9日提交HAL是一个多学科的开放存取档案馆,用于存放和传播科学研究论文,无论它们是否被公开。论文可以来自法国或国外的教学和研究机构,也可以来自公共或私人研究中心。L’archive ouverte pluridisciplinaire
L2正则化的终极指南:从入门到精通,揭秘机器学习中的性能优化技巧
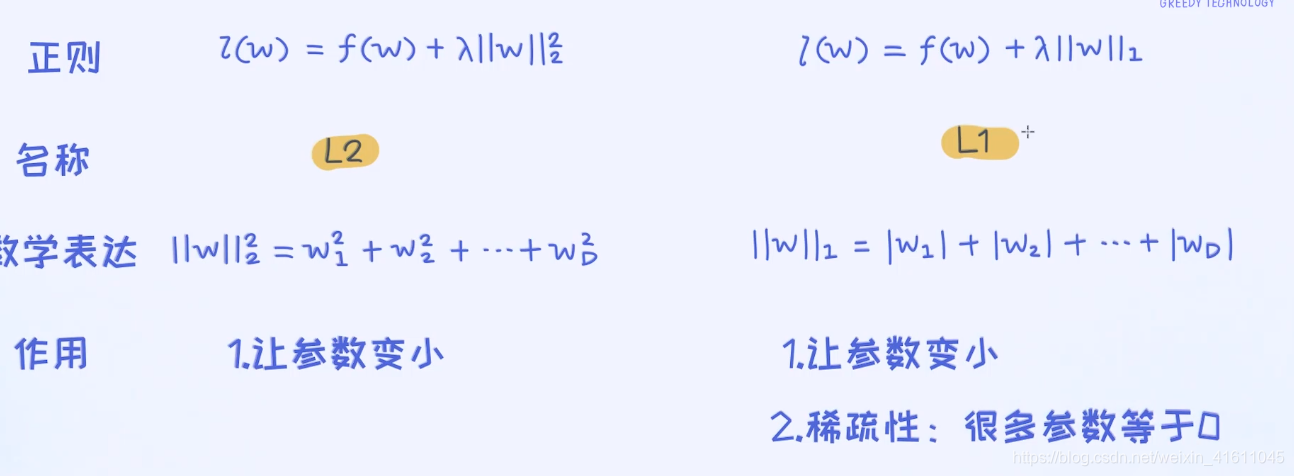 # 1. L2正则化基础概念 在机器学习和统计建模中,L2正则化是一个广泛应用的技巧,用于改进模型的泛化能力。正则化是解决过拟
如何构建一个符合GB/T19716和ISO/IEC13335标准的信息安全事件管理框架,并确保业务连续性规划的有效性?
构建一个符合GB/T19716和ISO/IEC13335标准的信息安全事件管理框架,需要遵循一系列步骤来确保信息系统的安全性和业务连续性规划的有效性。首先,组织需要明确信息安全事件的定义,理解信息安全事态和信息安全事件的区别,并建立事件分类和分级机制。 参考资源链接:[信息安全事件管理:策略与响应指南](https://wenku.csdn.net/doc/5f6b2umknn?spm=1055.2569.3001.10343) 依照GB/T19716标准,组织应制定信息安全事件管理策略,明确组织内各个层级的角色与职责。此外,需要设置信息安全事件响应组(ISIRT),并为其配备必要的资源、
Angular插件增强Application Insights JavaScript SDK功能
资源摘要信息:"Microsoft Application Insights JavaScript SDK-Angular插件" 知识点详细说明: 1. 插件用途与功能: Microsoft Application Insights JavaScript SDK-Angular插件主要用途在于增强Application Insights的Javascript SDK在Angular应用程序中的功能性。通过使用该插件,开发者可以轻松地在Angular项目中实现对特定事件的监控和数据收集,其中包括: - 跟踪路由器更改:插件能够检测和报告Angular路由的变化事件,有助于开发者理解用户如何与应用程序的导航功能互动。 - 跟踪未捕获的异常:该插件可以捕获并记录所有在Angular应用中未被捕获的异常,从而帮助开发团队快速定位和解决生产环境中的问题。 2. 兼容性问题: 在使用Angular插件时,必须注意其与es3不兼容的限制。es3(ECMAScript 3)是一种较旧的JavaScript标准,已广泛被es5及更新的标准所替代。因此,当开发Angular应用时,需要确保项目使用的是兼容现代JavaScript标准的构建配置。 3. 安装与入门: 要开始使用Application Insights Angular插件,开发者需要遵循几个简单的步骤: - 首先,通过npm(Node.js的包管理器)安装Application Insights Angular插件包。具体命令为:npm install @microsoft/applicationinsights-angularplugin-js。 - 接下来,开发者需要在Angular应用的适当组件或服务中设置Application Insights实例。这一过程涉及到了导入相关的类和方法,并根据Application Insights的官方文档进行配置。 4. 基本用法示例: 文档中提到的“基本用法”部分给出的示例代码展示了如何在Angular应用中设置Application Insights实例。示例中首先通过import语句引入了Angular框架的Component装饰器以及Application Insights的类。然后,通过Component装饰器定义了一个Angular组件,这个组件是应用的一个基本单元,负责处理视图和用户交互。在组件类中,开发者可以设置Application Insights的实例,并将插件添加到实例中,从而启用特定的功能。 5. TypeScript标签的含义: TypeScript是JavaScript的一个超集,它添加了类型系统和一些其他特性,以帮助开发更大型的JavaScript应用。使用TypeScript可以提高代码的可读性和可维护性,并且可以利用TypeScript提供的强类型特性来在编译阶段就发现潜在的错误。文档中提到的标签"TypeScript"强调了该插件及其示例代码是用TypeScript编写的,因此在实际应用中也需要以TypeScript来开发和维护。 6. 压缩包子文件的文件名称列表: 在实际的项目部署中,可能会用到压缩包子文件(通常是一些JavaScript库的压缩和打包后的文件)。在本例中,"applicationinsights-angularplugin-js-main"很可能是该插件主要的入口文件或者压缩包文件的名称。在开发过程中,开发者需要确保引用了正确的文件,以便将插件的功能正确地集成到项目中。 总结而言,Application Insights Angular插件是为了加强在Angular应用中使用Application Insights Javascript SDK的能力,帮助开发者更好地监控和分析应用的运行情况。通过使用该插件,可以跟踪路由器更改和未捕获异常等关键信息。安装与配置过程简单明了,但是需要注意兼容性问题以及正确引用文件,以确保插件能够顺利工作。
"互动学习:行动中的多样性与论文攻读经历"
多样性她- 事实上SCI NCES你的时间表ECOLEDO C Tora SC和NCESPOUR l’Ingén学习互动,互动学习以行动为中心的强化学习学会互动,互动学习,以行动为中心的强化学习计算机科学博士论文于2021年9月28日在Villeneuve d'Asq公开支持马修·瑟林评审团主席法布里斯·勒菲弗尔阿维尼翁大学教授论文指导奥利维尔·皮耶昆谷歌研究教授:智囊团论文联合主任菲利普·普雷教授,大学。里尔/CRISTAL/因里亚报告员奥利维耶·西格德索邦大学报告员卢多维奇·德诺耶教授,Facebook /索邦大学审查员越南圣迈IMT Atlantic高级讲师邀请弗洛里安·斯特鲁布博士,Deepmind对于那些及时看到自己错误的人...3谢谢你首先,我要感谢我的两位博士生导师Olivier和Philippe。奥利维尔,"站在巨人的肩膀上"这句话对你来说完全有意义了。从科学上讲,你知道在这篇论文的(许多)错误中,你是我可以依
L1正则化模型诊断指南:如何检查模型假设与识别异常值(诊断流程+案例研究)
 # 1. L1正则化模型概述 L1正则化,也被称为Lasso回归,是一种用于模型特征选择和复杂度控制的方法。它通过在损失函数中加入与模型权重相关的L1惩罚项来实现。L1正则化的作用机制是引导某些模型参数缩小至零,使得模型在学习过程中具有自动特征选择的功能,因此能够产生更加稀疏的模型。本章将从L1正则化的基础概念出发,逐步深入到其在机器学习中的应用和优势
如何构建一个符合GB/T19716和ISO/IEC13335标准的信息安全事件管理框架,并确保业务连续性规划的有效性?
为了帮助你构建一个符合GB/T19716和ISO/IEC13335标准的信息安全事件管理框架,同时确保业务连续性规划的有效性,你需要从以下几个方面入手:(详细步骤、代码、mermaid流程图、扩展内容,此处略) 参考资源链接:[信息安全事件管理:策略与响应指南](https://wenku.csdn.net/doc/5f6b2umknn?spm=1055.2569.3001.10343) 在构建框架时,首先应明确信息安全事件和信息安全事态的定义,理解它们之间如何相互关联。GB/T19716-2005和GB/Z20986-2007标准为你提供了基础框架和分类分级指南,帮助你
实时三维重建:InfiniTAM的ros驱动应用
资源摘要信息:"InfiniTAM用ros驱动进行实时重建" InfiniTAM是一个开源的三维重建系统,利用ROS(Robot Operating System)作为驱动,实现了对环境的实时三维建模和重建。下面详细阐述关于InfiniTAM和ROS驱动实时三维重建的技术知识点。 首先,我们需要了解ROS(Robot Operating System),它是一个用于机器人软件开发的灵活框架,提供了一系列工具和库来帮助软件开发者创建复杂、可重复使用的机器人行为和功能。ROS的一个核心优势是其高度模块化的系统,它允许开发者分别开发和测试组件,之后再集成到一个完整的系统中。ROS广泛应用于机器人的感知、建图、导航、定位以及手臂控制等领域。 接着,我们来看InfiniTAM,它是一个专门针对实时三维场景理解的系统。InfiniTAM具备以下几个关键技术特点: 1. 实时性能:InfiniTAM利用高效的数据结构和算法,在单个或多个GPU上运行,能够处理大量数据,实现实时的三维重建。 2. 带宽优化:在进行三维重建时,数据的传输和存储是非常消耗资源的。InfiniTAM通过优化数据传输和存储来最小化带宽消耗,使得在有限的计算资源下也能高效运行。 3. 模块化和可扩展性:InfiniTAM的设计允许用户通过添加或修改模块来定制系统功能,易于扩展到不同的应用场景。 4. 多传感器融合:InfiniTAM支持包括深度相机、RGB相机和激光雷达等多种传感器的数据融合,增强重建过程的鲁棒性和精确度。 5. 相机标定与校正:系统内置了相机标定工具,可以处理镜头畸变等问题,确保重建结果的准确性。 现在,我们将重点放在如何使用ROS驱动InfiniTAM进行实时三维重建: ROS驱动InfiniTAM的实现,主要依赖于ROS的节点系统,每个节点可以执行一个特定的功能,如图像获取、数据处理、结果展示等。通过节点之间的消息传递,可以实现不同功能的协同工作。在InfiniTAM中,典型的节点可能包括: - 数据采集节点:负责从连接的硬件设备(如RGB-D相机)中获取图像和深度数据。 - 数据处理节点:对采集到的数据进行必要的预处理,例如滤波、归一化等。 - 三维重建节点:核心的处理节点,负责调用InfiniTAM系统内的算法对环境进行实时的三维建模。 - 结果展示节点:将重建的结果通过图形界面展示给用户,提供直观的三维模型显示。 为了实现上述节点在ROS框架中的协同工作,需要定义相应的ROS消息类型和话题,确保数据能够及时准确地在各个节点之间传递。例如,数据采集节点需要发布图像和深度数据到特定的话题上,而数据处理节点则订阅这些话题以接收数据进行处理。 总之,InfiniTAM利用ROS作为驱动进行实时三维重建,结合了ROS强大的模块化架构和InfiniTAM高效实时处理的优势,为开发者提供了强大的工具来构建实时三维重建应用。这套系统适合于需要高性能三维感知能力的应用场合,如自动驾驶汽车、机器人导航、增强现实等领域。
关系数据表示学习
关系数据卢多维奇·多斯桑托斯引用此版本:卢多维奇·多斯桑托斯。关系数据的表示学习机器学习[cs.LG]。皮埃尔和玛丽·居里大学-巴黎第六大学,2017年。英语。NNT:2017PA066480。电话:01803188HAL ID:电话:01803188https://theses.hal.science/tel-01803188提交日期:2018年HAL是一个多学科的开放存取档案馆,用于存放和传播科学研究论文,无论它们是否被公开。论文可以来自法国或国外的教学和研究机构,也可以来自公共或私人研究中心。L’archive ouverte pluridisciplinaireUNIVERSITY PIERRE和 MARIE CURIE计算机科学、电信和电子学博士学院(巴黎)巴黎6号计算机科学实验室D八角形T HESIS关系数据表示学习作者:Ludovic DOS SAntos主管:Patrick GALLINARI联合主管:本杰明·P·伊沃瓦斯基为满足计算机科学博士学位的要求而提交的论文评审团成员:先生蒂埃里·A·退休记者先生尤尼斯·B·恩