sentences=[' '.join(df['clean_review'])]解释代码意思
时间: 2023-08-25 07:04:34 浏览: 34
当我们处理文本数据时,通常需要将多条文本数据合并成一个大的字符串,以便进行后续的文本处理,比如进行词频统计、训练词向量等。这段代码的作用就是将DataFrame中名为'clean_review'的一列文本数据进行拼接,最终得到一个包含所有文本的字符串,存储在sentences列表中。
具体地,代码中使用了join()方法,将Series对象 df['clean_review'] 中的每个元素用空格拼接成一个字符串。然后,将得到的字符串存储在一个列表中。需要注意的是,这个列表中只有一个元素,即所有文本数据拼接后的字符串。
相关问题
model = Word2Vec(sentences=df.clean_review, size=100, window=5, min_count=1, workers=4)解释代码意思
这段代码使用了gensim库中的Word2Vec模型,目的是将语料库`df.clean_review`中的单词转换为向量表示,便于后续的文本处理和分析。
具体来说,这段代码的参数如下:
- `sentences`: 指定Word2Vec模型要处理的语料库,这里是一个pandas DataFrame中的一列`clean_review`,它包含了已经被处理过(如去掉了停用词、标点符号、数字等)的文本数据;
- `size`: 指定词向量的维度,这里是100,即每个单词将被转换为一个100维的向量;
- `window`: 指定Word2Vec模型中窗口大小,即模型会考虑目标单词周围的几个单词,这里是5,表示模型将考虑目标单词前后各5个单词;
- `min_count`: 指定单词在语料库中出现的最小次数,出现次数少于这个值的单词将被忽略,这里是1,表示出现过一次的单词都会被考虑;
- `workers`: 指定训练模型时使用的线程数,这里是4,表示使用4个线程来训练模型。
这段代码的功能是训练Word2Vec模型,并将其保存在一个变量`model`中,该模型可以将一段文本中的单词转换为对应的向量表示。
import tensorflow as tf import tensorflow_hub as hub from tensorflow.keras import layers import bert import numpy as np from transformers import BertTokenizer, BertModel # 设置BERT模型的路径和参数 bert_path = "E:\\AAA\\523\\BERT-pytorch-master\\bert1.ckpt" max_seq_length = 128 train_batch_size = 32 learning_rate = 2e-5 num_train_epochs = 3 # 加载BERT模型 def create_model(): input_word_ids = tf.keras.layers.Input(shape=(max_seq_length,), dtype=tf.int32, name="input_word_ids") input_mask = tf.keras.layers.Input(shape=(max_seq_length,), dtype=tf.int32, name="input_mask") segment_ids = tf.keras.layers.Input(shape=(max_seq_length,), dtype=tf.int32, name="segment_ids") bert_layer = hub.KerasLayer(bert_path, trainable=True) pooled_output, sequence_output = bert_layer([input_word_ids, input_mask, segment_ids]) output = layers.Dense(1, activation='sigmoid')(pooled_output) model = tf.keras.models.Model(inputs=[input_word_ids, input_mask, segment_ids], outputs=output) return model # 准备数据 def create_input_data(sentences, labels): tokenizer = bert.tokenization.FullTokenizer(vocab_file=bert_path + "trainer/vocab.small", do_lower_case=True) # tokenizer = BertTokenizer.from_pretrained('bert-base-uncased') input_ids = [] input_masks = [] segment_ids = [] for sentence in sentences: tokens = tokenizer.tokenize(sentence) tokens = ["[CLS]"] + tokens + ["[SEP]"] input_id = tokenizer.convert_tokens_to_ids(tokens) input_mask = [1] * len(input_id) segment_id = [0] * len(input_id) padding_length = max_seq_length - len(input_id) input_id += [0] * padding_length input_mask += [0] * padding_length segment_id += [0] * padding_length input_ids.append(input_id) input_masks.append(input_mask) segment_ids.append(segment_id) return np.array(input_ids), np.array(input_masks), np.array(segment_ids), np.array(labels) # 加载训练数据 train_sentences = ["Example sentence 1", "Example sentence 2", ...] train_labels = [0, 1, ...] train_input_ids, train_input_masks, train_segment_ids, train_labels = create_input_data(train_sentences, train_labels) # 构建模型 model = create_model() model.compile(optimizer=tf.keras.optimizers.Adam(lr=learning_rate), loss='binary_crossentropy', metrics=['accuracy']) # 开始微调 model.fit([train_input_ids, train_input_masks, train_segment_ids], train_labels, batch_size=train_batch_size, epochs=num_train_epochs)这段代码有什么问题吗?
这段代码存在一些问题:
1. `bert_path` 的值不应该是 ".ckpt" 结尾的文件路径,而是BERT模型的目录路径,例如:`bert_path = "E:\\AAA\\523\\BERT-pytorch-master\\"`。
2. `vocab_file` 参数应该是BERT模型目录下的 "vocab.txt" 文件路径,而不是训练器目录下的 "vocab.small" 文件路径。例如:`tokenizer = bert.tokenization.FullTokenizer(vocab_file=bert_path + "vocab.txt", do_lower_case=True)`。
3. `create_input_data` 函数中的 `tokenizer` 对象应该是从 `BertTokenizer` 类中导入的。因此,需要将 `from transformers import BertTokenizer` 加入代码开头,并使用 `BertTokenizer.from_pretrained()` 函数来加载BERT的tokenizer。
4. `train_sentences` 和 `train_labels` 应该是训练数据集,但是在代码中缺失了这些数据的定义和加载。
5. `create_input_data` 函数返回的 `labels` 应该是一维的,而不是二维的。因此,需要修改函数 `return` 语句为:`return np.array(input_ids), np.array(input_masks), np.array(segment_ids), np.array(labels).reshape(-1)`。
6. 在 `model.fit` 中,需要指定验证集的参数 `validation_data`,以便在训练过程中评估模型的性能。
综上所述,以下是修改后的代码示例:
```
import tensorflow as tf
import tensorflow_hub as hub
from tensorflow.keras import layers
import bert
import numpy as np
from transformers import BertTokenizer, BertModel
# 设置BERT模型的路径和参数
bert_path = "E:\\AAA\\523\\BERT-pytorch-master\\"
max_seq_length = 128
train_batch_size = 32
learning_rate = 2e-5
num_train_epochs = 3
# 加载BERT模型
def create_model():
input_word_ids = tf.keras.layers.Input(shape=(max_seq_length,), dtype=tf.int32, name="input_word_ids")
input_mask = tf.keras.layers.Input(shape=(max_seq_length,), dtype=tf.int32, name="input_mask")
segment_ids = tf.keras.layers.Input(shape=(max_seq_length,), dtype=tf.int32, name="segment_ids")
bert_layer = hub.KerasLayer(bert_path, trainable=True)
pooled_output, sequence_output = bert_layer([input_word_ids, input_mask, segment_ids])
output = layers.Dense(1, activation='sigmoid')(pooled_output)
model = tf.keras.models.Model(inputs=[input_word_ids, input_mask, segment_ids], outputs=output)
return model
# 准备数据
def create_input_data(sentences, labels):
tokenizer = BertTokenizer.from_pretrained('bert-base-uncased')
input_ids = []
input_masks = []
segment_ids = []
for sentence in sentences:
tokens = tokenizer.tokenize(sentence)
tokens = ["[CLS]"] + tokens + ["[SEP]"]
input_id = tokenizer.convert_tokens_to_ids(tokens)
input_mask = [1] * len(input_id)
segment_id = [0] * len(input_id)
padding_length = max_seq_length - len(input_id)
input_id += [0] * padding_length
input_mask += [0] * padding_length
segment_id += [0] * padding_length
input_ids.append(input_id)
input_masks.append(input_mask)
segment_ids.append(segment_id)
return np.array(input_ids), np.array(input_masks), np.array(segment_ids), np.array(labels).reshape(-1)
# 加载训练数据
train_sentences = ["Example sentence 1", "Example sentence 2", ...]
train_labels = [0, 1, ...]
train_input_ids, train_input_masks, train_segment_ids, train_labels = create_input_data(train_sentences, train_labels)
# 构建模型
model = create_model()
model.compile(optimizer=tf.keras.optimizers.Adam(lr=learning_rate),
loss='binary_crossentropy',
metrics=['accuracy'])
# 开始微调
model.fit([train_input_ids, train_input_masks, train_segment_ids], train_labels,
batch_size=train_batch_size,
epochs=num_train_epochs,
validation_data=([val_input_ids, val_input_masks, val_segment_ids], val_labels))
```
相关推荐
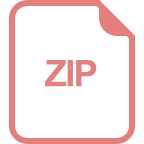
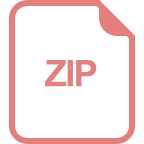













