详细分析代码“from sklearn.cross_validation import StratifiedKFold from sklearn.naive_bayes import MultinomialNB from sklearn.metrics import accuracy_score,precision_score #from sklearn.model_selection import train_test_split x,y=zip(*sentences) from sklearn.feature_extraction.text import CountVectorizer vec = CountVectorizer( analyzer='word', # tokenise by character ngrams ngram_range=(1,4), # use ngrams of size 1 and 2 max_features=20000, # keep the most common 1000 ngrams ) vec.fit(x) def stratifiedkfold_cv(x,y,clf_class,shuffle=True,n_folds=5,**kwargs): stratifiedk_fold = StratifiedKFold(y, n_folds=n_folds, shuffle=shuffle) y_pred = y[:] for train_index, test_index in stratifiedk_fold: X_train, X_test = x[train_index], x[test_index] y_train = y[train_index] clf = clf_class(**kwargs) clf.fit(X_train,y_train) y_pred[test_index] = clf.predict(X_test) return y_pred NB = MultinomialNB print(precision_score(y ,stratifiedkfold_cv(vec.transform(x) ,np.array(y),NB) , average='macro'))”并添加注释,每段代码的作用,参数代表什么
时间: 2023-12-04 09:03:15 浏览: 47
```
# 导入StratifiedKFold交叉验证、MultinomialNB朴素贝叶斯分类器和评估指标accuracy_score、precision_score
from sklearn.cross_validation import StratifiedKFold
from sklearn.naive_bayes import MultinomialNB
from sklearn.metrics import accuracy_score, precision_score
# 从sentences中分离出x和y
x, y = zip(*sentences)
# 导入CountVectorizer词袋模型,并对x进行处理
from sklearn.feature_extraction.text import CountVectorizer
vec = CountVectorizer(
analyzer='word', # 按照单词进行分割
ngram_range=(1,4), # 使用1~4个单词的组合方式
max_features=20000, # 保留最常见的20000个单词组合
)
vec.fit(x)
# 对数据进行StratifiedKFold交叉验证
def stratifiedkfold_cv(x,y,clf_class,shuffle=True,n_folds=5,**kwargs):
stratifiedk_fold = StratifiedKFold(y, n_folds=n_folds, shuffle=shuffle) # 将数据划分为5折,每一折中正负样本比例都相同
y_pred = y[:] # 复制y
for train_index, test_index in stratifiedk_fold: # 循环5次,每一次使用其中4折作为训练数据,1折作为测试数据
X_train, X_test = x[train_index], x[test_index]
y_train = y[train_index]
clf = clf_class(**kwargs) # 使用朴素贝叶斯分类器
clf.fit(X_train,y_train) # 训练模型
y_pred[test_index] = clf.predict(X_test) # 预测测试数据
return y_pred
NB = MultinomialNB # 定义朴素贝叶斯分类器
print(precision_score(y, # 计算精确率
stratifiedkfold_cv(vec.transform(x), # 对数据进行词袋模型转换
np.array(y), # 将y转换为数组形式
NB), # 使用朴素贝叶斯分类器进行分类
average='macro')) # 按照宏平均计算
```
参数说明:
- x: 输入的文本数据,类型为tuple或list
- y: 对应的标签,类型为tuple或list
- analyzer: 按照什么方式进行分割,默认为'word',即按照单词进行分割
- ngram_range: 使用几个单词的组合,默认为(1,1),即只使用单个单词
- max_features: 保留最常见的单词组合数量,默认为None,即保留所有单词组合
- shuffle: 是否对数据进行随机排序,默认为True
- n_folds: 将数据划分为几折,默认为5
- clf_class: 指定分类器的类别,默认为MultinomialNB
- kwargs: 指定分类器的其他参数
此段代码的作用是:对文本数据进行朴素贝叶斯分类器的精确率评估,并使用StratifiedKFold交叉验证对数据进行划分,保证每一折中正负样本的比例相同。首先将文本数据进行词袋模型转换,然后使用stratifiedkfold_cv函数对数据进行交叉验证和分类,最后计算并输出精确率值。
相关推荐
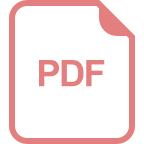














